On the Nature of Disks at High Redshift Seen by JWST/CEERS with Contrastive Learning and Cosmological Simulations
arxiv(2024)
摘要
Visual inspections of the first optical rest-frame images from JWST have indicated a surprisingly high fraction of disk galaxies at high redshifts. Here, we alternatively apply self-supervised machine learning to explore the morphological diversity at z >= 3. Our proposed data-driven representation scheme of galaxy morphologies, calibrated on mock images from the TNG50 simulation, is shown to be robust to noise and to correlate well with the physical properties of the simulated galaxies, including their 3D structure. We apply the method simultaneously to F200W and F356W galaxy images of a mass-complete sample (M-*/M-circle dot > 10(9)) at 3 <= z <= 6 from the first JWST/NIRCam CEERS data release. We find that the simulated and observed galaxies do not exactly populate the same manifold in the representation space from contrastive learning. We also find that half the galaxies classified as disks-either convolutional neural network-based or visually-populate a similar region of the representation space as TNG50 galaxies with low stellar specific angular momentum and nonoblate structure. Although our data-driven study does not allow us to firmly conclude on the true nature of these galaxies, it suggests that the disk fraction at z >= 3 remains uncertain and possibly overestimated by traditional supervised classifications. Deeper imaging and spectroscopic follow-ups as well as comparisons with other simulations will help to unambiguously determine the true nature of these galaxies, and establish more robust constraints on the emergence of disks at very high redshift.
更多查看译文
关键词
Galaxy formation,Galaxy evolution,High-redshift galaxies,Neural networks
AI 理解论文
溯源树
样例
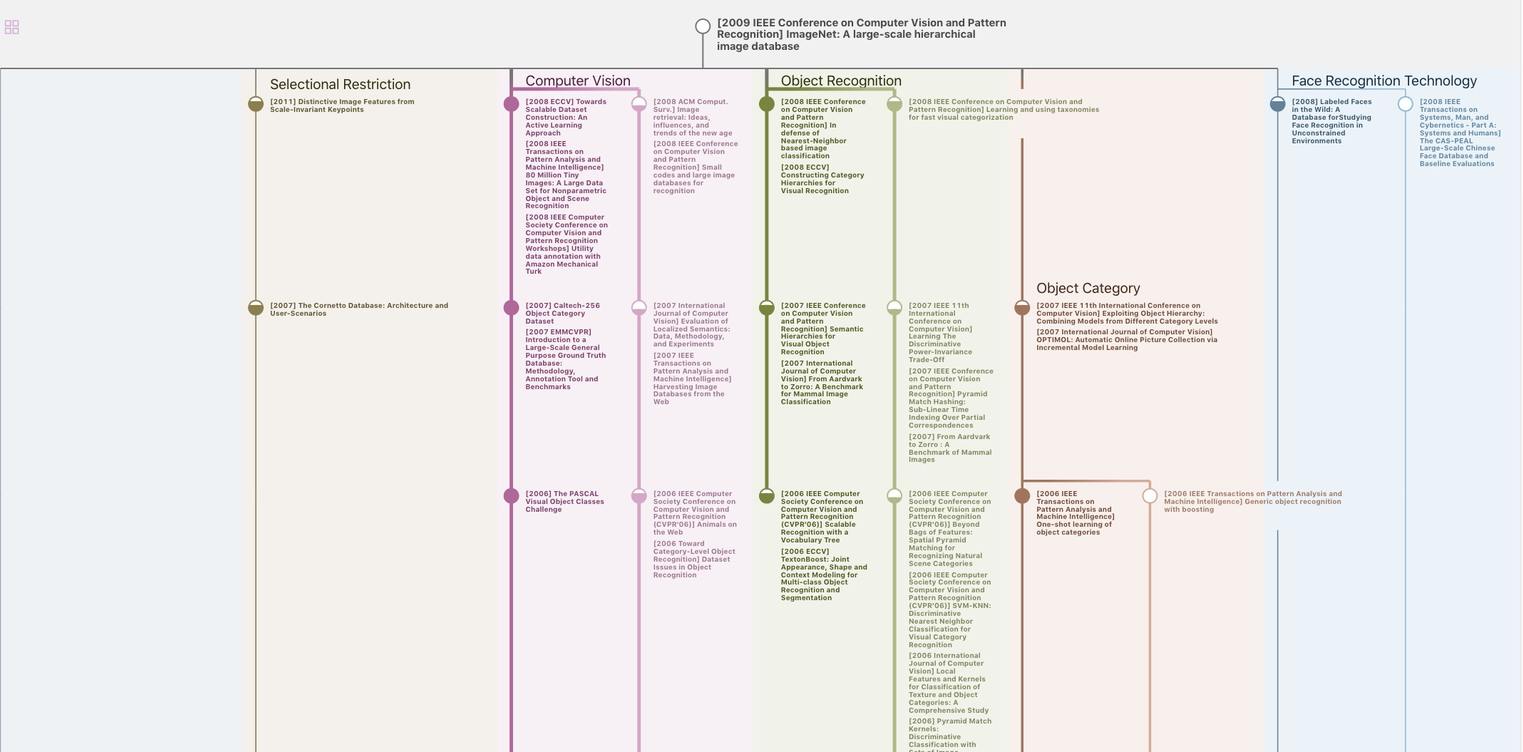
生成溯源树,研究论文发展脉络
Chat Paper
正在生成论文摘要