A Lightweight Broad Learning System for Wireless Traffic Prediction
2022 14th International Conference on Wireless Communications and Signal Processing (WCSP)(2022)
摘要
Currently, traffic trends to grow explosively under the scenarios of advanced wireless communication networks and diversified services. How to design suitable model to accurately and efficiently predict wireless traffic has become a significant technical challenge. To get over this dilemma, this paper proposes a lightweight broad learning system (LBLS) for wireless traffic prediction. Specifically, the LBLS firstly adopts restricted Boltzmann machine (RBM) to perform feature extraction. Then, gated recurrent unit (G RU) is introduced into enhancement layer to describe temporal relations of multivariate time series. Finally, elastic-net considering both
$L$
1-norm and
$L_{2}$
-norm regularization is used to realize connection weight estimation. Experimental results on two typical traffic datasets show that the proposed LBLS can not only reduce the complexity of existing BLS and its variants, but also has strong modeling and prediction ability for wireless traffic prediction.
更多查看译文
关键词
wireless traffic prediction,broad learning system,lightweight
AI 理解论文
溯源树
样例
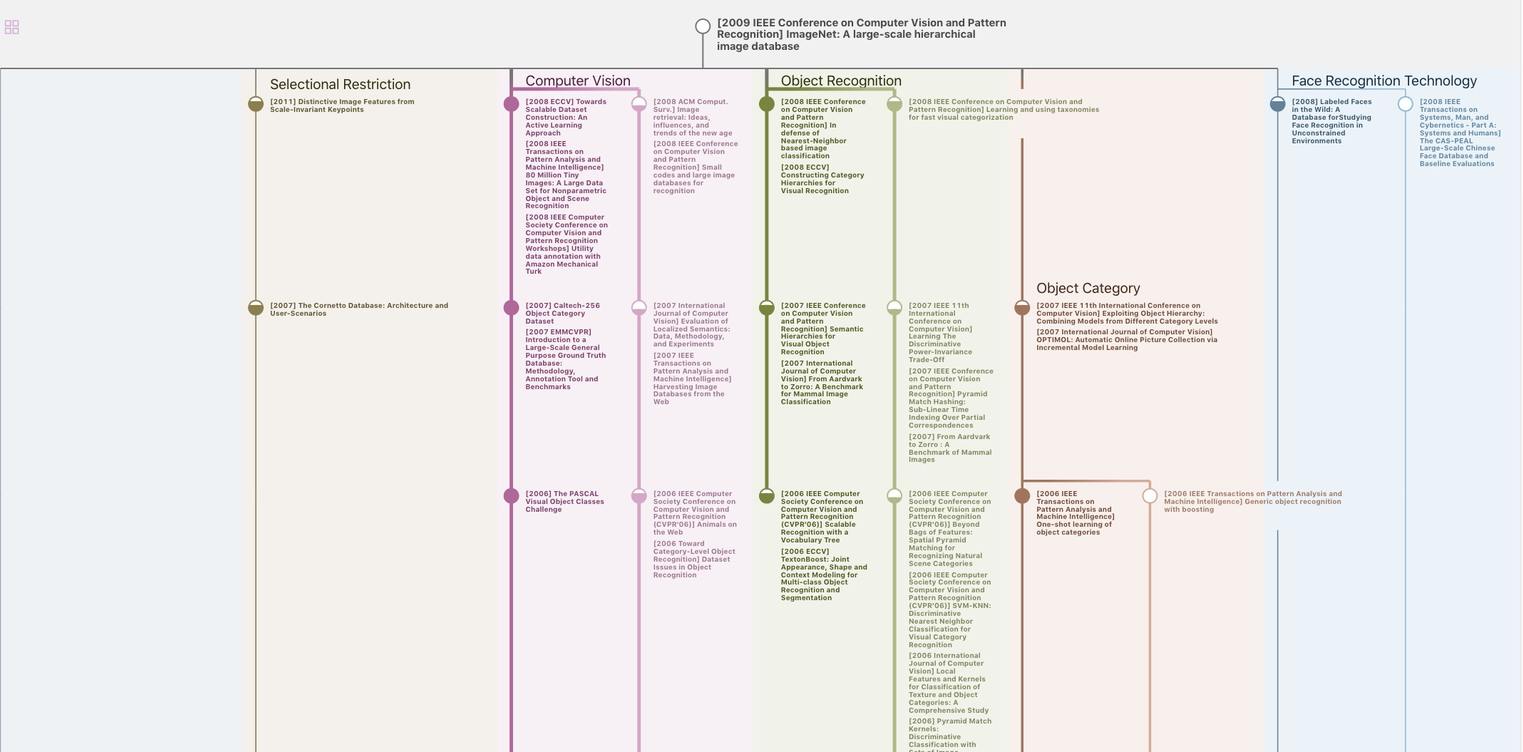
生成溯源树,研究论文发展脉络
Chat Paper
正在生成论文摘要