Class-oriented and label embedding analysis dictionary learning for pattern classification
MULTIMEDIA TOOLS AND APPLICATIONS(2022)
摘要
Analysis dictionary learning (ADL) has obtained lots of research interest in sparse representation-based classification recent years, due to its flexibility and low complexity for out-of-sample representation. However, the discrimination of the dominant analysis dictionary is not fully explored and the manifold information is not inherited into analysis atoms for classification. To remedy the deficiencies, we present joint class-oriented and label embedding (COLE) constraints on the analysis dictionary for pattern classification. Specifically, the comprehensive class-oriented constraints on the analysis subdictionaries efficiently yield discriminative class-wise atoms and between-class separable representation for classification. The redundant atoms can be eliminated by orthogonal subdictionary constraints, leading to a robust and within-class compact analysis dictionary. Furthermore, the label embedding term of analysis atoms inherits the supervised manifold information of the training samples and guarantees an ideal block-diagonal representation. Finally, an computationally efficient alternating direction minimization algorithm is presented with iterative reweighted and closed-form solutions, which avoids the time-consuming multiplication of class-specific data samples and the subdictionaries. Extensive experiments on five benchmark databases demonstrate at least comparable or better classification accuracy and efficiency of the proposed model compared with state-of-the-art ADL models.
更多查看译文
关键词
Analysis dictionary learning,Sparse representation,Incoherence promotion,Block-diagonal representation
AI 理解论文
溯源树
样例
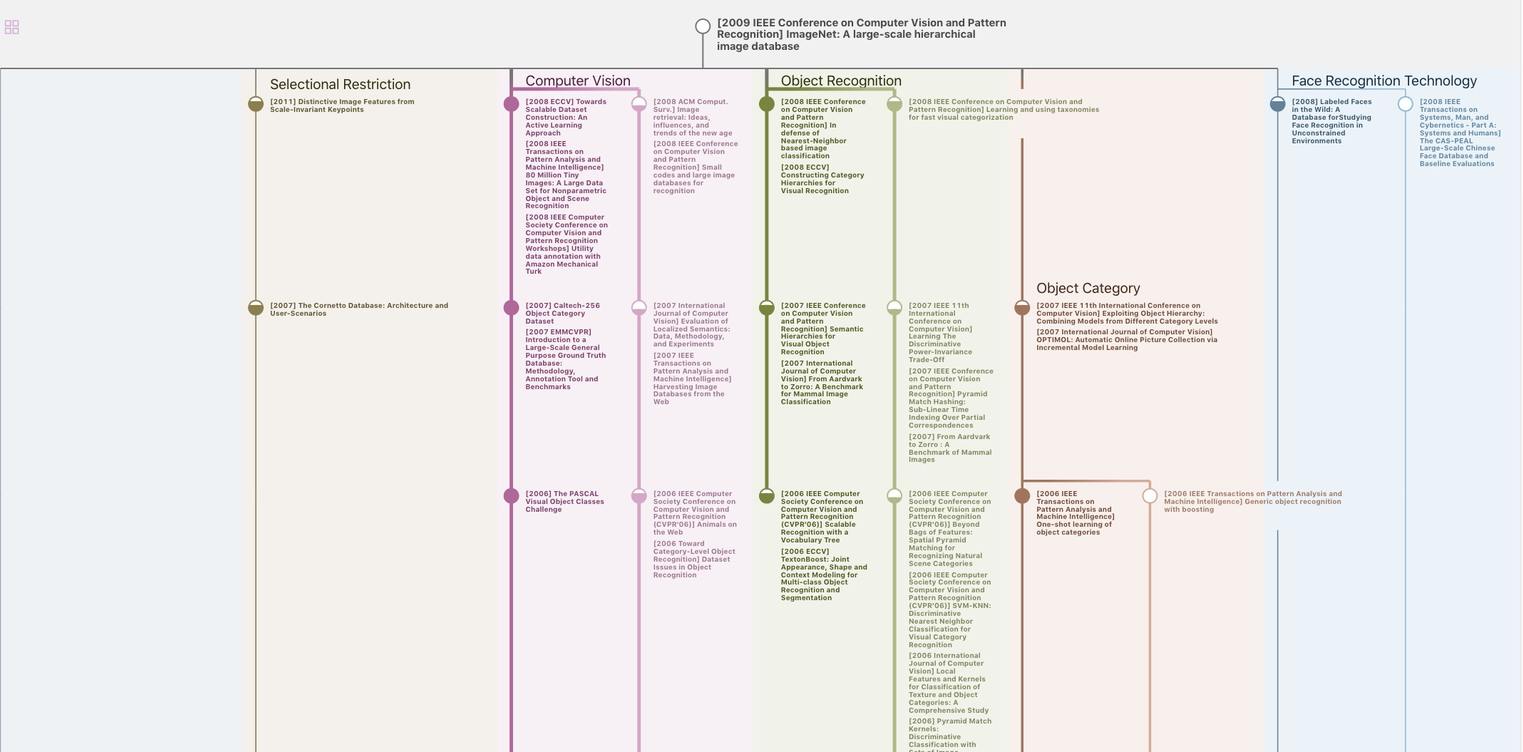
生成溯源树,研究论文发展脉络
Chat Paper
正在生成论文摘要