Multiview nonnegative matrix factorization with dual HSIC constraints for clustering
INTERNATIONAL JOURNAL OF MACHINE LEARNING AND CYBERNETICS(2022)
摘要
To utilize multiple features for clustering, this paper proposes a novel method named as multiview nonnegative matrix factorization with dual HSIC constraints for clustering. The Hilbert-Schmidt independence criterion (HSIC) is employed to measure the correlation(including linear and nonlinear correlation) between the latent representation of each view and the common ones (representation constraint). The independence among the vectors of the basis matrix for each view (basis constraint) is maximized to pursue the discriminant and informative basis. To maintain the nonlinear structure of multiview data, we directly optimize the kernel of the common representation and make its values of the same neighborhood are larger than the others. We adopt partition entropy to constrain the uniformity level of the its values. A novel iterative update algorithm is designed to seek the optimal solutions. We extensively test the proposed algorithm and several state-of-the-art NMF-based multiview methods on four datasets. The clustering results validate the effectiveness of our method.
更多查看译文
关键词
Nonnegative matrix factorization,HSIC,Multiview clustering
AI 理解论文
溯源树
样例
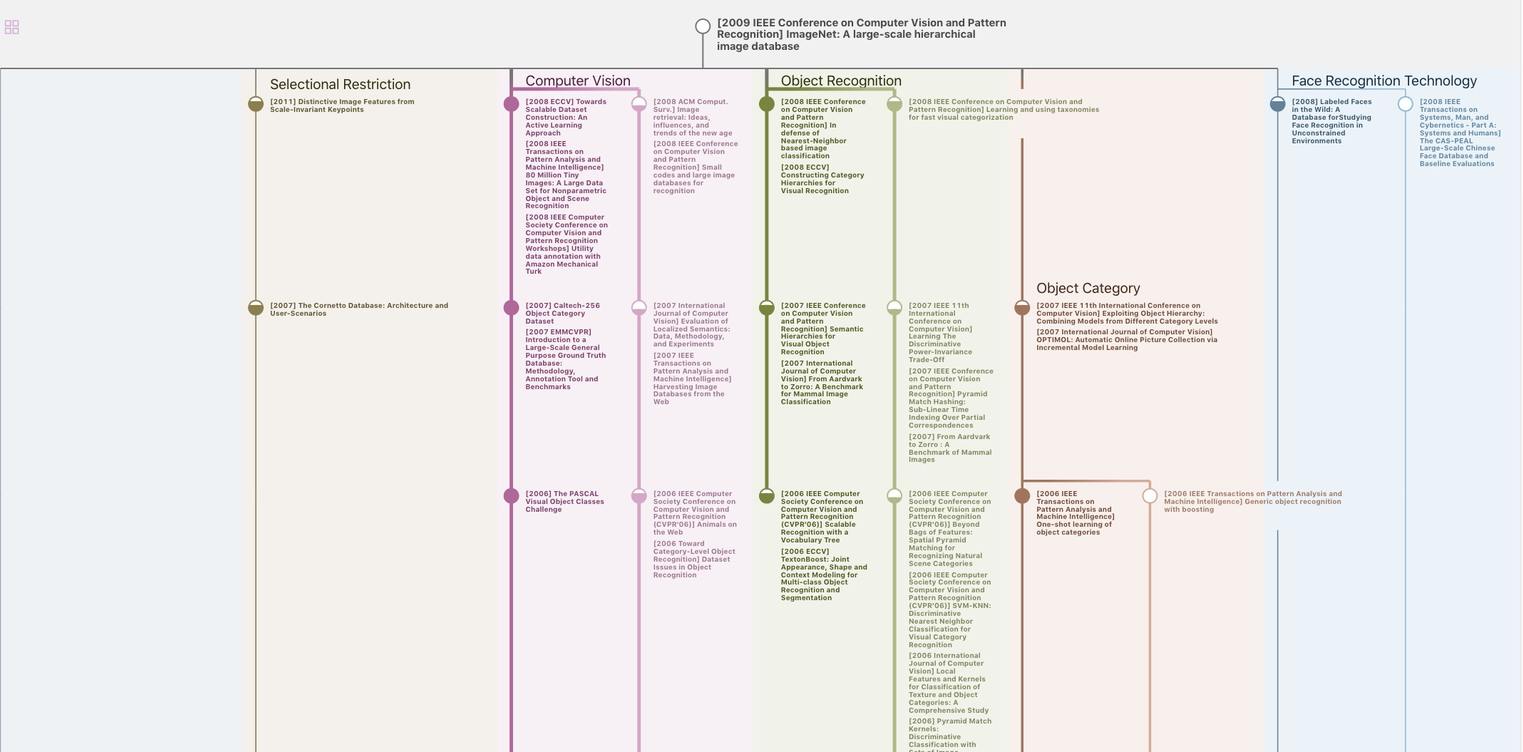
生成溯源树,研究论文发展脉络
Chat Paper
正在生成论文摘要