Machine Learning-Driven Snow Cover Mapping Techniques using Google Earth Engine
2022 IEEE 19th India Council International Conference (INDICON)(2022)
摘要
Snow cover is a significant factor influencing the surface albedo. Thus an accurate snow cover mapping algorithm is of utmost importance in energy balance, land surface and climate models. Traditional methods utilizing satellite imagery to determine snow cover used snow indices such as Normalized Difference Snow Index, Normalized Difference Forest Snow Index and S3 index. Recent studies have demonstrated improved performance of snow mapping using machine learning-driven supervised classification techniques. Through this study, the authors present a set of algorithms developed using Google Earth Engine for spatial and temporal extension of machine learning-driven snow cover estimation. Further, the study evaluates the use of machine learning models viz. Classification and Regression Tree (CART), Support Vector Machine (SVM), Gradient Tree Boosting and Random Forest for the generation of snow cover maps using accuracy metrics such as overall accuracy, producer accuracy, consumer accuracy, and kappa coefficient. Gradient Tree Boosting consistently depicted the best spatial and temporal accuracies. All machine learning classifiers performed better than the conventional index-derived snow cover maps.
更多查看译文
关键词
Snow cover,Classification and Regression Tree,Support Vector Machine,Gradient Tree Boosting,Random Forest,Google Earth Engine,Supervised classification
AI 理解论文
溯源树
样例
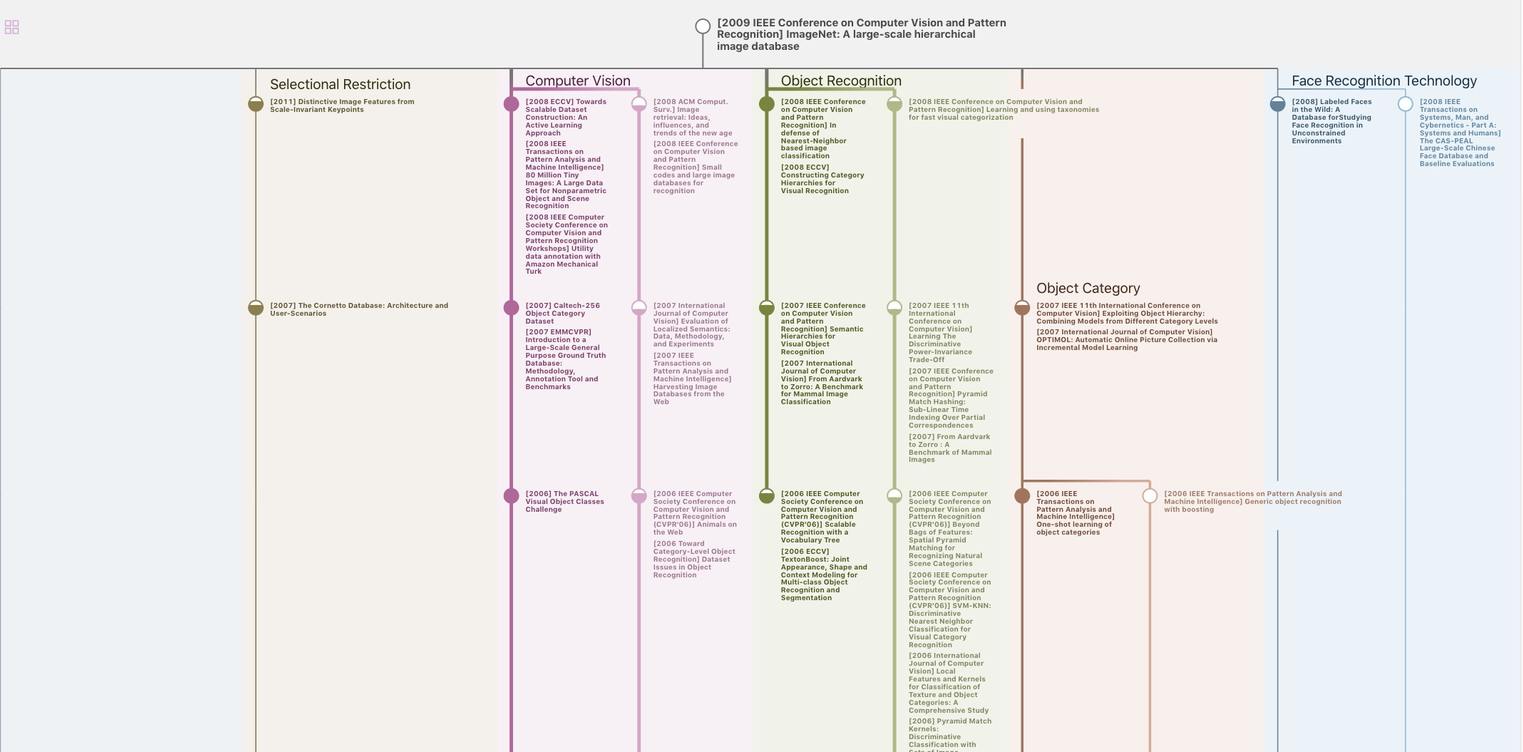
生成溯源树,研究论文发展脉络
Chat Paper
正在生成论文摘要