Instance-specific linear relaxations of semidefinite optimization problems
arxiv(2023)
摘要
We introduce a generic technique to obtain linear relaxations of semidefi-
nite programs with provable guarantees based on the commutativity of the
constraint and the objective matrices. We study conditions under which the
optimal value of the SDP and the proposed linear relaxation match, which we
then relax to provide a flex- ible methodology to derive effective linear
relaxations. We specialize these results to provide linear programs that
approximate well-known semidefinite programs for the max cut problem proposed
by Poljak and Rendl, and the Lovasz theta number; we prove that the linear
program proposed for max cut certifies a known eigenvalue bound for the maximum
cut value and is in fact stronger. Our ideas can be used to warm-start
algorithms that solve semidefinite programs by iterative polyhedral ap-
proximation of the feasible region. We verify this capability through multiple
exper- iments on the max cut semidefinite program, the Lovasz theta number and
on three families of semidefinite programs obtained as convex relaxations of
certain quadrati- cally constrained quadratic problems
更多查看译文
关键词
semidefinite optimization
AI 理解论文
溯源树
样例
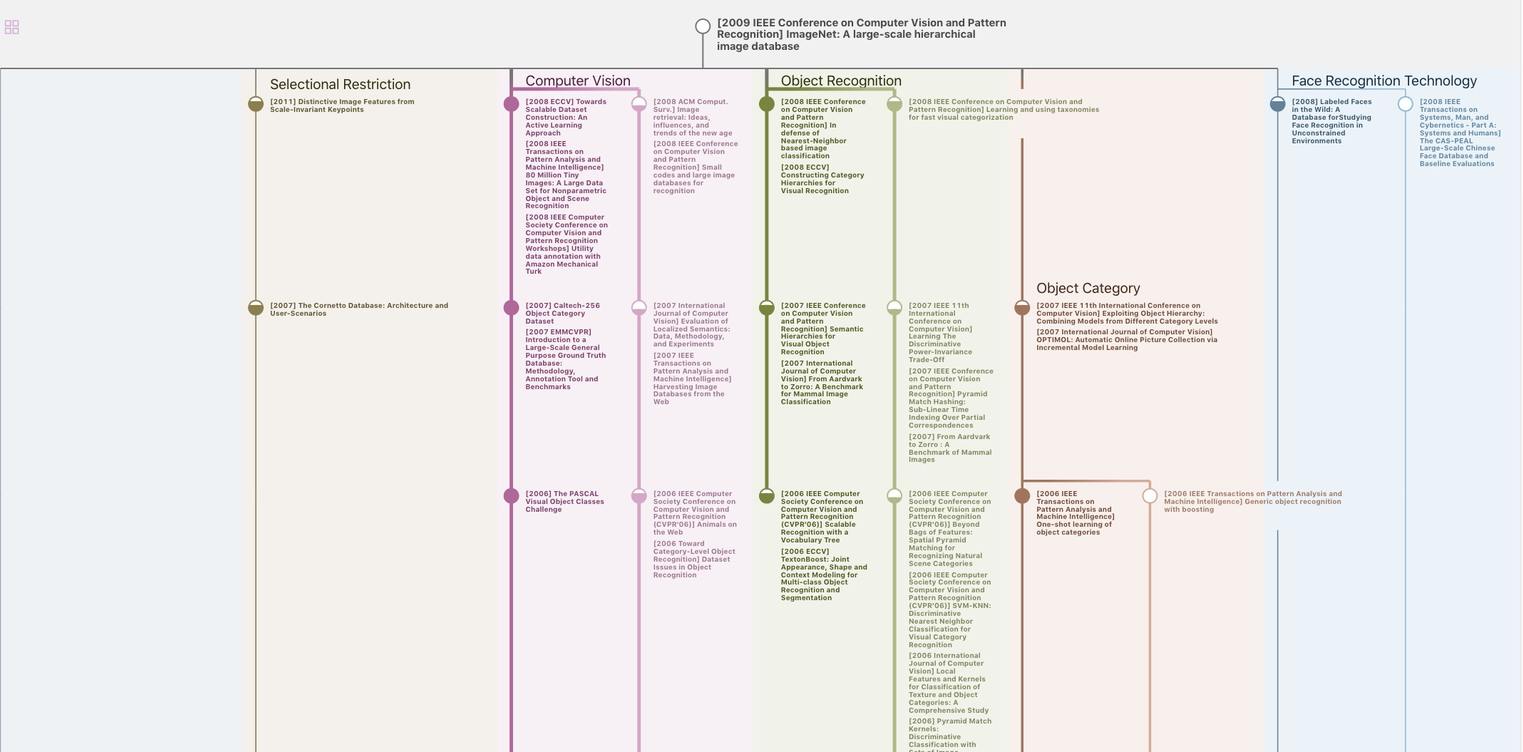
生成溯源树,研究论文发展脉络
Chat Paper
正在生成论文摘要