Total Stem Biomass Estimation Using Sentinel-1 and-2 Data in a Dense Coniferous Forest of Complex Structure and Terrain
FORESTS(2022)
摘要
Accurate above-ground biomass (AGB) estimation across multiple spatial and temporal scales is essential for mitigating climate change and optimizing forest management strategies. The aim of the present study was to investigate the potential of Sentinel optical and Synthetic Aperture Radar (SAR) data in reliably estimating the plot-level total stem biomass (TSB), which constitutes the dominant material among the different tree components of AGB (stem, branches, and leaves). The study area was located in a dense coniferous forest characterized by an uneven-aged structure and intense topography. A random forest (RF) regression analysis was performed to develop TSB predictive models using Sentinel-1 and -2 images in an individual and combined manner. Consequently, three RF models were produced and evaluated for their predictive performance through the k-fold cross-validation (CV) method. The results showcased that the individual use of Sentinel-1 contributed to the production of the most accurate plot-level TSB estimates (i.e., coefficient of determination-R-2 = 0.74, relative mean square error (RMSE) = 1.76 Mg/1000 m(2), mean absolute error (MAE) = 1.48 Mg/1000 m(2)), compared to the use of Sentinel-2 data individually and the Sentinel-1 and -2 combination. In fact, the synergistic use of optical and SAR data led to the generation of an RF model that only marginally underperformed the SAR model (R-2 = 0.73 and R-2 = 0.72, respectively).
更多查看译文
关键词
above-ground biomass,total stem biomass,remote sensing,SAR,Sentinel-1,Sentinel-2,random forest regression
AI 理解论文
溯源树
样例
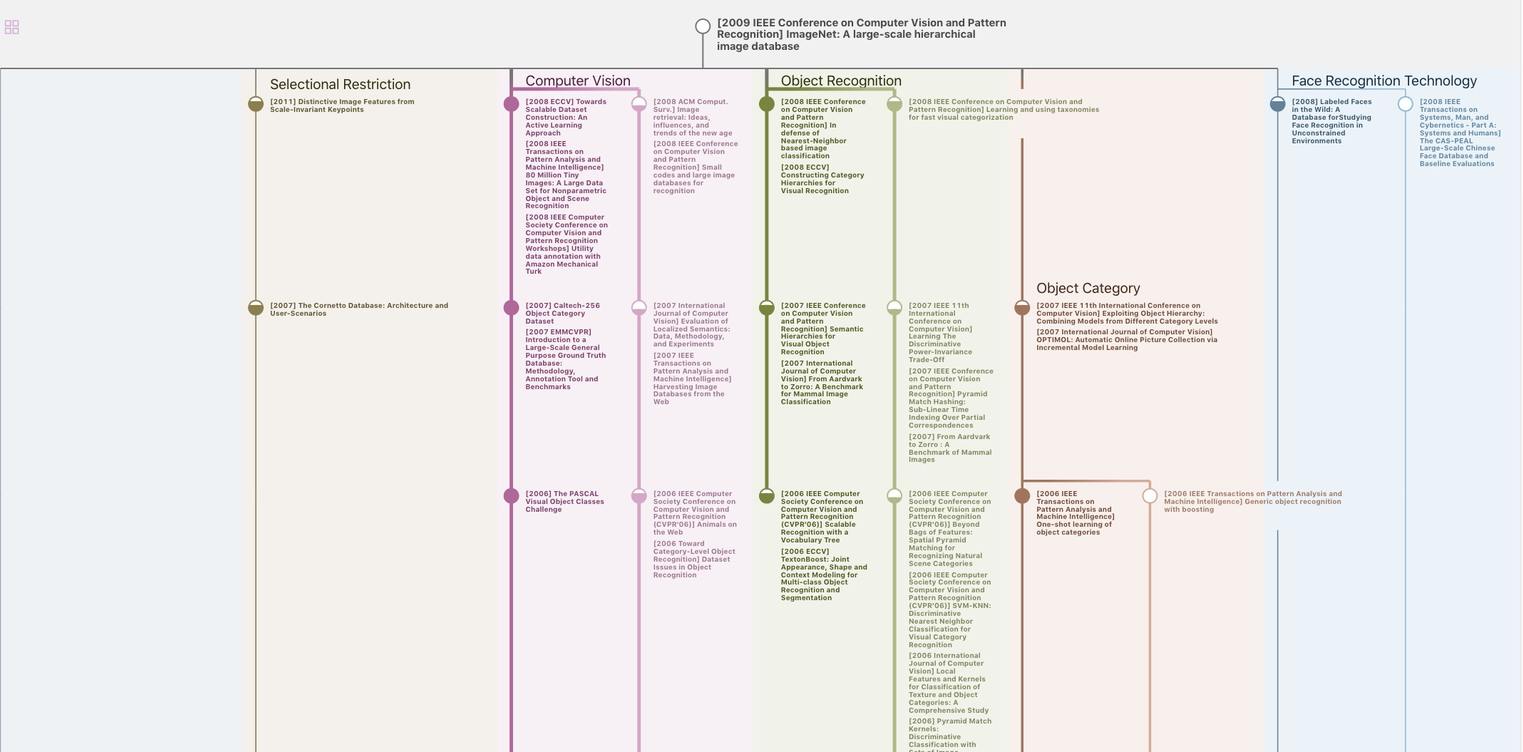
生成溯源树,研究论文发展脉络
Chat Paper
正在生成论文摘要