Low-Cost Traffic Perception for Road Detector Data Estimation: A Deep Learning Approach
2022 14th International Conference on Wireless Communications and Signal Processing (WCSP)(2022)
摘要
The road network data collected by the traffic detection system is essential to the modern intelligent transportation systems (ITS). Generally, roadside sensors are the primary approach to obtaining traffic data. However, low-cost traffic sensing is a challenge as obtaining complete traffic network information often requires expensive detector equipment. Therefore, it is critical to deploy the sensors sparsely to obtain low-cost detector deployment methods on the road network. In this paper, we investigate the relationship between the sparsity of detectors and the perceived accuracy of road traffic flow in urban road networks. Specifically, we remove a portion of the sensors in the road network to obtain a sparse sensor layout. Then, the transformer algorithm is used to estimate the data of the removed virtual sensors from the data of the unremoved actual sensors. In the simulation experiment, we build a classic road network model in the AIMSUN simulation software to recover virtual detector data. The simulation results verify that it is possible to use 35% of the original detector to monitor the traffic condition of the whole urban road network and the estimation accuracy can reach 3 m/s.
更多查看译文
关键词
Speed estimation,low-cost traffic perception,deep learning,the Sioux Falls network
AI 理解论文
溯源树
样例
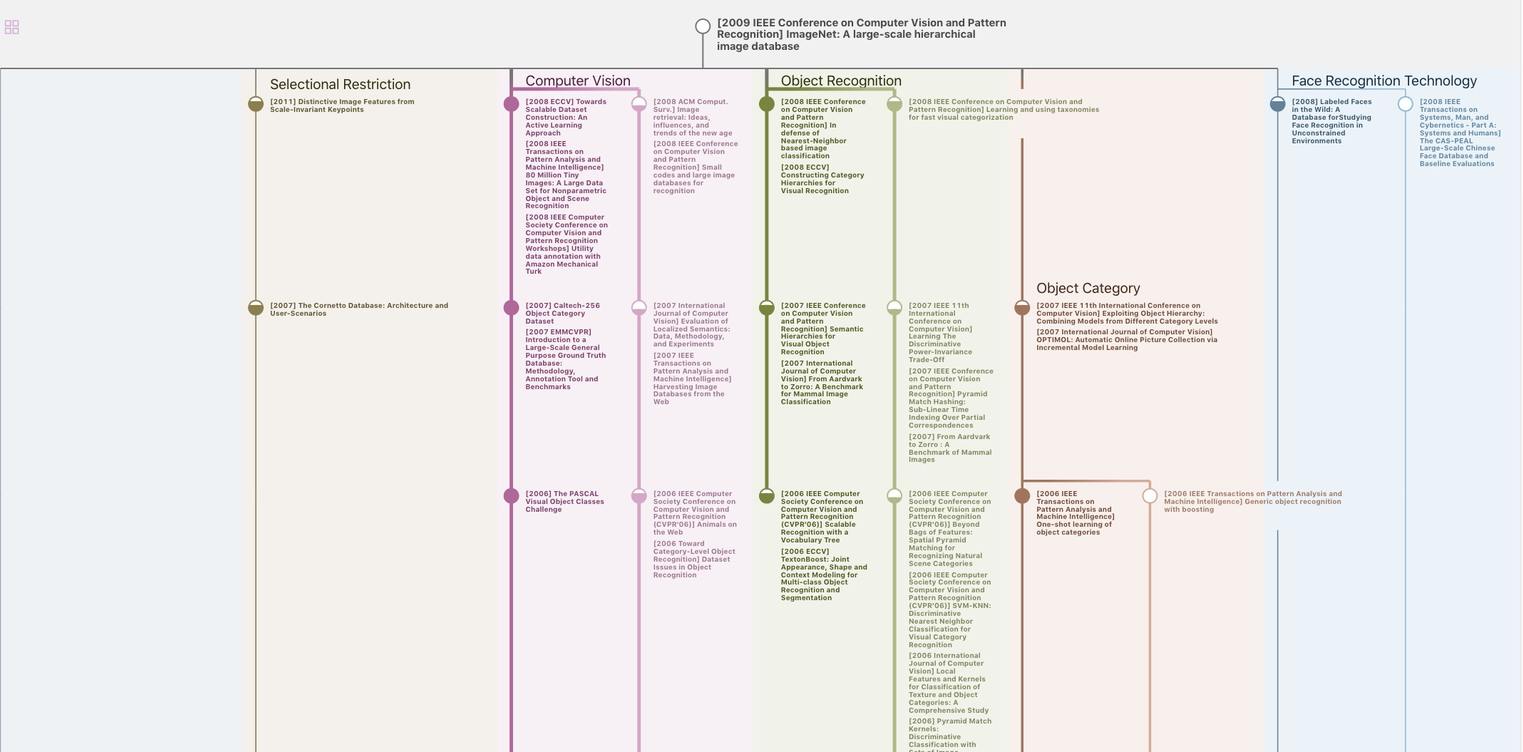
生成溯源树,研究论文发展脉络
Chat Paper
正在生成论文摘要