An improved near-real-time precipitation retrieval for Brazil
ATMOSPHERIC MEASUREMENT TECHNIQUES(2022)
摘要
Observations from geostationary satellites can provide spatially continuous coverage at continental scales with high spatial and temporal resolution. Because of this, they are commonly used to complement ground-based precipitation measurements, whose coverage is often more limited. We present Hydronn, a neural-network-based, near-real-time precipitation retrieval for Brazil based on visible and infrared (Vis-IR) observations from the Advanced Baseline Imager (ABI) on the Geostationary Operational Environmental Satellite 16 (GOES-16). The retrieval, which employs a convolutional neural network to perform Bayesian precipitation retrievals, was developed with the aims of (1) leveraging the full potential of latest-generation geostationary observations and (2) providing probabilistic precipitation estimates with well-calibrated uncertainties. The retrieval is trained using more than 3 years of collocations with combined radar and radiometer retrievals from the Global Precipitation Measurement (GPM) core observatory over South America. The accuracy of instantaneous precipitation estimates is assessed using aseparate year of GPM combined retrievals and compared to retrievals frompassive microwave (PMW) sensors and HYDRO, the Vis-IR retrieval that iscurrently in operational use at the Brazilian Institute for Space Research.Using all available channels of the ABI, Hydronn achieves accuracy closeto that of state-of-the-art PMW precipitation retrievals in both precipitation estimation and detection despite the lower information content of the Vis-IR observations. Hourly, daily, and monthly precipitation accumulations are evaluated againstgauge measurements for June and December 2020 and compared to HYDRO, the PrecipitationEstimation from Remotely Sensed Information using Artificial Neural Networks(PERSIANN) Cloud Classification System (CCS), and the Integrated Multi-satellitE Retrievals for GPM (IMERG). Compared to HYDRO, Hydronn reduces the mean absolute error for hourly accumulations by21 % (22 %) compared to HYDROby 44 % (41 %) for the mean squared error (MSE) and increases the correlationby 138 % (312 %) for June (December) 2020. Compared toIMERG, the improvements correspond to 16 % (14 %),12 % (12 %), and 20 % (56 %),respectively. Furthermore, we show that the probabilistic retrieval is wellcalibrated against gauge measurements when differences in the distributions of the training data and the gauge measurements are accounted for. Hydronn has the potential to significantly improve near-real-time precipitation retrievals over Brazil. Furthermore, our results show that precipitation retrievals based on convolutional neural networks (CNNs) that leverage the full range of available observationsfrom latest-generation geostationary satellites can provide instantaneousprecipitation estimates with accuracy close to that of state-of-the-art PMWretrievals. The high temporal resolution of the geostationary observation allows Hydronn to provide more accurate precipitation accumulations than any of the tested conventional precipitation retrievals. Hydronn thus clearly shows thepotential of deep-learning-based precipitation retrievals to improveprecipitation estimates from currently available satellite imagery.
更多查看译文
关键词
precipitation,near-real-time
AI 理解论文
溯源树
样例
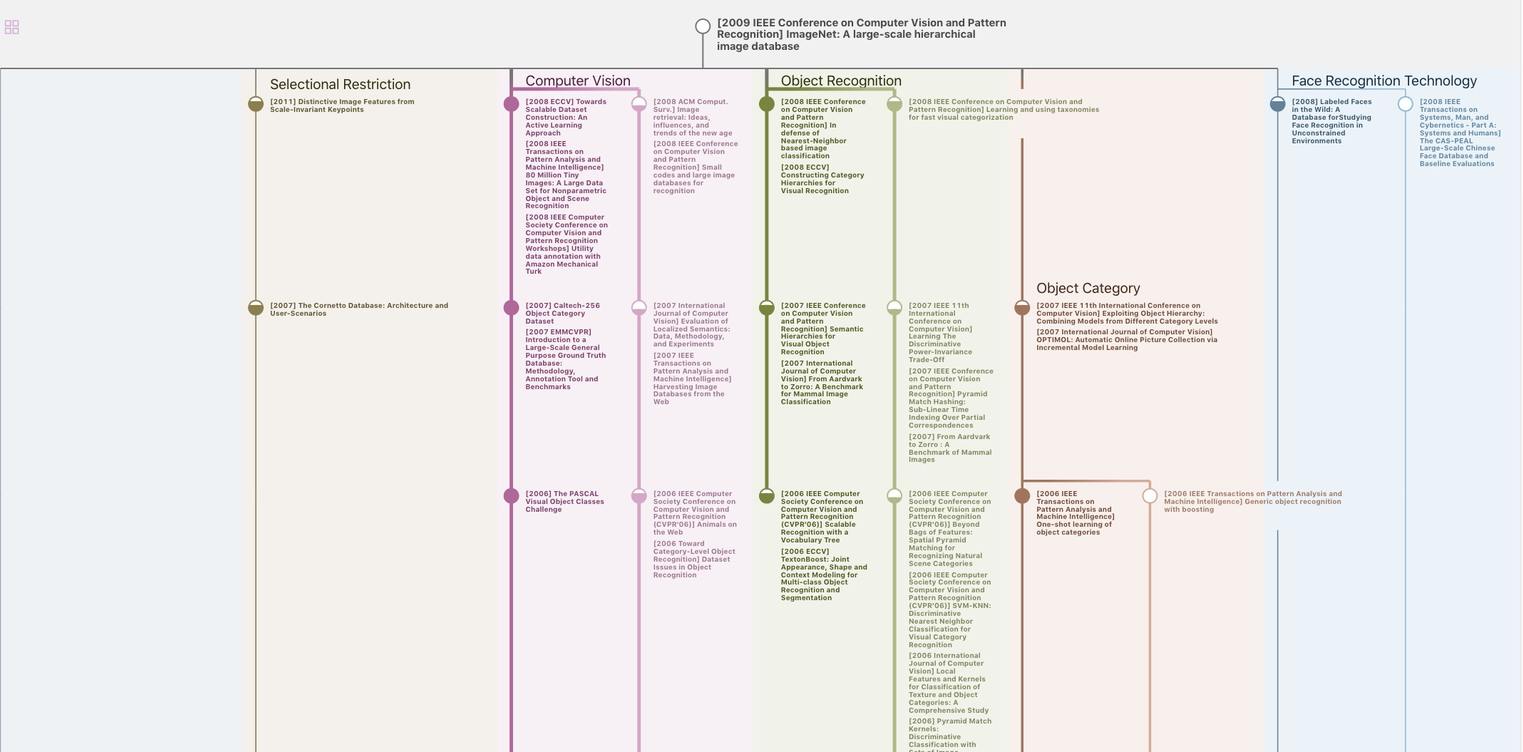
生成溯源树,研究论文发展脉络
Chat Paper
正在生成论文摘要