Performance evaluation of learning models for intrusion detection system using feature selection
JOURNAL OF COMPUTER VIROLOGY AND HACKING TECHNIQUES(2023)
摘要
As computer malware is enlarging with time, the evolution of enhanced intrusion detection systems (IDSs) has become critical. As a result, there is a necessity to create an effective intrusion detection system capable of detecting novel, sophisticated malware. In this work, to enhance the detection capabilities of IDS,effective feature selection techniques namely Chi-Square feature selection and Information Gain feature have been presentedfor extracting the most significant features. A dataset including a wide range of intrusions simulated in a military network environment is used in this work. The Network Intrusion Detection dataset used in the study is available publicly on Kaggle. Initially, the dataset is pre-processed by dropping all the rows that contain any null values and then the standardization of the dataset is performed. Several computational methods consisting of classical machine learning and ensemble learning models have been applied to classify whether there has been an intrusion or not based on the networking stats. The classical machine learning models used in this study are Logistic Regression, K Nearest Neighbour, Naïve Bayes, Decision Tree, and Support Vector Machine. The prominent ensemble learning models like Random Forest, Gradient Boost, Extreme Gradient Boost, and AdaBoost are used for the classification.
更多查看译文
关键词
Intrusion detection,Machine learning,Ensemble learning,Feature selection
AI 理解论文
溯源树
样例
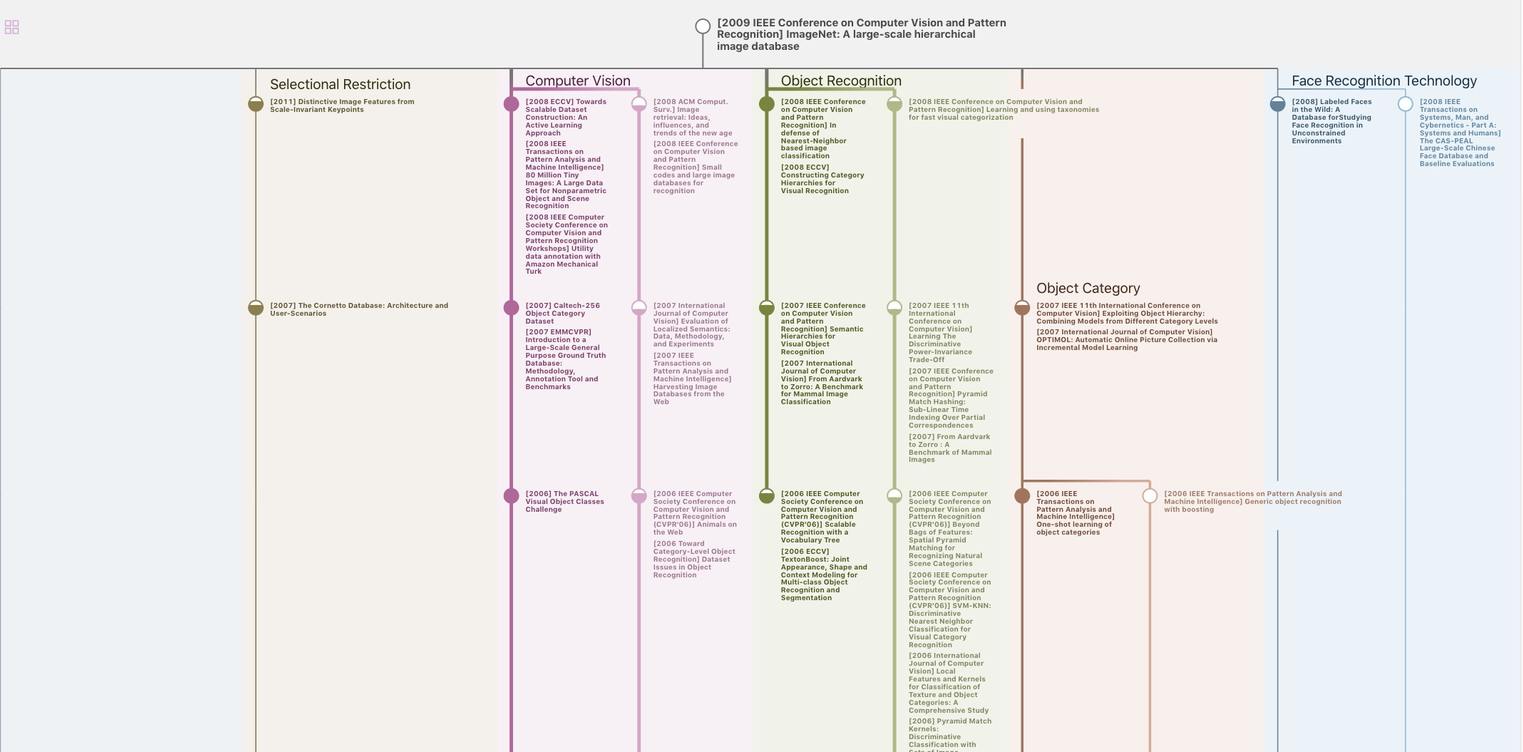
生成溯源树,研究论文发展脉络
Chat Paper
正在生成论文摘要