Mixed-Sized Biomedical Image Segmentation Based on U-Net Architectures
APPLIED SCIENCES-BASEL(2023)
摘要
Convolutional neural networks (CNNs) are becoming increasingly popular in medical Image Segmentation. Among them, U-Net is a widely used model that can lead to cutting-edge results for 2D biomedical Image Segmentation. However, U-Net performance can be influenced by many factors, such as the size of the training dataset, the performance metrics used, the quality of the images and, in particular, the shape and size of the organ to be segmented. This could entail a loss of robustness of the U-Net-based models. In this paper, the performance of the considered networks is determined by using the publicly available images from the 3D-IRCADb-01 dataset. Different organs with different features are considered. Experimental results show that the U-Net-based segmentation performance decreases when organs with sparse binary masks are considered. The solution proposed in this paper, based on automated zooming of the parts of interest, allows improving the performance of the segmentation model by up to 20% in terms of Dice coefficient metric, when very sparse segmentation images are used, without affecting the cost of the learning process.
更多查看译文
关键词
Image Segmentation,convolutional neural networks,biomedical image analysis
AI 理解论文
溯源树
样例
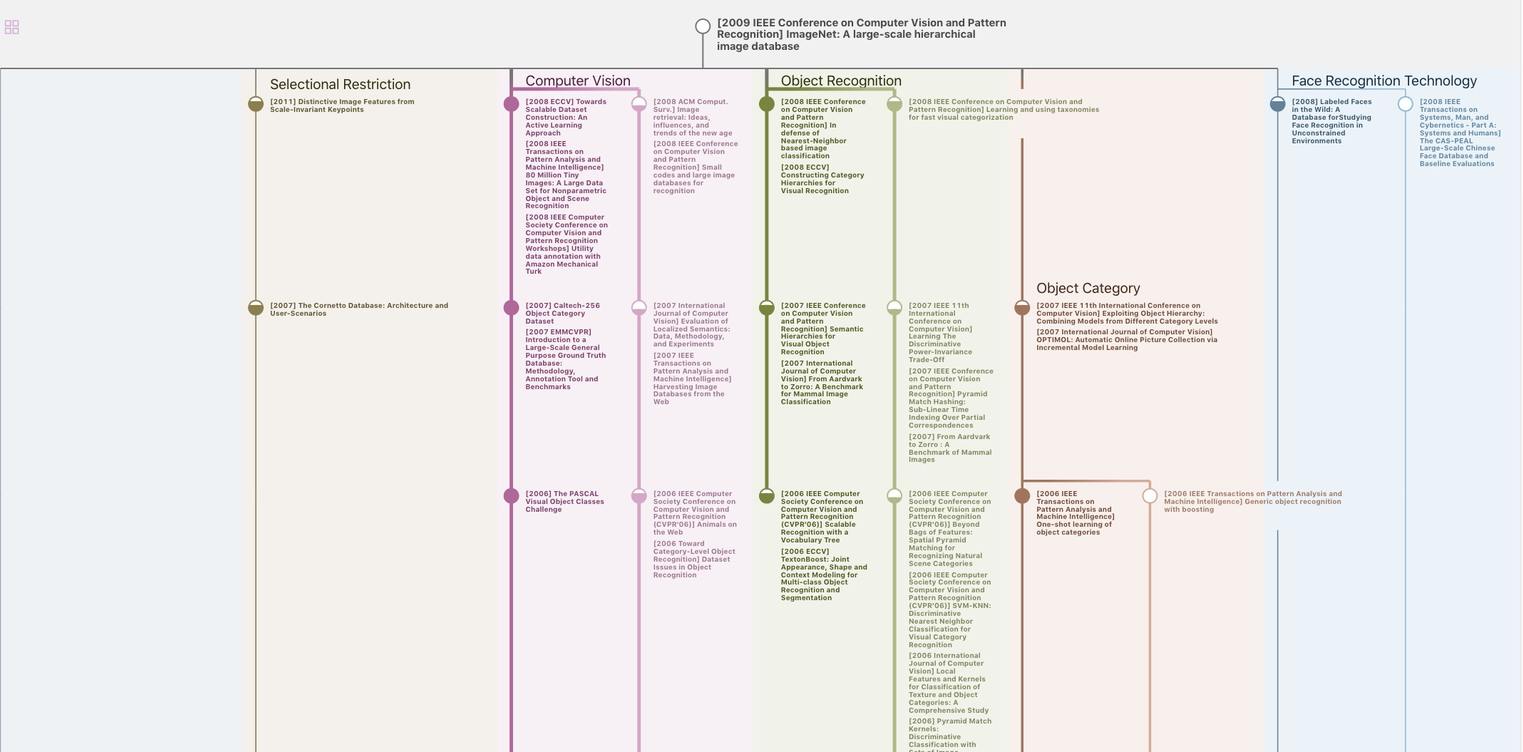
生成溯源树,研究论文发展脉络
Chat Paper
正在生成论文摘要