A Bayesian approach for estimating the survivor average causal effect when outcomes are truncated by death in cluster-randomized trials.
American journal of epidemiology(2023)
摘要
Many studies encounter clustering due to multicenter enrollment and non-mortality outcomes, such as quality-of-life, that are truncated due to death; i.e., missing not at random and nonignorable. Traditional missing data methods and target causal estimands are suboptimal for statistical inference in the presence of these combined issues, which are especially common in multicenter studies and cluster-randomized trials (CRTs) among the elderly or seriously ill. Using principal stratification, we developed a Bayesian estimator that jointly identifies the always-survivor principal stratum in a clustered/hierarchical data setting and estimates the average treatment effect among them (i.e., the survivor average causal effect, SACE). In simulations, we observed low bias and good coverage with our method. In a motivating CRT, the SACE and the estimate from complete case analysis differed in magnitude, but both were small, and neither was incompatible with a null effect. However, the SACE estimate has a clear causal interpretation. The option to assess the rigorously defined SACE estimand in studies with informative truncation and clustering can provide additional insight into an important subset of study participants. Based on the simulation study and CRT reanalysis, we provide practical recommendations for using the SACE in CRTs and code to support future research.
更多查看译文
关键词
Bayesian estimation,Cluster-randomized trials,always survivors,counterfactual outcomes,death truncation,quality of life,survivor average causal effect
AI 理解论文
溯源树
样例
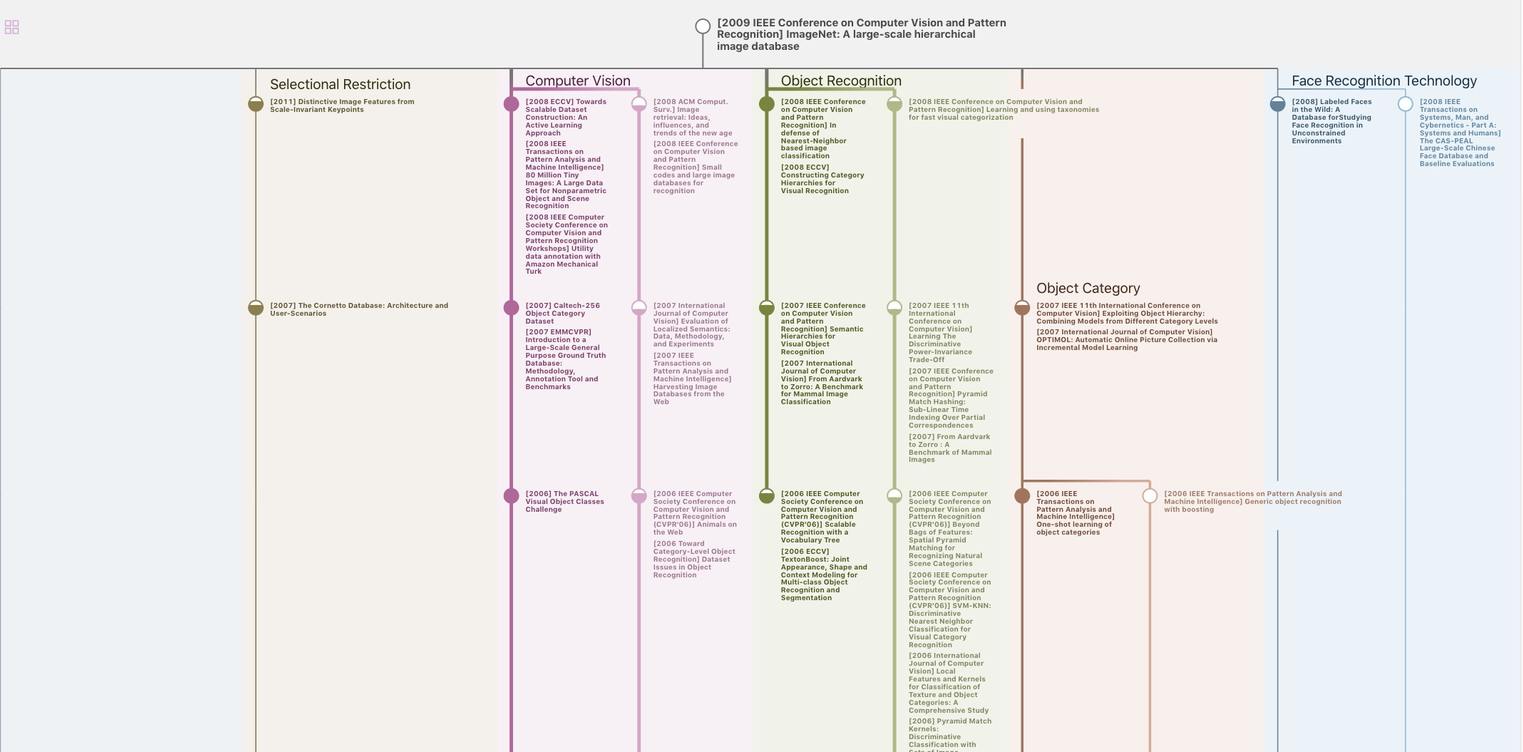
生成溯源树,研究论文发展脉络
Chat Paper
正在生成论文摘要