Machine learning–assisted internal standard calibration label-free SERS strategy for colon cancer detection
Analytical and bioanalytical chemistry(2023)
摘要
Liquid biopsies have significance for early colon cancer screening and improving patient survival. Recently, several researchers have applied surface-enhanced Raman spectroscopy (SERS) for the label-free and non-invasive detection of serum. Most of these studies performed the assay using a mixture of noble metal nanoparticles (NMNPs) with serum. However, SERS analysis of serum remains a challenge in terms of reproducibility and stability, as NMNPs tend to aggregate when mixed with serum, resulting in a non-uniform distribution of hot spots. Here, we report on the non-invasive identification of colon cancer (CC) using an internal standard (IS)–calibrated label-free serum SERS assay in combination with machine learning. Serum SERS spectra of 50 CC patients and 50 health volunteers have been obtained using silver nanoparticle (Ag NP) colloid and mercaptopropionic acid–modified Ag NPs (Ag NPs–MPA) as the SERS substrates. Decision tree (DT), random forest (RF), and principal component and linear discriminant analysis (PCA–LDA) algorithms were utilized to establish the diagnosis model for SERS spectra data classifying. The results show that the RF model provides a high diagnostic accuracy compared to PCA–LDA. Following calibration with IS molecules, high diagnostic accuracy of over 90% and 100% specificity can be achieved with DT, RF, and PCA–LDA algorithms to differentiate between cancer and normal groups. The results from this exploratory work demonstrate that serum SERS detection combined with multivariate statistical methods and IS calibration has great potential for the non-invasive and label-free detection of CC.
更多查看译文
关键词
Colon cancer,Internal standard calibration,Label-free,Machine learning,SERS
AI 理解论文
溯源树
样例
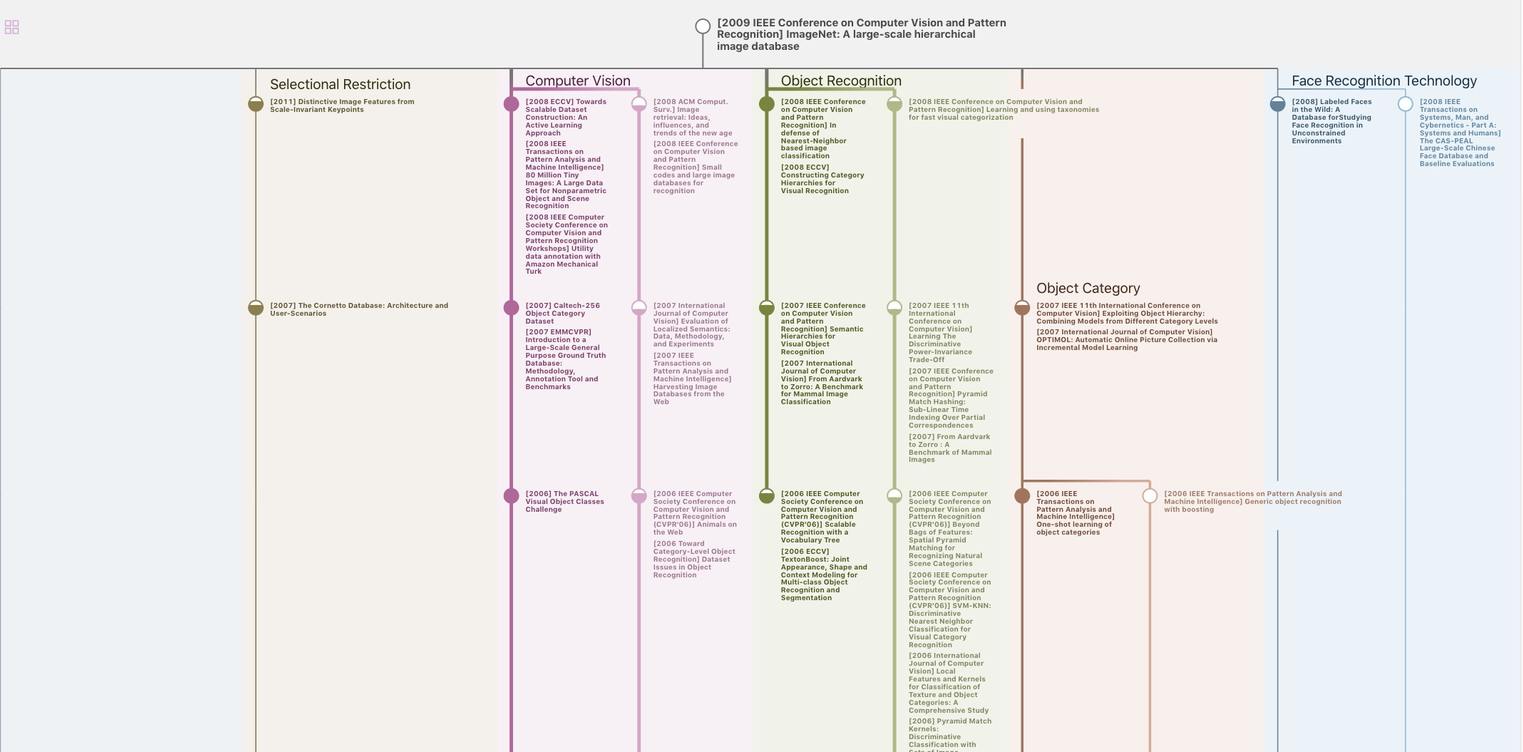
生成溯源树,研究论文发展脉络
Chat Paper
正在生成论文摘要