Solid waste mapping based on very high resolution remote sensing imagery and a novel deep learning approach
GEOCARTO INTERNATIONAL(2023)
摘要
The urbanization worldwide leads to the rapid increase of solid waste, posing a threat to environment and people's wellbeing. However, it is challenging to detect solid waste sites with high accuracy due to complex landscape, and very few studies considered solid waste mapping across multi-cities and in large areas. To tackle this issue, this study proposes a novel deep learning model for solid waste mapping from very high resolution remote sensing imagery. By integrating a multi-scale dilated convolutional neural network (CNN) and a Swin-Transformer, both local and global features are aggregated. Experiments in China, India and Mexico indicate that the proposed model achieves high performance with an average accuracy of 90.62%. The novelty lies in the fusion of CNN and Transformer for solid waste mapping in multi-cities without the need for pixel-wise labelled data. Future work would consider more sophisticated methods such as semantic segmentation for fine-grained solid waste classification.
更多查看译文
关键词
solid waste,remote sensing,deep learning,feature fusion
AI 理解论文
溯源树
样例
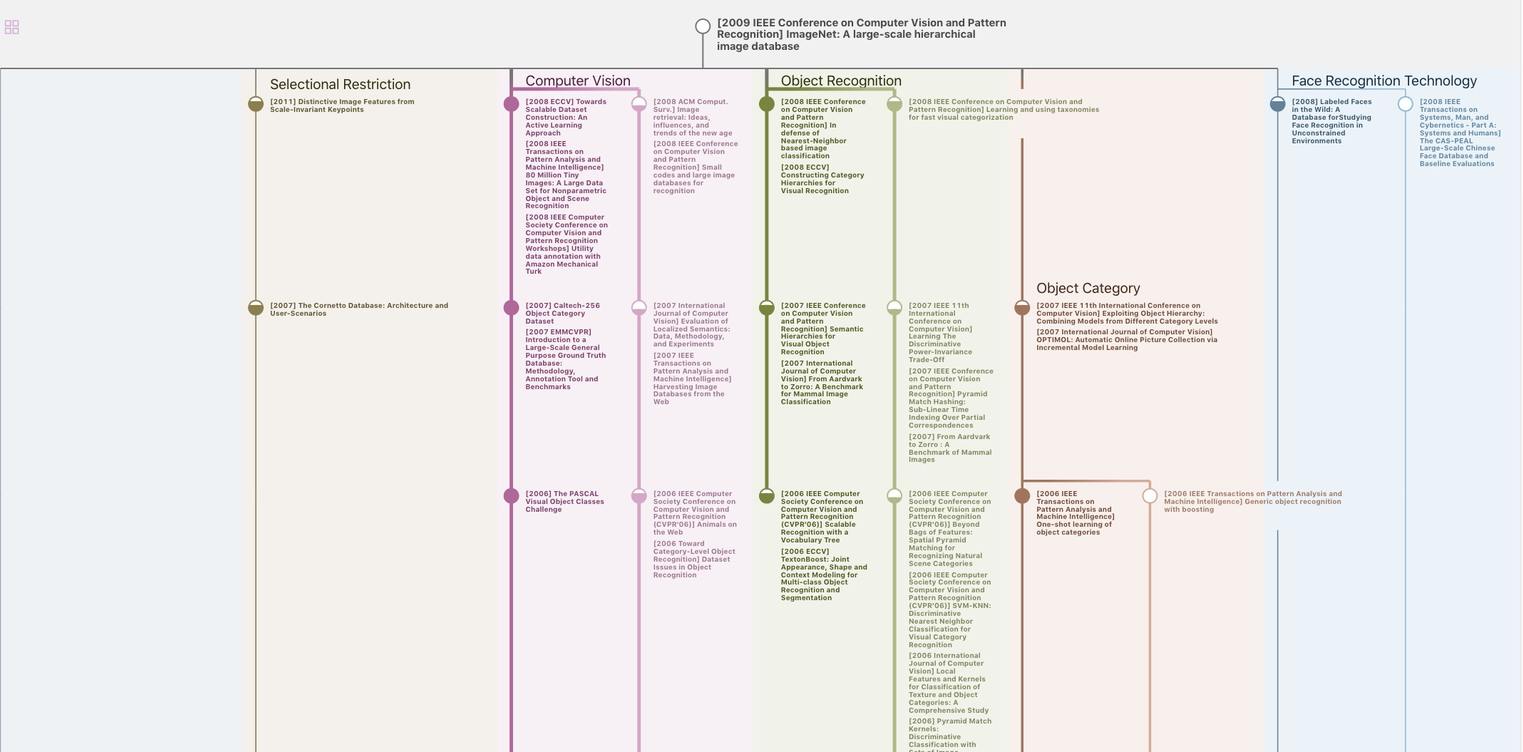
生成溯源树,研究论文发展脉络
Chat Paper
正在生成论文摘要