Unsupervised structural damage detection based on an improved generative adversarial network and cloud model
JOURNAL OF LOW FREQUENCY NOISE VIBRATION AND ACTIVE CONTROL(2023)
摘要
The measurements derived from damaged conditions are difficult to acquire in actual structures, which limits the applicability of supervised damage detection methods. In this study, an unsupervised damage detection method that leverages an improved generative adversarial network (IGAN) and cloud model (CM) is proposed. This method only needs the data in the healthy state of the structure for model training, which can solve the above problems. Firstly, an IGAN model is established, which uses the encoder-decoder-encoder generative network to encode, reconstruct and re-encode the structural response in healthy state. The essential features hidden in complex data are learned by minimizing the distance between the real response and the reconstructed response, and the distance between the latent vectors obtained after two different encodings. During the test phase, when unknown state data is input into the model, the differences of the latent features of the two different codes can be used to preliminarily judge whether the structure is damaged. In addition, CM theory is used to further quantify structural damage and solve the problem of damage misjudgment caused by measurement noise, model parameters, feature selection, and other uncertain factors. The effectiveness of the proposed damage detection method is verified by Phase I IASC-ASCE benchmark structure and a bridge health monitoring benchmark model. The results prove that the proposed method can accurately detect the damage that has occurred.
更多查看译文
关键词
Structural health monitoring,damage detection,unsupervised learning,generative adversarial network,auto-encoder,cloud model
AI 理解论文
溯源树
样例
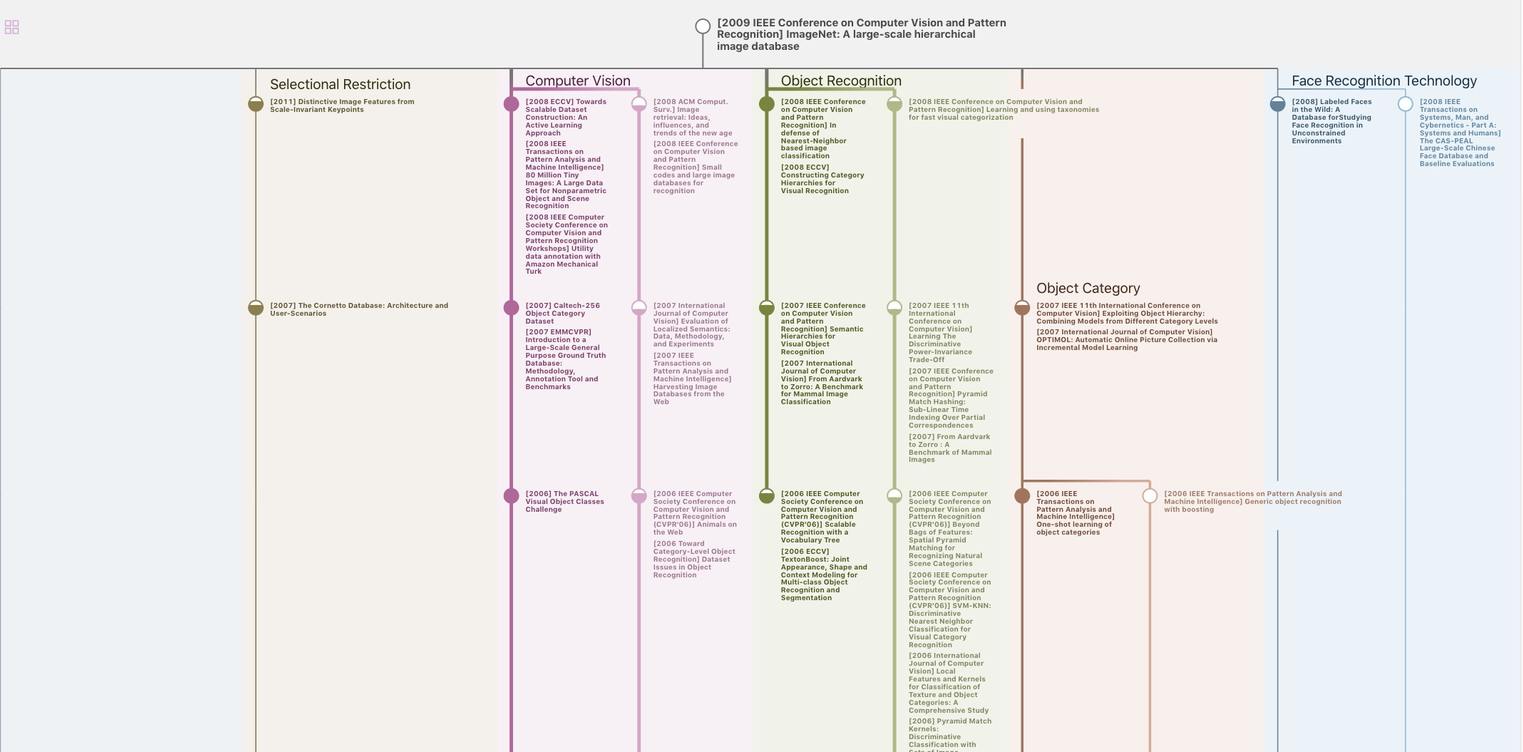
生成溯源树,研究论文发展脉络
Chat Paper
正在生成论文摘要