Do Deep Neural Networks Capture Compositionality in Arithmetic Reasoning?
arxiv(2023)
摘要
Compositionality is a pivotal property of symbolic reasoning. However, how well recent neural models capture compositionality remains underexplored in the symbolic reasoning tasks. This study empirically addresses this question by systematically examining recently published pre-trained seq2seq models with a carefully controlled dataset of multi-hop arithmetic symbolic reasoning. We introduce a skill tree on compositionality in arithmetic symbolic reasoning that defines the hierarchical levels of complexity along with three compositionality dimensions: systematicity, productivity, and substitutivity. Our experiments revealed that among the three types of composition, the models struggled most with systematicity, performing poorly even with relatively simple compositions. That difficulty was not resolved even after training the models with intermediate reasoning steps.
更多查看译文
关键词
deep neural,deep neural networks,neural networks,arithmetic
AI 理解论文
溯源树
样例
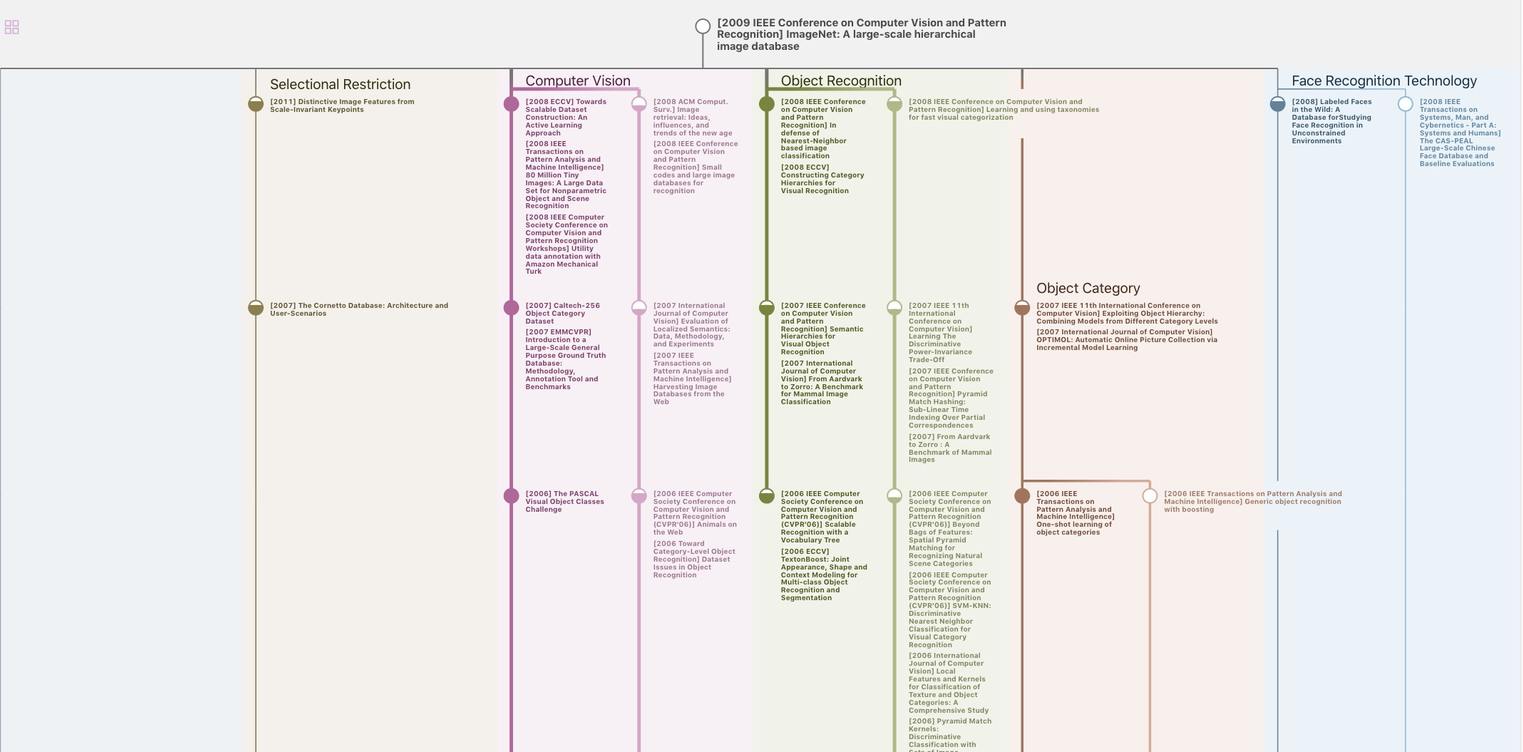
生成溯源树,研究论文发展脉络
Chat Paper
正在生成论文摘要