An Attention-based Approach to Hierarchical Multi-label Music Instrument Classification
arxiv(2023)
摘要
Although music is typically multi-label, many works have studied hierarchical music tagging with simplified settings such as single-label data. Moreover, there lacks a framework to describe various joint training methods under the multi-label setting. In order to discuss the above topics, we introduce hierarchical multi-label music instrument classification task. The task provides a realistic setting where multi-instrument real music data is assumed. Various hierarchical methods that jointly train a DNN are summarized and explored in the context of the fusion of deep learning and conventional techniques. For the effective joint training in the multi-label setting, we propose two methods to model the connection between fine- and coarse-level tags, where one uses rule-based grouped max-pooling, the other one uses the attention mechanism obtained in a data-driven manner. Our evaluation reveals that the proposed methods have advantages over the method without joint training. In addition, the decision procedure within the proposed methods can be interpreted by visualizing attention maps or referring to fixed rules.
更多查看译文
关键词
instrument,classification,music,attention-based,multi-label
AI 理解论文
溯源树
样例
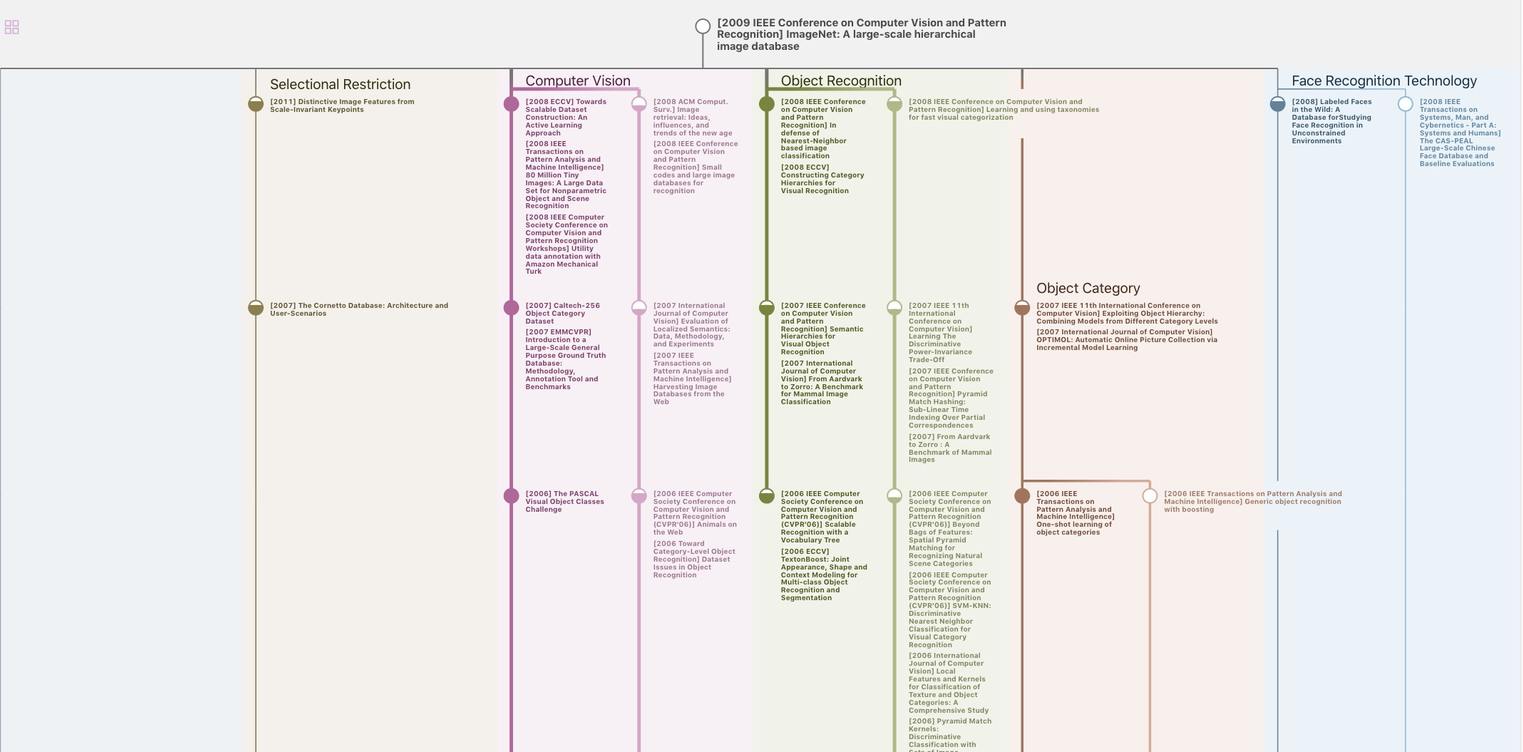
生成溯源树,研究论文发展脉络
Chat Paper
正在生成论文摘要