Dataset Interfaces: Diagnosing Model Failures Using Controllable Counterfactual Generation
arxiv(2023)
摘要
Distribution shifts are a major source of failure of deployed machine learning models. However, evaluating a model's reliability under distribution shifts can be challenging, especially since it may be difficult to acquire counterfactual examples that exhibit a specified shift. In this work, we introduce dataset interfaces: a framework which allows users to scalably synthesize such counterfactual examples from a given dataset. Specifically, we represent each class from the input dataset as a custom token within the text space of a text-to-image diffusion model. By incorporating these tokens into natural language prompts, we can then generate instantiations of objects in that dataset under desired distribution shifts. We demonstrate how applying our framework to the ImageNet dataset enables us to study model behavior across a diverse array of shifts, including variations in background, lighting, and attributes of the objects themselves. Code available at https://github.com/MadryLab/dataset-interfaces.
更多查看译文
关键词
model failures
AI 理解论文
溯源树
样例
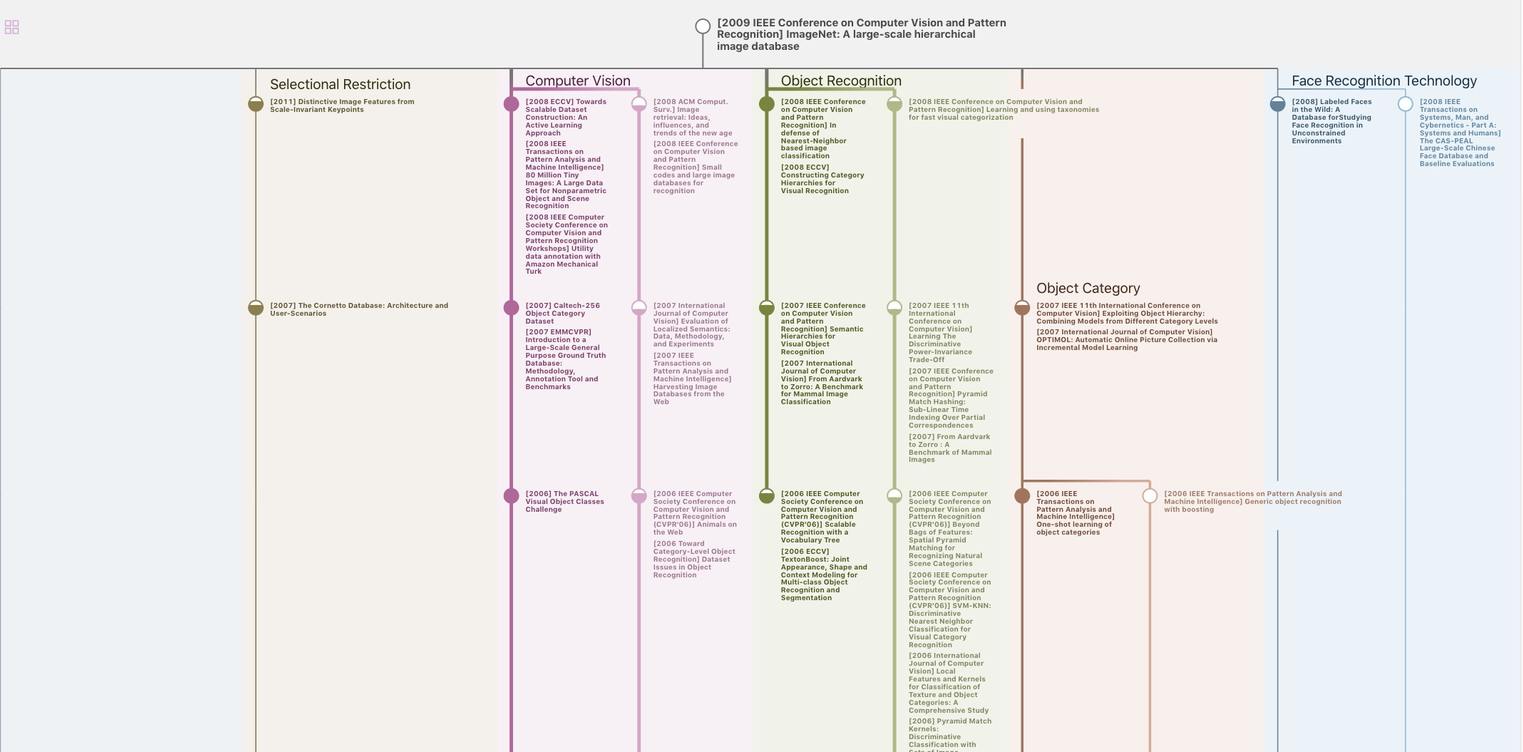
生成溯源树,研究论文发展脉络
Chat Paper
正在生成论文摘要