A Channel-based Authentication Using Machine Learning for Body Sensor Networks.
GLOBECOM(2022)
摘要
In Body Sensor Networks (BSNs), connected nodes on the body exhibit different channel characteristics than any other remote node, such as an attacker who wishes to disrupt the network. Based on this observation, several channel-based Physical Layer Authentication (PLA) protocols have used Received Signal Strength Indicator (RSSI) for authentication. Supported by experiments performed with ESP32 WiFi modules, we introduce a robust feature set that consists of statistical characteristics of RSSI and Frame Loss Rate (FLR) to design a lightweight authentication scheme. To create a realistic dataset in a BSN (using the IEEE 802.15.6 standard), Castalia was used with a network configuration of 6 sensor nodes, a controller node, and an attacker node. To learn the channel behavior of sensor nodes, we adopt a machine learning approach. The model has been trained with a dataset that includes the RSSI and FLR features of 6 sensors and 1 attacker and is capable of classifying received packets by sender, which allows us to detect any attacker trying to send data to the network. The accuracy of the proposed scheme is 95%. Moreover, true attack detection and false attack detection rate is 88%, and 4.1% respectively.
更多查看译文
关键词
BSN,IEEE 802.15.6,Machine Learning,Authentication
AI 理解论文
溯源树
样例
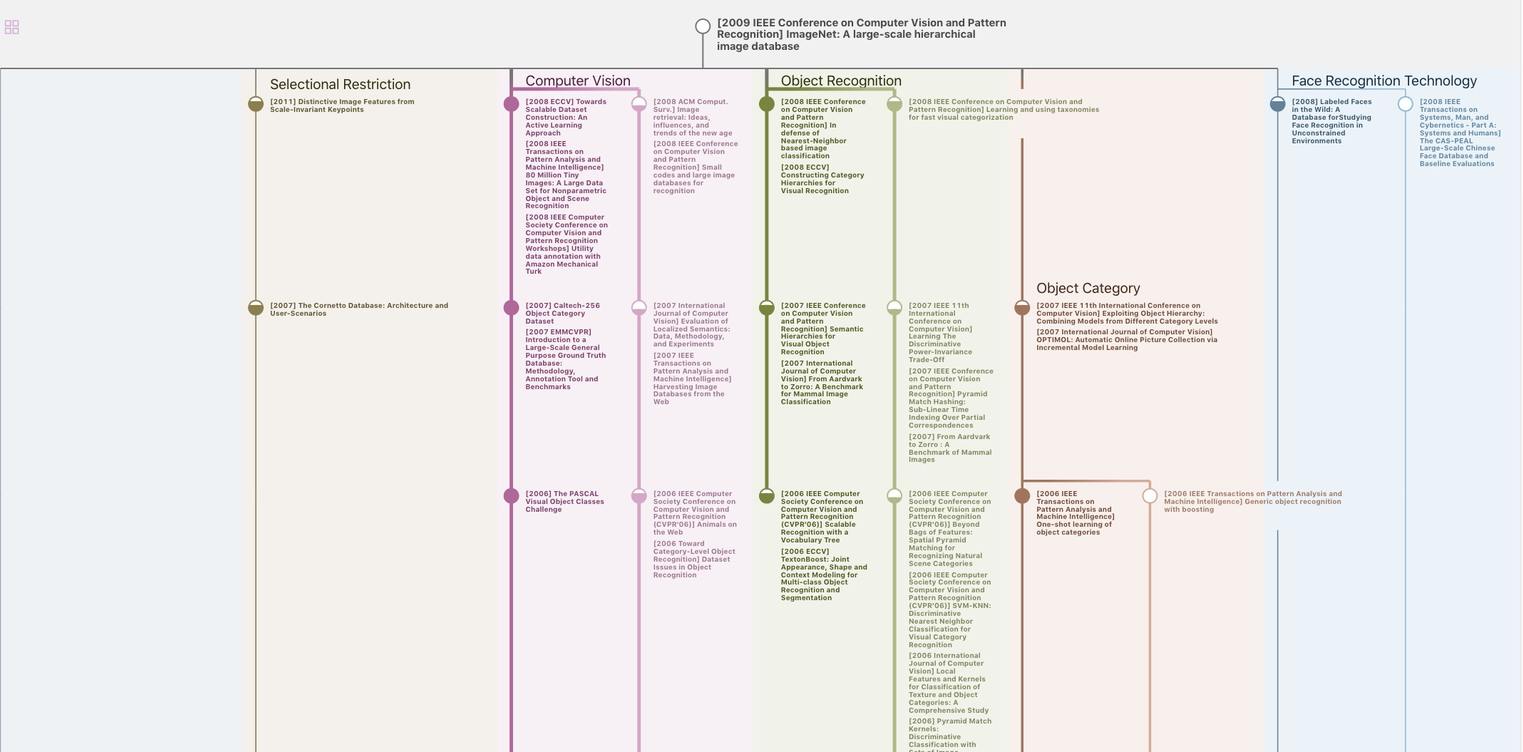
生成溯源树,研究论文发展脉络
Chat Paper
正在生成论文摘要