Face Gender and Age Classification Based on Multi-Task, Multi-Instance and Multi-Scale Learning
APPLIED SCIENCES-BASEL(2022)
摘要
Featured Application Facial recognition. Automated facial gender and age classification has remained a challenge because of the high inter-subject and intra-subject variations. We addressed this challenging problem by studying multi-instance- and multi-scale-enhanced multi-task random forest architecture. Different from the conventional single facial attribute recognition method, we designed effective multi-task architecture to learn gender and age simultaneously and used the dependency between gender and age to improve its recognition accuracy. In the study, we found that face gender has a great influence on face age grouping; thus, we proposed a random forest face age grouping method based on face gender conditions. Specifically, we first extracted robust multi-instance and multi-scale features to reduce the influence of various intra-subject distortion types, such as low image resolution, illumination and occlusion, etc. Furthermore, we used a random forest classifier to recognize facial gender. Finally, a gender conditional random forest was proposed for age grouping to address inter-subject variations. Experiments were conducted by using two popular MORPH-II and Adience datasets. The experimental results showed that the gender and age recognition rates in our method can reach 99.6% and 96.14% in the MORPH-II database and 93.48% and 63.72% in the Adience database, reaching the state-of-the-art level.
更多查看译文
关键词
facial attribute recognition,feature extraction,deep learning,random forest
AI 理解论文
溯源树
样例
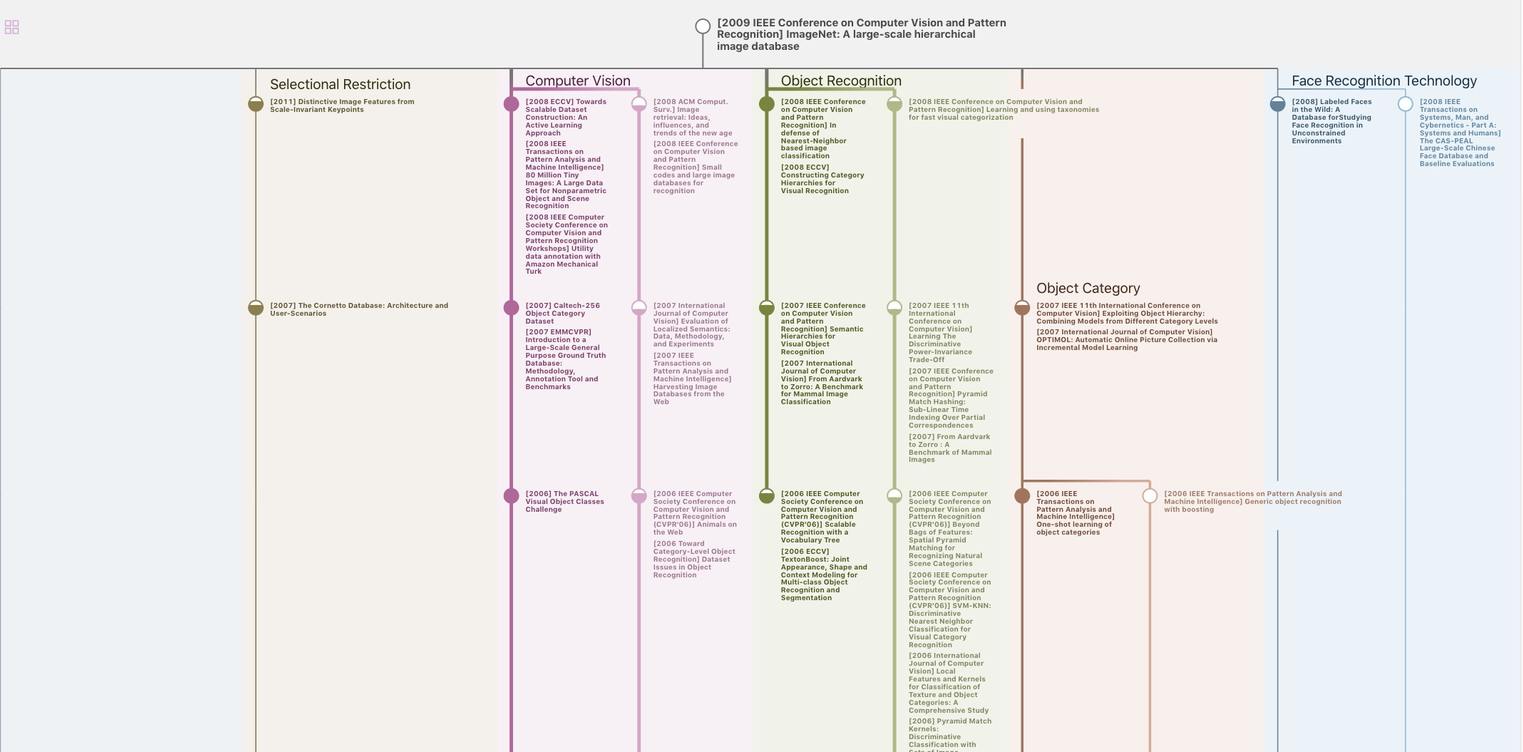
生成溯源树,研究论文发展脉络
Chat Paper
正在生成论文摘要