Statistical Depth for Text Data: An Application to the Classification of Healthcare Data
MATHEMATICS(2023)
摘要
This manuscript introduces a new concept of statistical depth function: the compositional D-depth. It is the first data depth developed exclusively for text data, in particular, for those data vectorized according to a frequency-based criterion, such as the tf-idf (term frequency-inverse document frequency) statistic, which results in most vector entries taking a value of zero. The proposed data depth consists of considering the inverse discrete Fourier transform of the vectorized text fragments and then applying a statistical depth for functional data, D. This depth is intended to address the problem of sparsity of numerical features resulting from the transformation of qualitative text data into quantitative data, which is a common procedure in most natural language processing frameworks. Indeed, this sparsity hinders the use of traditional statistical depths and machine learning techniques for classification purposes. In order to demonstrate the potential value of this new proposal, it is applied to a real-world case study which involves mapping Consolidated Framework for Implementation and Research (CFIR) constructs to qualitative healthcare data. It is shown that the DDG-classifier yields competitive results and outperforms all studied traditional machine learning techniques (logistic regression with LASSO regularization, artificial neural networks, decision trees, and support vector machines) when used in combination with the newly defined compositional D-depth.
更多查看译文
关键词
compositional depth,multivariate data,natural language processing,qualitative data,statistical depth,supervised classification,text mining
AI 理解论文
溯源树
样例
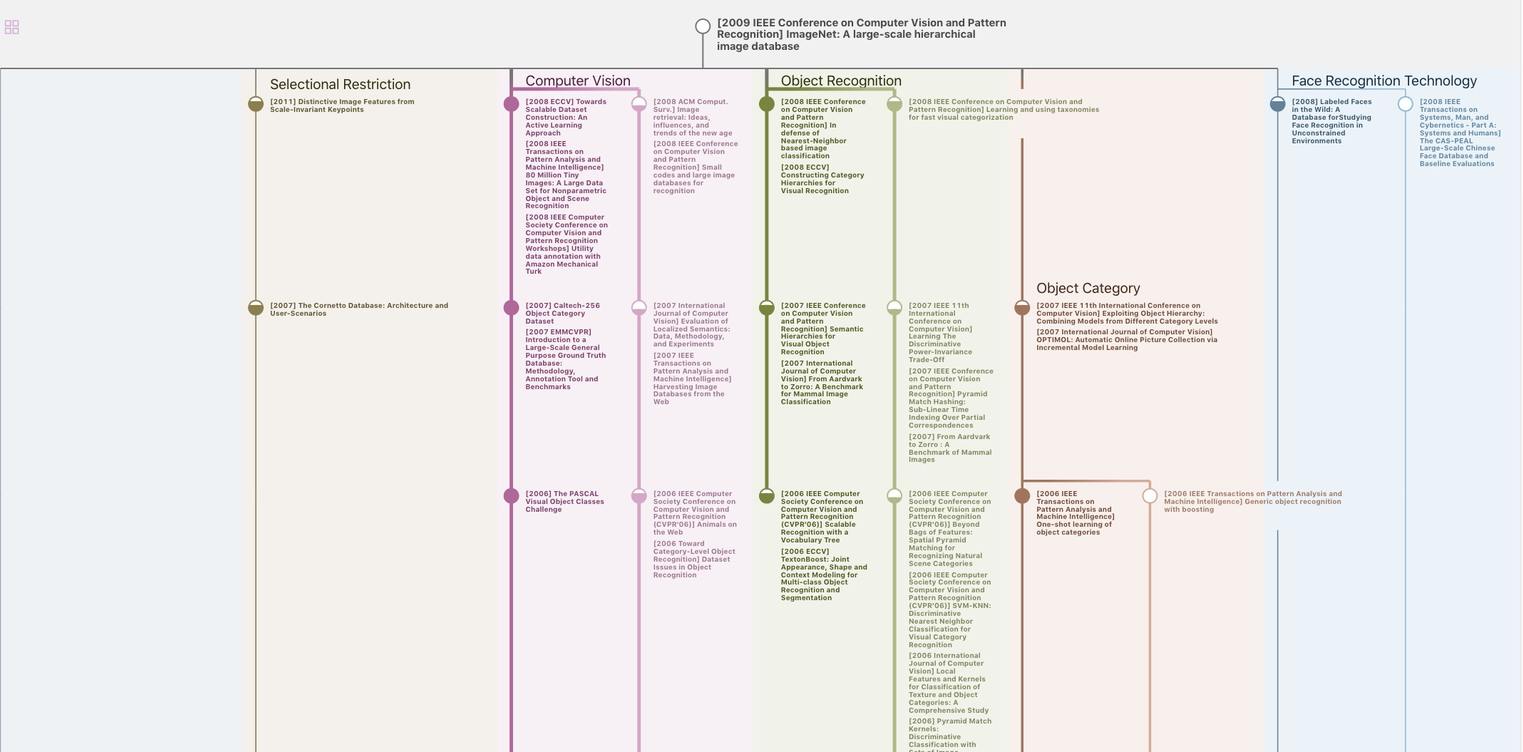
生成溯源树,研究论文发展脉络
Chat Paper
正在生成论文摘要