From Cell-Lines to Cancer Patients: Personalized Drug Synergy Prediction
biorxiv(2023)
摘要
Combination drug therapies are effective treatments for cancer. However, the genetic heterogeneity of the patients and exponentially large space of drug pairings pose significant challenges for finding the right combination for a specific patient. Current in silico prediction methods can be instrumental in reducing the vast number of candidate drug combinations. However, existing powerful methods are trained with cancer cell line gene expression data, which limits their applicability in clinical settings. While synergy measurements on cell lines models are available at large scale, patient-derived samples are too few to train a complex model. On the other hand, patient-specific single-drug response data are relatively more available. In this work, we propose a deep learning framework, Personalized Deep Synergy Predictor (PDSP), that enables us to use the patient-specific single drug response data for customizing patient drug synergy predictions. PDSP is first trained to learn synergy scores of drug pairs and their single drug presonses for a given cell line using drug structures and large scale cell line gene expression data. Then, the model is fine-tuned for patients with their patient gene expression data and associated single drug response measured on the patient ex vivo samples. In this study, we evaluate PDSP on data from three leukemia patients and observe that it improves the prediction accuracy by 27% compared to models trained on cancer cell line data. PDSP is built and available at https://github.com/hikuru/PDSP
### Competing Interest Statement
The authors have declared no competing interest.
更多查看译文
关键词
cancer patients,cell-lines cell-lines,drug,prediction
AI 理解论文
溯源树
样例
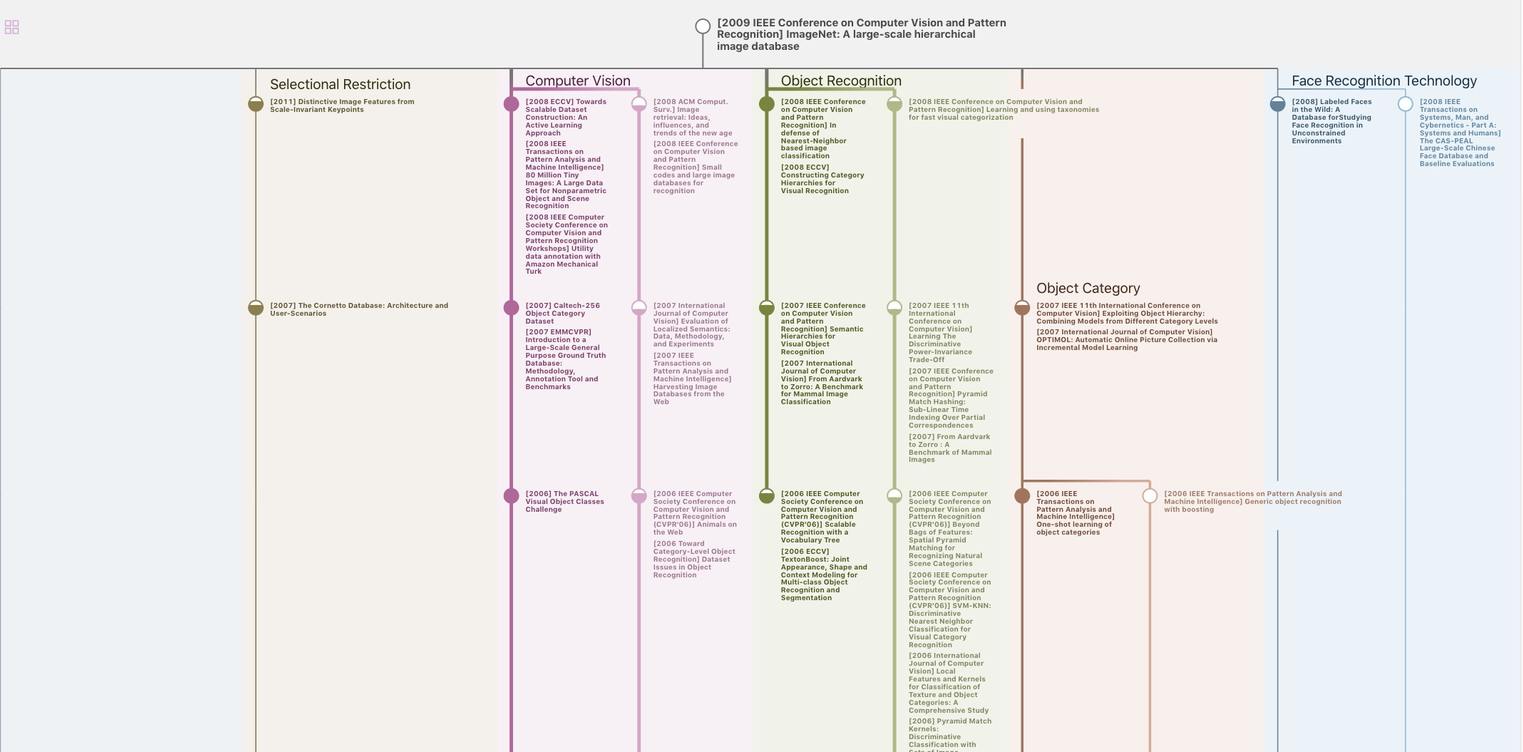
生成溯源树,研究论文发展脉络
Chat Paper
正在生成论文摘要