Are labels informative in semi-supervised learning? -- Estimating and leveraging the missing-data mechanism
arxiv(2023)
摘要
Semi-supervised learning is a powerful technique for leveraging unlabeled data to improve machine learning models, but it can be affected by the presence of ``informative'' labels, which occur when some classes are more likely to be labeled than others. In the missing data literature, such labels are called missing not at random. In this paper, we propose a novel approach to address this issue by estimating the missing-data mechanism and using inverse propensity weighting to debias any SSL algorithm, including those using data augmentation. We also propose a likelihood ratio test to assess whether or not labels are indeed informative. Finally, we demonstrate the performance of the proposed methods on different datasets, in particular on two medical datasets for which we design pseudo-realistic missing data scenarios.
更多查看译文
关键词
labels,learning,estimating,semi-supervised,missing-data
AI 理解论文
溯源树
样例
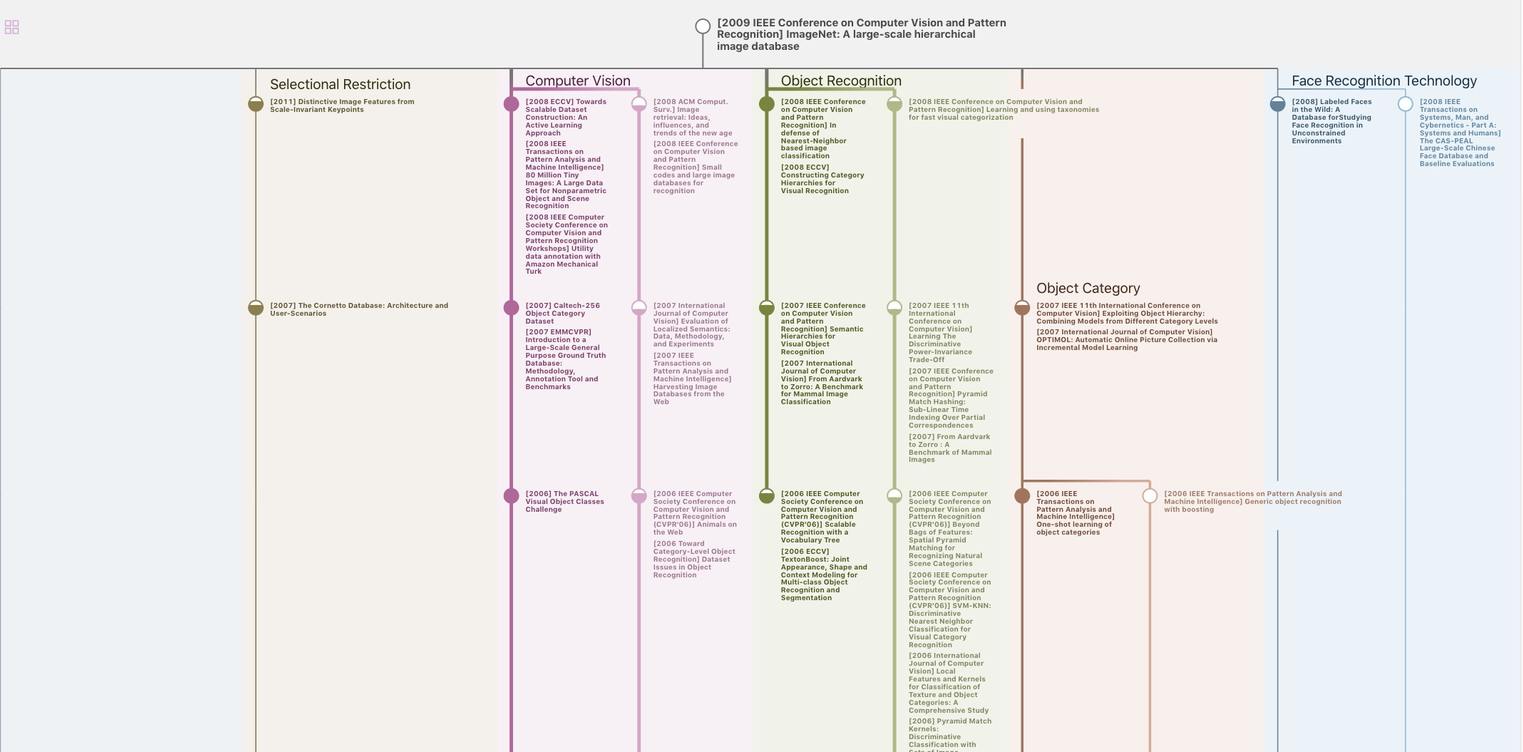
生成溯源树,研究论文发展脉络
Chat Paper
正在生成论文摘要