Fully Automated Placental Volume Quantification From 3D Ultrasound for Prediction of Small-for-Gestational-Age Infants
OBSTETRICAL & GYNECOLOGICAL SURVEY(2022)
摘要
Preeclampsia, fetal growth restriction, and other adverse pregnancy outcomes are significant contributors to perinatal morbidity and mortality. Growing evidence links abnormalities in gross placental development to these outcomes. As such, placental morphometry has recently become a major focus of research. Ultrasound is inexpensive, broadly availability, and image acquisition is easy; therefore, it is the most common imaging modality used to study the placenta. However, 2D imaging has weak associations with adverse perinatal outcomes, whereas 3D ultrasound has allowed investigators insight into the relationship between clinical outcomes and first trimester placental volumes (PVs). However, the currently available techniques are laborious and require manual input. Automated segmentation methods would improve implementation in clinical practice. In this study, a state-of-the-art method for fully automated placenta segmentation and volume measurement is presented, with the overarching goal to create a multivariable prediction model capable of first-trimester identification of those with increased risk for developing fetal growth disorders. This secondary analysis of a prospective cohort of singleton gestations utilized volume data sets for the development of the fully automated segmentation pipeline. The ultrasound data volume sets were obtained at 11 to 14 weeks of gestation, using the max quality setting. The fully automated placenta segmentation pipeline required a ground truth set for the segmentation task and used 99 3D ultrasound manually segmented volume sets to train 3 convolutional neural networks (CNNs) through downsampling and upsampling structures. To evaluate reproducibility of this method, 25 unique subjects were identified for analysis using the CNN pipeline, then the resulting PV measurements were compared with those obtained using the more manual VOCAL tool reported in the primary analysis. Tests demonstrated that the fully automated CNN pipeline achieved a mean Dice similarity coefficient (a measurement of the performance of image segmentation analysis) of 0.8721 +/- 0.0591 on the n = 99 training set. Adjusted models including PV based on CNN (PVCNN) were predictive of SGA < 10%ile (area under the curve [AUC]: PV = 0.780). The addition of PVCNN to a clinical model yielded improvement in AUC (P < 0.05). For predicting SGA < 5%ile, including the PVCNN (0.897) improved performance of the clinical model (0.839, P = 0.015). At 11-14 weeks, PV measurements obtained using this tool were reproducible, significantly associated with the delivery of an SGA infant, and validated when compared with manual segmentation. Limitations of the study include the limited data set used to train during the CNN phase. However, additional evaluation using an independent n = 25 set yielded similar results to the 4-fold cross-validation produced from the n = 99 training set. In addition, all data set images in the study were obtained from a single team, using the same equipment, at one institution. Thus, performance drop is a possibility if this is applied to other equipment and teams. Creation and development of simplified and automated tools for the purposes of volume measurement (and automated placental segmentation) could potentially enable risk stratification earlier in pregnancy, and potentially allow the inclusion of placental biometry in clinical risk assessment. Further advancement of such tools also enables robust assessment of placental surface area and shape. Future development of microvascular Doppler could extend the use of automated placental segmentation tools to also map of the Doppler signal or aid the volumetric quantification. These advances all contribute to the achievable pregnancy clinical management goal of incorporating direct assessment of placental development.
更多查看译文
AI 理解论文
溯源树
样例
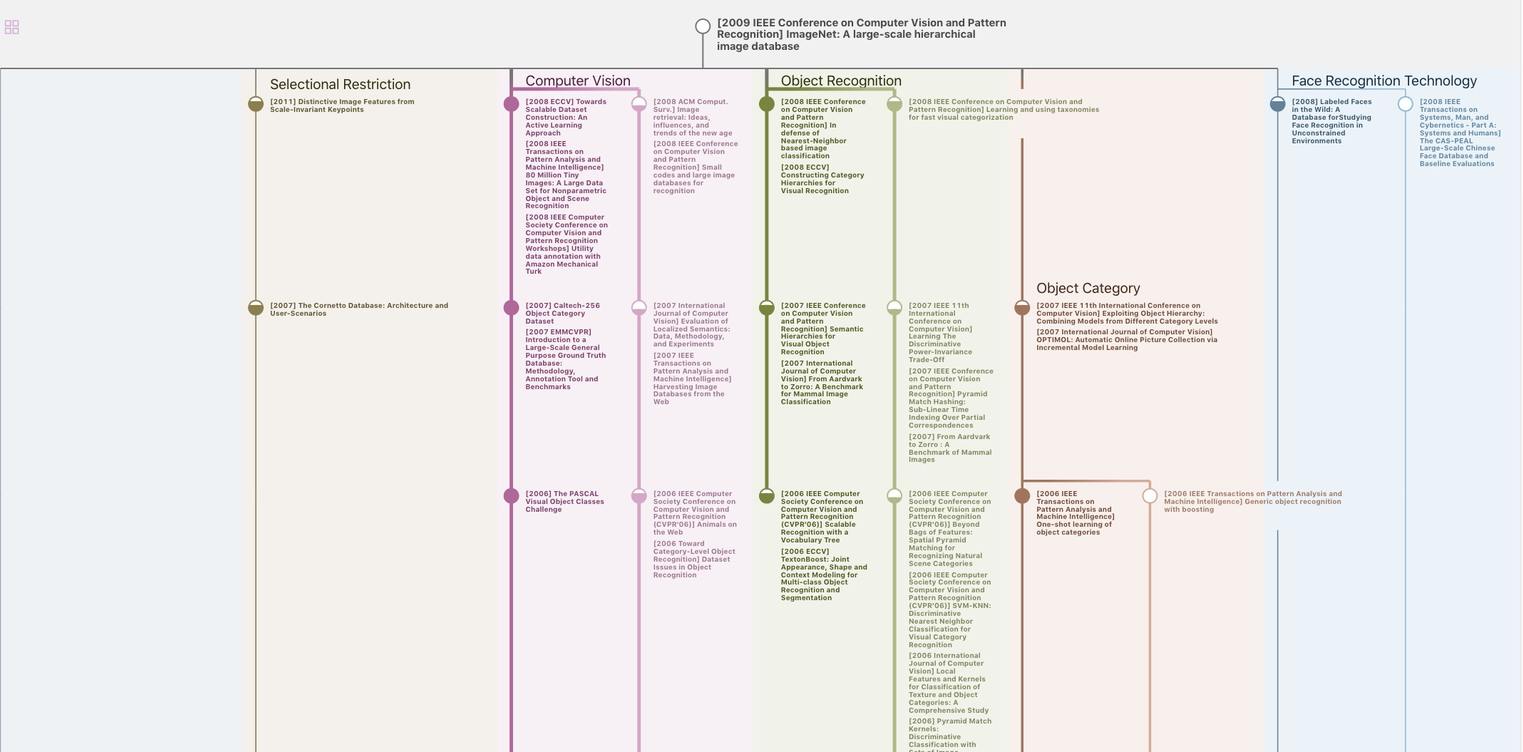
生成溯源树,研究论文发展脉络
Chat Paper
正在生成论文摘要