TransUPR: A Transformer-based Uncertain Point Refiner for LiDAR Point Cloud Semantic Segmentation
arxiv(2023)
摘要
In this work, we target the problem of uncertain points refinement for image-based LiDAR point cloud semantic segmentation (LiDAR PCSS). This problem mainly results from the boundary-blurring problem of convolution neural networks (CNNs) and quantitation loss of spherical projection, which are often hard to avoid for common image-based LiDAR PCSS approaches. We propose a plug-and-play transformer-based uncertain point refiner (TransUPR) to address the problem. Through local feature aggregation, uncertain point localization, and self-attention-based transformer design, TransUPR, integrated into an existing range image-based LiDAR PCSS approach (e.g., CENet), achieves the state-of-the-art performance (68.2% mIoU) on Semantic-KITTI benchmark, which provides a performance improvement of 0.6% on the mIoU.
更多查看译文
关键词
lidar point,uncertain point refiner,cloud semantic segmentation,transformer-based
AI 理解论文
溯源树
样例
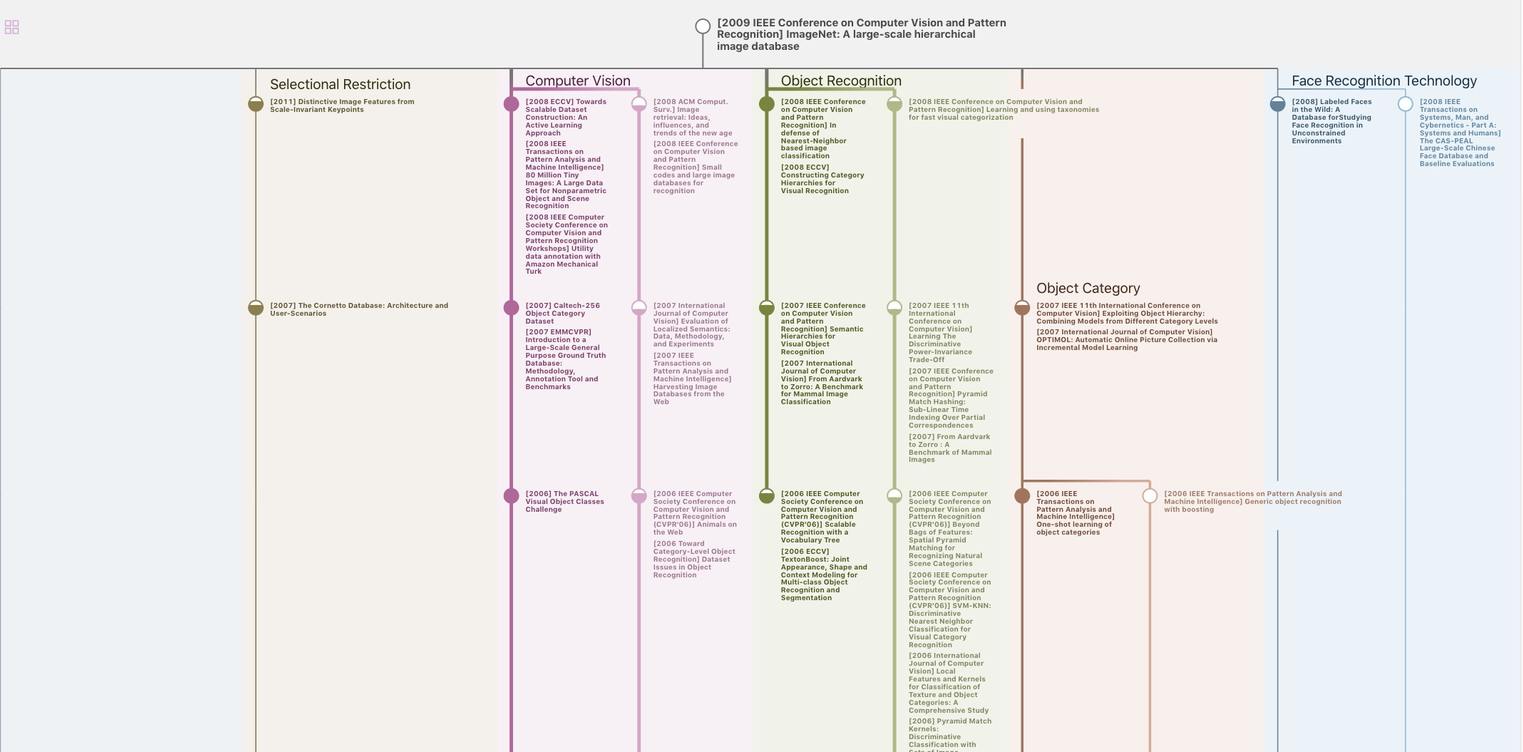
生成溯源树,研究论文发展脉络
Chat Paper
正在生成论文摘要