Development of a compressed FCN architecture for semantic segmentation using Particle Swarm Optimization
Neural computing & applications(2023)
摘要
Researchers have adapted the conventional deep learning classification networks to generate Fully Conventional Networks (FCN) for carrying out accurate semantic segmentation. However, such models are expensive both in terms of storage and inference time and not readily employable on edge devices. In this paper, a compressed version of VGG16-based Fully Convolution Network (FCN) has been developed using Particle Swarm Optimization. It has been shown that the developed model can offer tremendous saving in storage space and also faster inference time, and can be implemented on edge devices. The efficacy of the proposed approach has been tested using potato late blight leaf images from publicly available PlantVillage dataset, street scene image dataset and lungs X-Ray dataset and it has been shown that it approaches the accuracies offered by standard FCN even after 851× compression.
更多查看译文
关键词
FCN architecture,Semantic segmentation,Particle Swarm Optimization,Optimization,Compression and acceleration,Disease segmentation
AI 理解论文
溯源树
样例
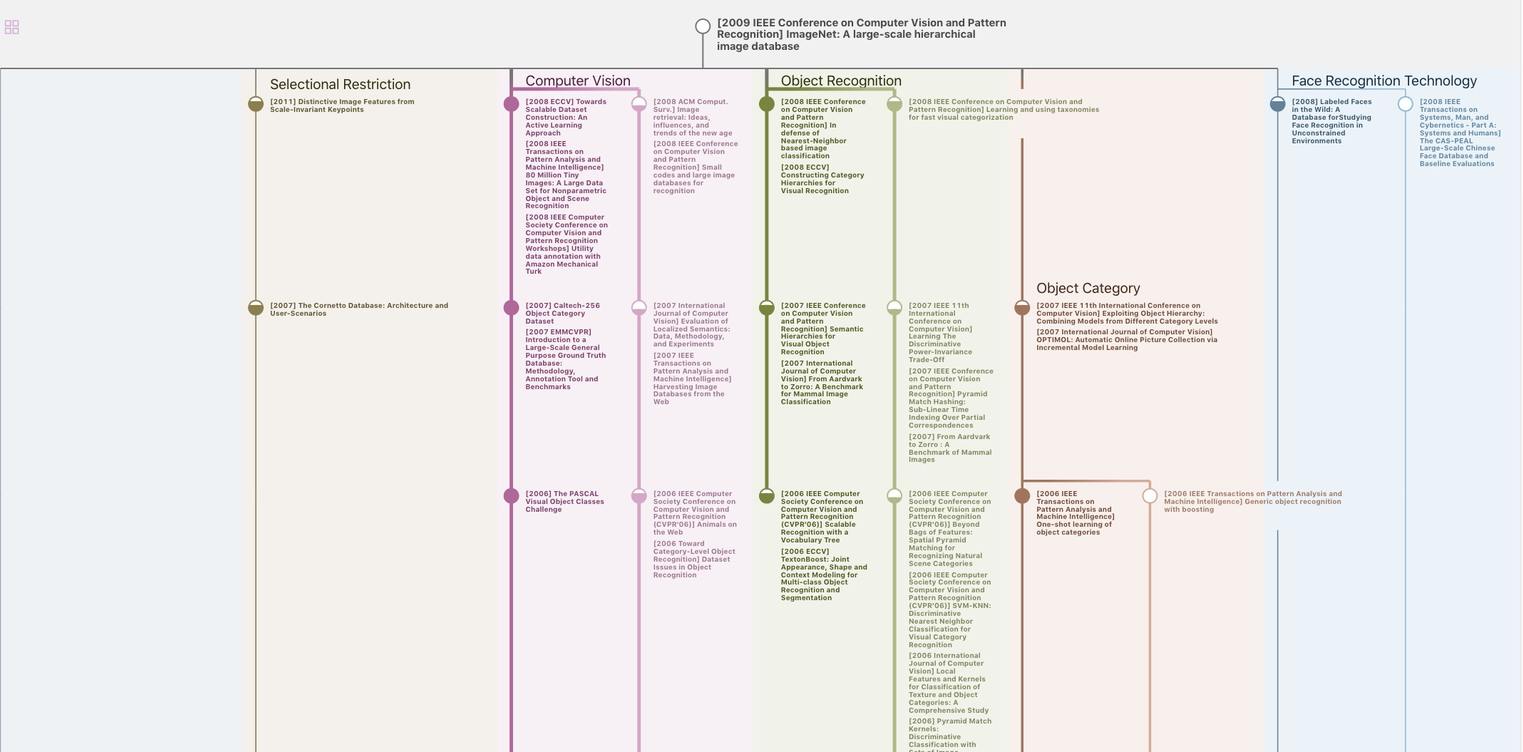
生成溯源树,研究论文发展脉络
Chat Paper
正在生成论文摘要