Evaluating Language Characteristics and Related Gender Bias in Letters of Recommendation for Hand Surgery Fellowship.
The Journal of hand surgery(2023)
摘要
PURPOSE:Letters of recommendation (LORs) function as an indicator of competence and future potential for a trainee. Our purpose was to evaluate gender bias in hand surgery fellowship applicant LORs.
METHODS:This was a retrospective study of all LORs submitted to a hand surgery fellowship program between 2015 and 2020. Demographic data about applicants and letter writers were collected. Linguistic analysis was performed using a text analysis software, and results were evaluated with nonparametric tests, multiple linear regression models, and a mixed effects regression model.
RESULTS:Letters of recommendation were analyzed; 720 letters for 188 (23.4%) female applicants and 2,337 letters for 616 (76.6%) male applicants. Compared with LORs written for men, those written for women had more references to categories of anxiety (eg, worried and fearful) and affiliation (eg, ally and friend). Letters for women had more "clout." In subgroup analysis, letters for female plastic surgery applicants had more words signaling power, whereas recommendations for female applicants from orthopedic residencies had more words related to anxiety, achievement, work, and leisure.
CONCLUSIONS:Letters of recommendation written for female residents applying to hand fellowship had more references to anxiety but were written with higher clout and more words of affiliation. Subgroup analysis looking at orthopedic and plastic surgery applicants separately found a mixed picture. Overall, these LORs written for applicants to hand fellowship had no notable specific patterns of gender bias in our analyses.
CLINICAL RELEVANCE:Because programs look to train the next generation of hand surgeons, alerting letter readers to trends in implicit bias may help in the selection of qualified applicants. Bringing topics of implicit bias forward may help writers think more critically about word choice and topics.
更多查看译文
AI 理解论文
溯源树
样例
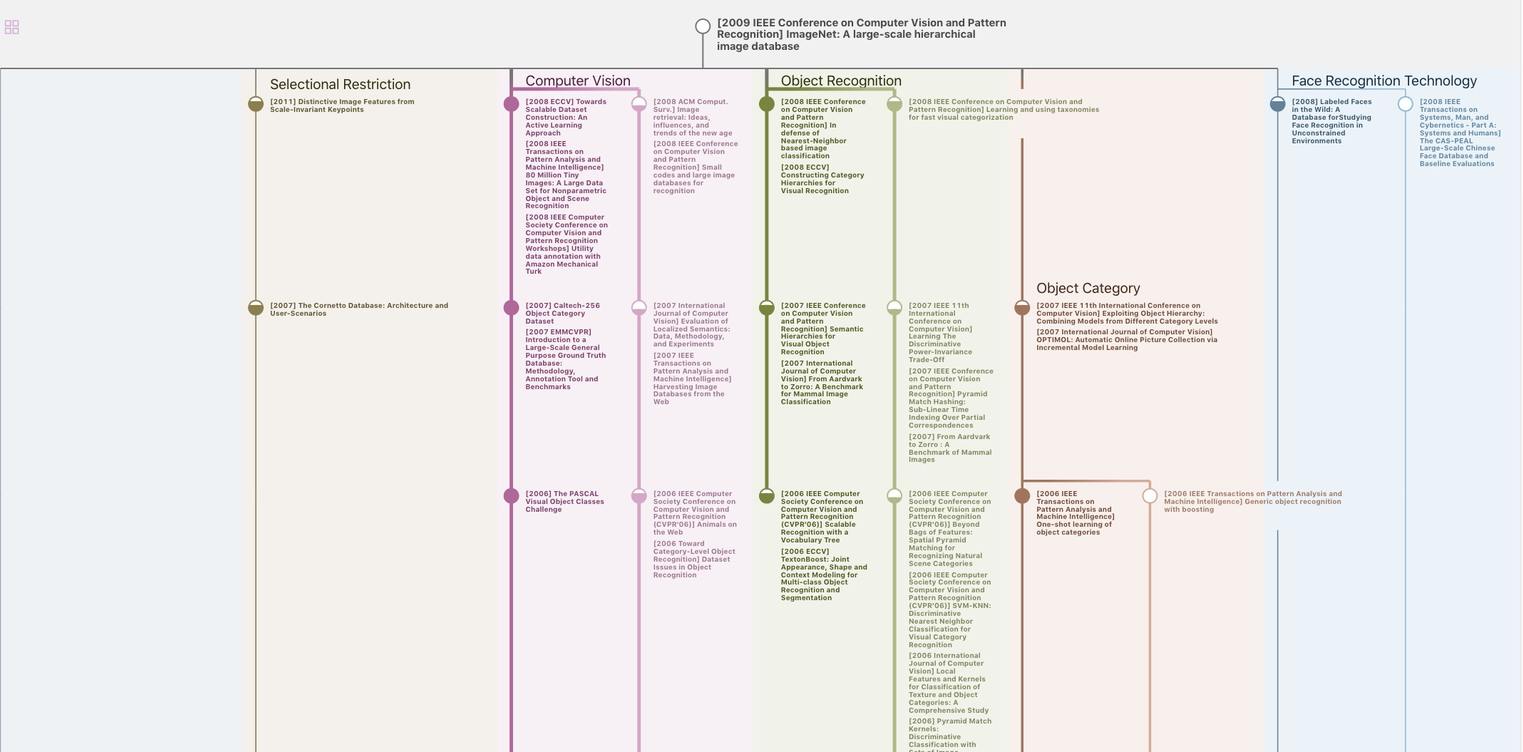
生成溯源树,研究论文发展脉络
Chat Paper
正在生成论文摘要