Privately Customizing Prefinetuning to Better Match User Data in Federated Learning
arxiv(2023)
摘要
In Federated Learning (FL), accessing private client data incurs communication and privacy costs. As a result, FL deployments commonly prefinetune pretrained foundation models on a (large, possibly public) dataset that is held by the central server; they then FL-finetune the model on a private, federated dataset held by clients. Evaluating prefinetuning dataset quality reliably and privately is therefore of high importance. To this end, we propose FreD (Federated Private Fr\'echet Distance) -- a privately computed distance between a prefinetuning dataset and federated datasets. Intuitively, it privately computes and compares a Fr\'echet distance between embeddings generated by a large language model on both the central (public) dataset and the federated private client data. To make this computation privacy-preserving, we use distributed, differentially-private mean and covariance estimators. We show empirically that FreD accurately predicts the best prefinetuning dataset at minimal privacy cost. Altogether, using FreD we demonstrate a proof-of-concept for a new approach in private FL training: (1) customize a prefinetuning dataset to better match user data (2) prefinetune (3) perform FL-finetuning.
更多查看译文
关键词
better match user data,customizing prefinetuning,learning
AI 理解论文
溯源树
样例
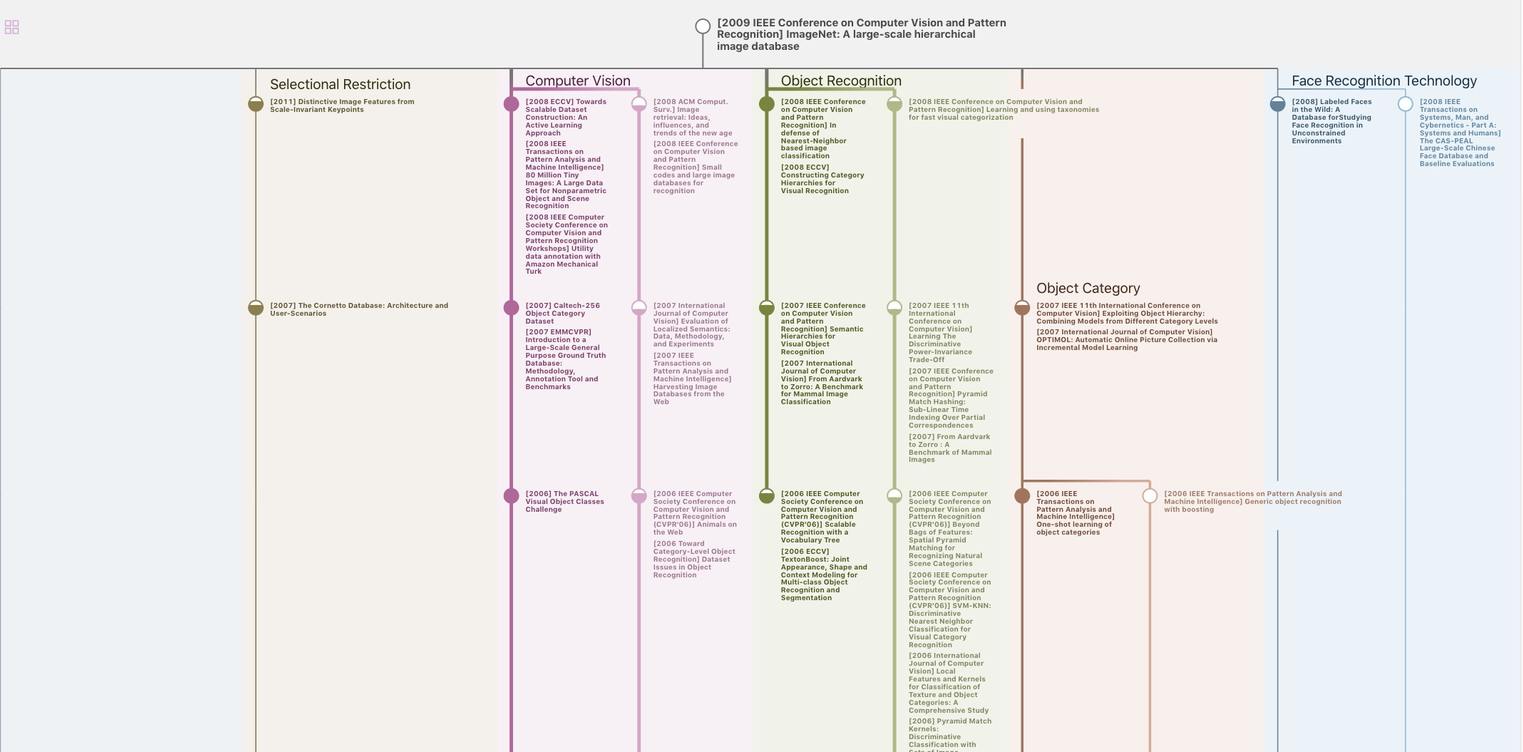
生成溯源树,研究论文发展脉络
Chat Paper
正在生成论文摘要