Exploring External Knowledge for Accurate modeling of Visual and Language Problems
arxiv(2023)
摘要
The interest in Artificial Intelligence (AI) and its applications has seen unprecedented growth in the last few years. The success can be partly attributed to the advancements of deep neural networks made in the sub-fields of AI such as Computer Vision (CV) and Natural Language Processing (NLP). The promising research area that this dissertation focuses on is visual and language understanding which involves many challenging tasks, i.e., classification, detection, segmentation, machine translation and captioning, etc. The state-of-the-art methods for solving these problems usually involves only two parts: source data and target labels, which is rather insufficient especially when the dataset is small. Meanwhile, many external tools or sources can provide extra useful information (external knowledge) that can help improve the performance of these methods. For example, a detection model has been applied to provide better object features than state-of-the-art ResNet for image captioning models. Inspired by this observation, we developed a methodology that we can first extract external knowledge and then integrate it with the original models. The external knowledge has to be extracted from the dataset, or can directly come from external, e.g., grammar rules or scene graphs. We apply this methodology to different AI tasks, including machine translation and image captioning and improve the original state-of-the-art models by a large margin.
更多查看译文
关键词
external knowledge,accurate modeling,language problems
AI 理解论文
溯源树
样例
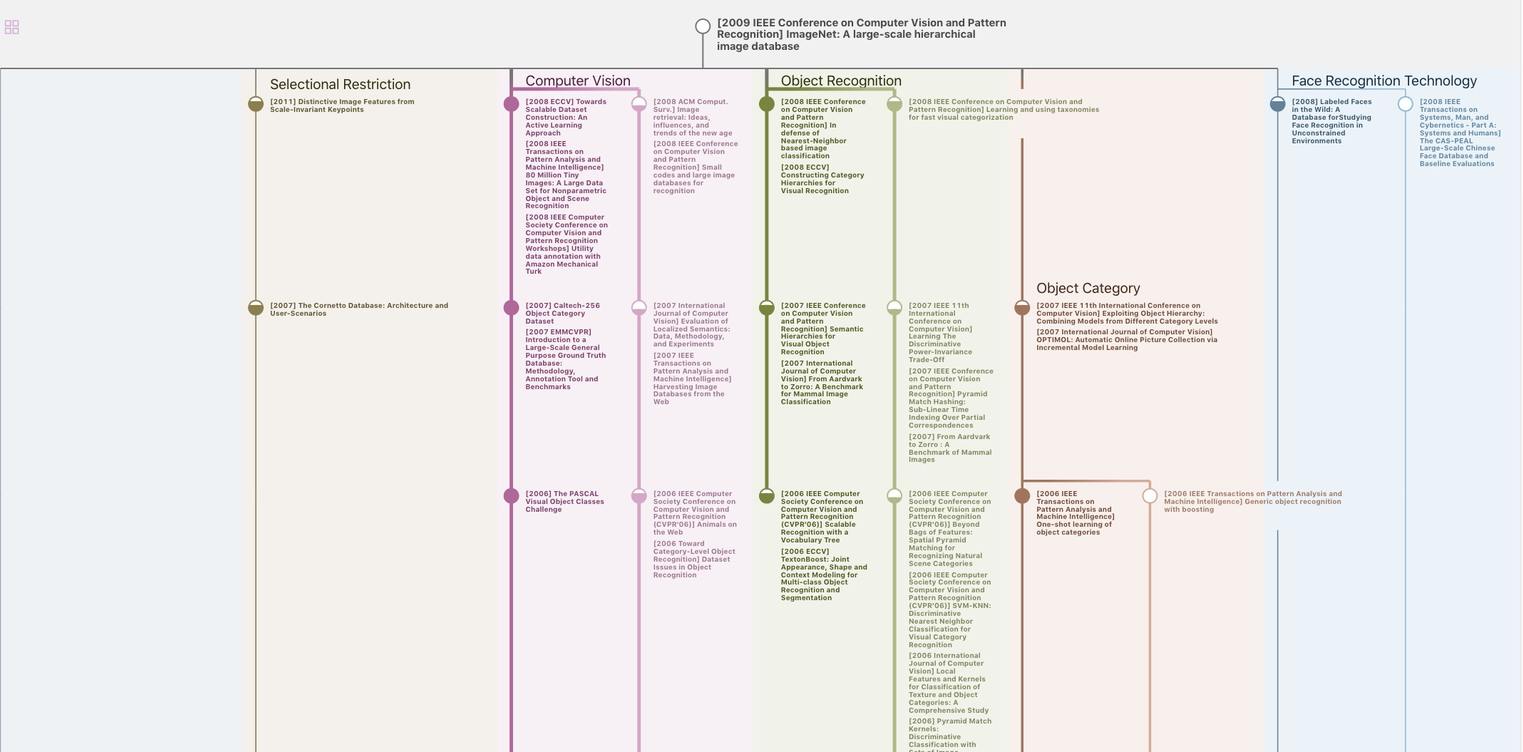
生成溯源树,研究论文发展脉络
Chat Paper
正在生成论文摘要