Multimap targeted free energy estimation
arxiv(2023)
摘要
We present a new method to compute free energies at a quantum mechanical (QM) level of theory from molecular simulations using cheap reference potential energy functions, such as force fields. To overcome the poor overlap between the reference and target distributions, we generalize targeted free energy perturbation (TFEP) to employ multiple configuration maps. While TFEP maps have been obtained before from an expensive training of a normalizing flow neural network (NN), our multimap estimator allows us to use the same set of QM calculations to both optimize the maps and estimate the free energy, thus removing almost completely the overhead due to training. A multimap extension of the multistate Bennett acceptance ratio estimator is also derived for cases where samples from two or more states are available. Furthermore, we propose a one-epoch learning policy that can be used to efficiently avoid overfitting when computing the loss function is expensive compared to generating data. Finally, we show how our multimap approach can be combined with enhanced sampling strategies to overcome the pervasive problem of poor convergence due to slow degrees of freedom. We test our method on the HiPen dataset of drug-like molecules and fragments, and we show that it can accelerate the calculation of the free energy difference of switching from a force field to a DFTB3 potential by about 3 orders of magnitude compared to standard FEP and by a factor of about 8 compared to previously published nonequilibrium calculations.
更多查看译文
关键词
free energy estimation,multimap
AI 理解论文
溯源树
样例
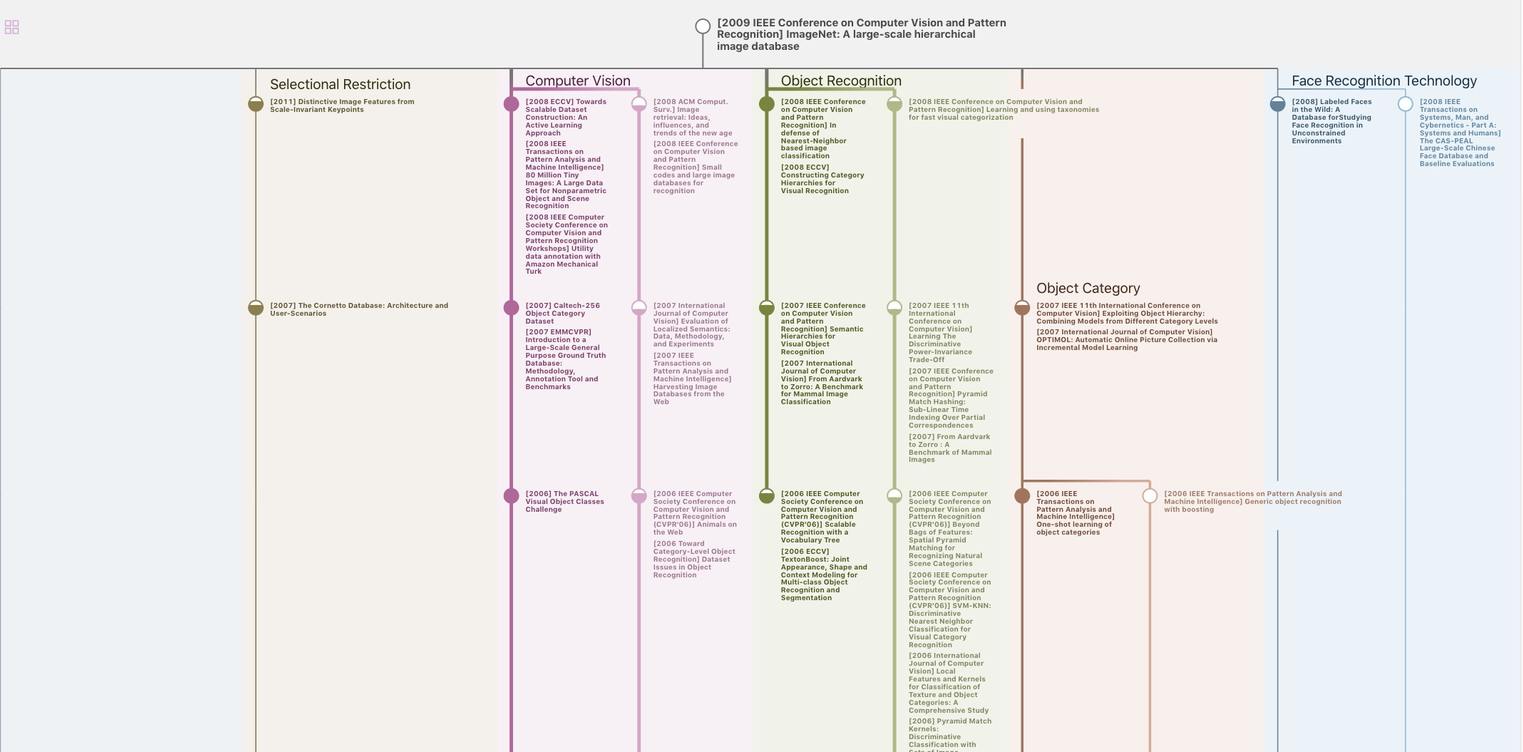
生成溯源树,研究论文发展脉络
Chat Paper
正在生成论文摘要