Recurrent Neural Network-Based Nonlinear Optimization for Braking Control of Electric Vehicles
ENERGIES(2022)
摘要
In this paper, electro-hydraulic braking (EHB) force allocation for electric vehicles (EVs) is modeled as a constrained nonlinear optimization problem (NOP). Recurrent neural networks (RNNs) are advantageous in many folds for solving NOPs, yet existing RNNs' convergence usually requires convexity with calculation of second-order partial derivatives. In this paper, a recurrent neural network-based NOP solver (RNN-NOPS) is developed. It is seen that the RNN-NOPS is designed to drive all state variables to asymptotically converge to the feasible region, with loose requirement on the NOP's first-order partial derivative. In addition, the RNN-NOPS's equilibria are proved to meet Karush-Kuhn-Tucker (KKT) conditions, and the RNN-NOPS behaves with a strong robustness against the violation of the constraints. The comparative studies are conducted to show RNN-NOPS's advantages for solving the EHB force allocation problem, it is reported that the overall regenerative energy of RNN-NOPS is 15.39% more than that of the method for comparison under SC03 cycle.
更多查看译文
关键词
recurrent neural network (RNN),nonlinear optimization problems (NOP),electric vehicle (EV),electro-hydraulic braking (EHB),asymptotical convergence
AI 理解论文
溯源树
样例
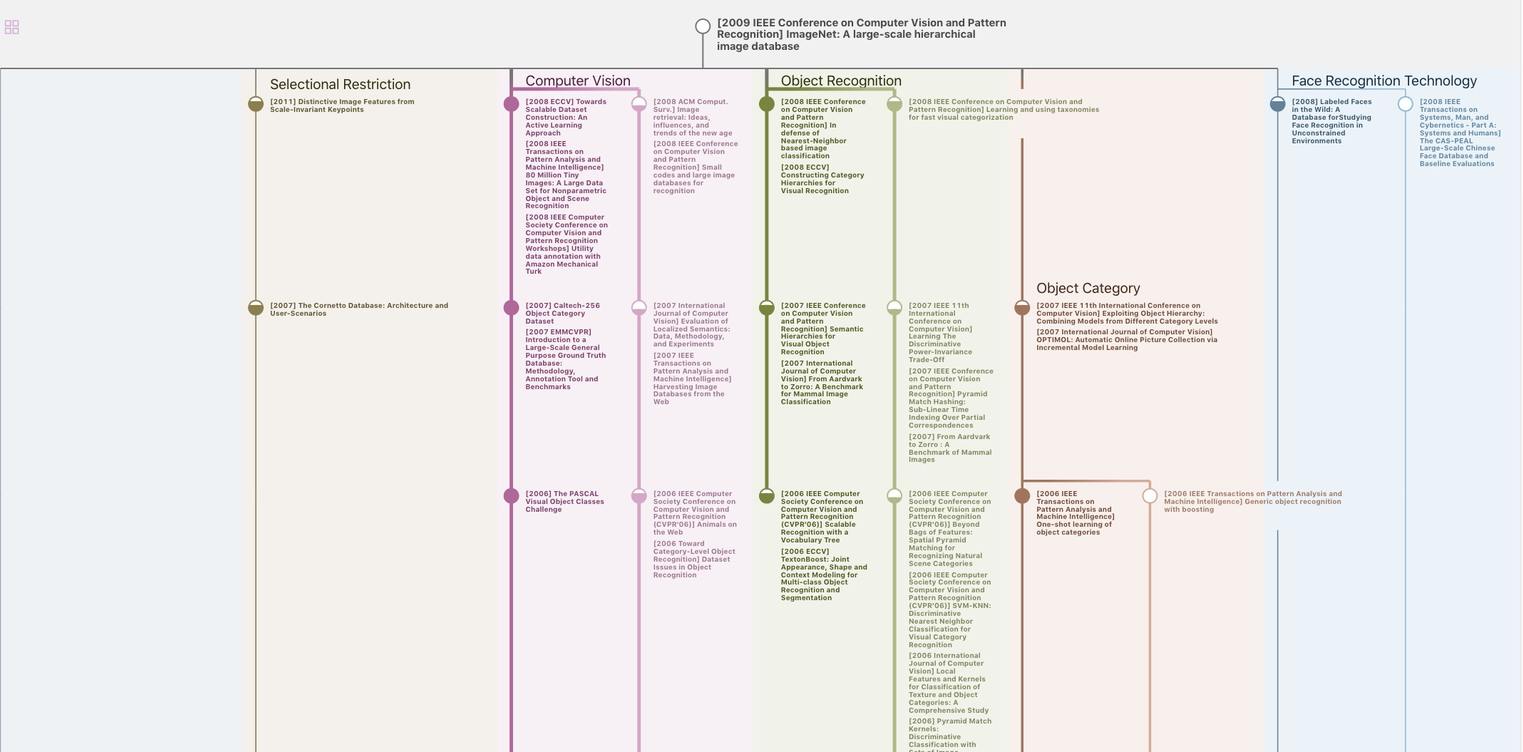
生成溯源树,研究论文发展脉络
Chat Paper
正在生成论文摘要