Linear Bandits with Memory: from Rotting to Rising
arxiv(2023)
摘要
Nonstationary phenomena, such as satiation effects in recommendation, are a common feature of sequential decision-making problems. While these phenomena have been mostly studied in the framework of bandits with finitely many arms, in many practically relevant cases linear bandits provide a more effective modeling choice. In this work, we introduce a general framework for the study of nonstationary linear bandits, where current rewards are influenced by the learner's past actions in a fixed-size window. In particular, our model includes stationary linear bandits as a special case. After showing that the best sequence of actions is NP-hard to compute in our model, we focus on cyclic policies and prove a regret bound for a variant of the OFUL algorithm that balances approximation and estimation errors. Our theoretical findings are supported by experiments (which also include misspecified settings) where our algorithm is seen to perform well against natural baselines.
更多查看译文
关键词
memory
AI 理解论文
溯源树
样例
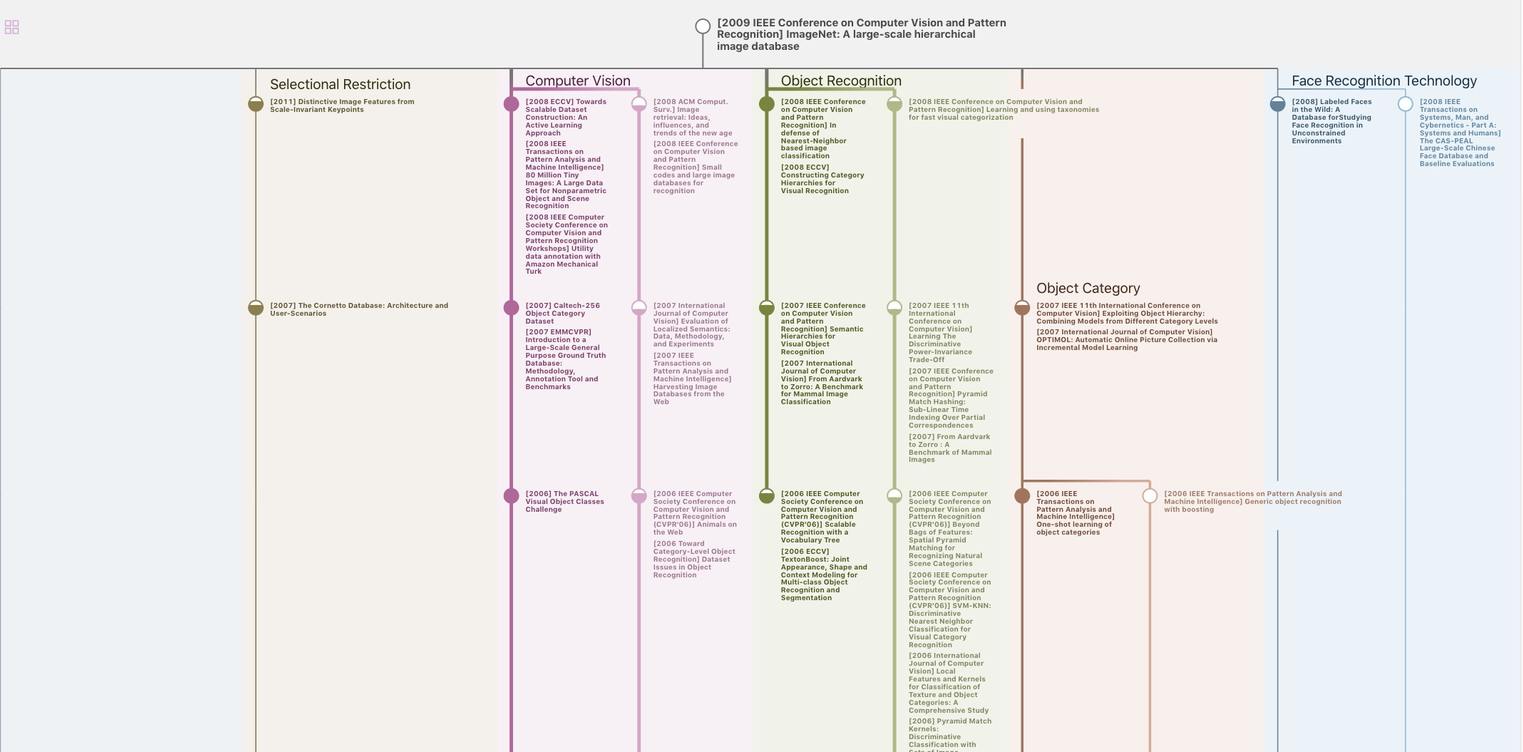
生成溯源树,研究论文发展脉络
Chat Paper
正在生成论文摘要