Leveraging Deep Reinforcement Learning With Attention Mechanism for Virtual Network Function Placement and Routing
IEEE Transactions on Parallel and Distributed Systems(2023)
摘要
The efficacy of Network Function Virtualization (NFV) depends critically on (1) where the virtual network functions (VNFs) are placed and (2) how the traffic is routed. Unfortunately, these aspects are not easily optimized, especially under time-varying network states with different QoS requirements. Given the importance of NFV, many approaches have been proposed to solve the VNF placement and Service Function Chaining (SFC) routing problem. However, those prior approaches mainly assume that the network state is static and known, disregarding dynamic network variations. To bridge that gap, we leverage Markov Decision Process (MDP) to model the dynamic network state transitions. To jointly minimize the delay and cost of NFV providers and maximize the revenue, we first devise a customized Deep Reinforcement Learning (DRL) algorithm for the VNF placement problem. The algorithm uses the attention mechanism to ascertain smooth network behavior within the general framework of network utility maximization (NUM). We then propose attention mechanism-based DRL algorithm for the SFC routing problem, which is to find the path to deliver traffic for the VNFs placed on different nodes. The simulation results show that our proposed algorithms outperform the state-of-the-art algorithms in terms of network utility, delay, cost, and acceptance ratio.
更多查看译文
关键词
Deep reinforcement learning,network function virtualization,placement,routing
AI 理解论文
溯源树
样例
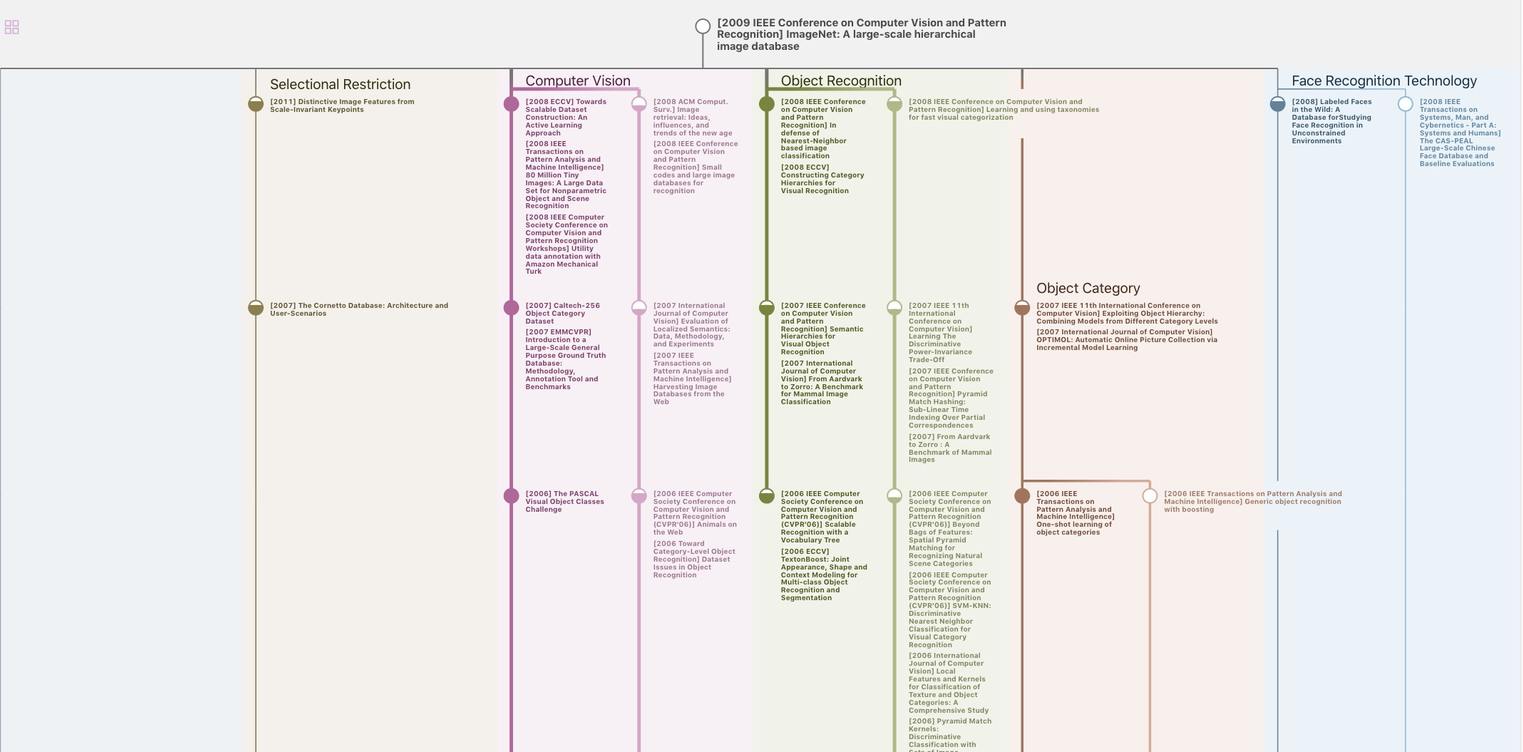
生成溯源树,研究论文发展脉络
Chat Paper
正在生成论文摘要