Active Kriging-Based Adaptive Importance Sampling for Reliability and Sensitivity Analyses of Stator Blade Regulator
CMES-COMPUTER MODELING IN ENGINEERING & SCIENCES(2023)
摘要
The reliability and sensitivity analyses of stator blade regulator usually involve complex characteristics like high-nonlinearity, multi-failure regions, and small failure probability, which brings in unacceptable computing efficiency and accuracy of the current analysis methods. In this case, by fitting the implicit limit state function (LSF) with active Kriging (AK) model and reducing candidate sample pool with adaptive importance sampling (AIS), a novel AK-AIS method is proposed. Herein, the AK model and Markov chain Monte Carlo (MCMC) are first established to identify the most probable failure region(s) (MPFRs), and the adaptive kernel density estimation (AKDE) importance sampling function is constructed to select the candidate samples. With the best samples sequentially attained in the reduced candidate samples and employed to update the Kriging-fitted LSF, the failure probability and sensitivity indices are acquired at a lower cost. The proposed method is verified by two multi-failure numerical examples, and then applied to the reliability and sensitivity analyses of a typical stator blade regulator. With methods comparison, the proposed AK-AIS is proven to hold the computing advantages on accuracy and efficiency in complex reliability and sensitivity analysis problems.
更多查看译文
关键词
Markov chain Monte Carlo,active Kriging,adaptive kernel density estimation,importance sampling
AI 理解论文
溯源树
样例
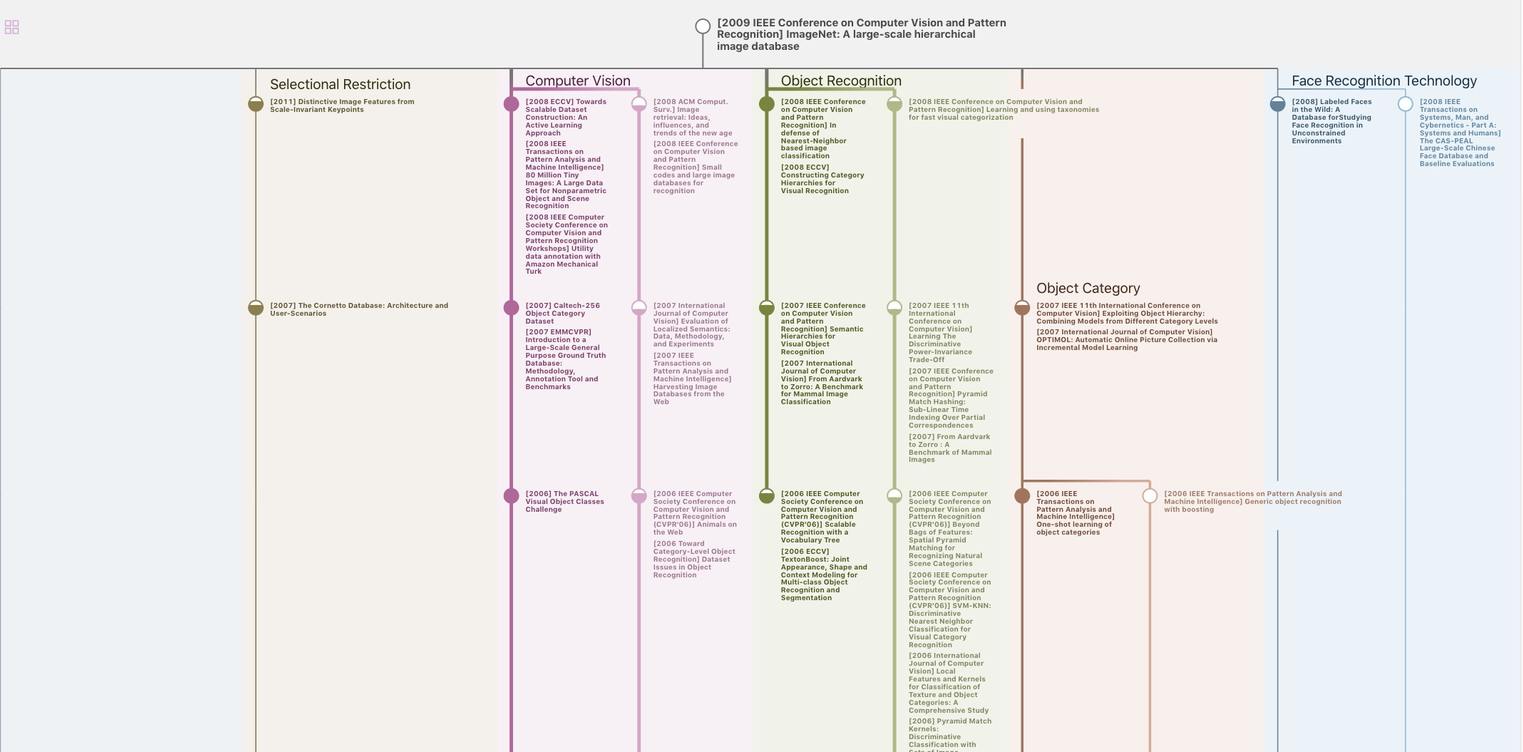
生成溯源树,研究论文发展脉络
Chat Paper
正在生成论文摘要