SupSiam: Non-contrastive Auxiliary Loss for Learning from Molecular Conformers
arxiv(2023)
摘要
We investigate Siamese networks for learning related embeddings for augmented samples of molecular conformers. We find that a non-contrastive (positive-pair only) auxiliary task aids in supervised training of Euclidean neural networks (E3NNs) and increases manifold smoothness (MS) around point-cloud geometries. We demonstrate this property for multiple drug-activity prediction tasks while maintaining relevant performance metrics, and propose an extension of MS to probabilistic and regression settings. We provide an analysis of representation collapse, finding substantial effects of task-weighting, latent dimension, and regularization. We expect the presented protocol to aid in the development of reliable E3NNs from molecular conformers, even for small-data drug discovery programs.
更多查看译文
关键词
molecular
AI 理解论文
溯源树
样例
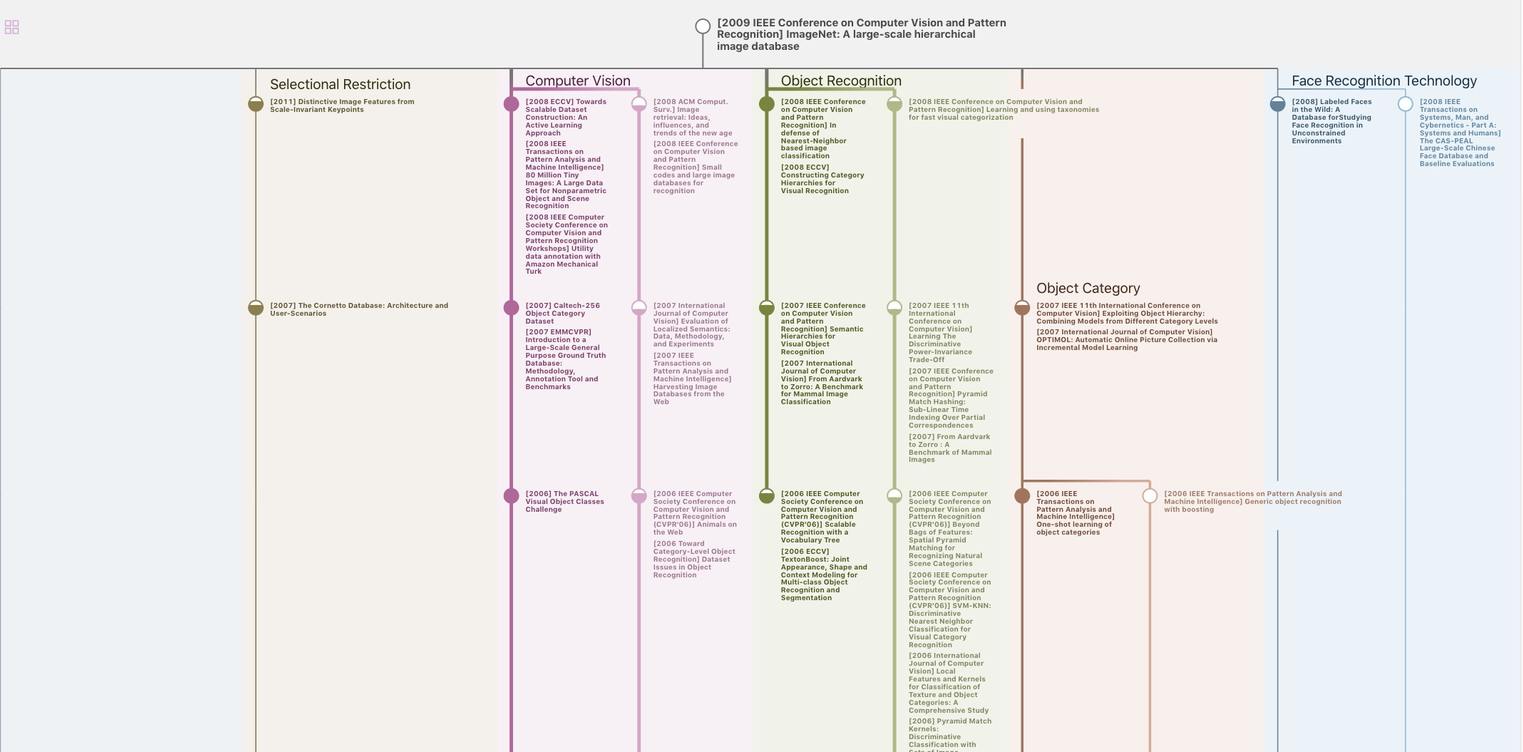
生成溯源树,研究论文发展脉络
Chat Paper
正在生成论文摘要