Detecting and quantifying spatial misalignment between longitudinal kilovoltage computed tomography (kVCT) scans of the head and neck by using convolutional neural networks (CNNs)
Technology and health care : official journal of the European Society for Engineering and Medicine(2023)
摘要
BACKGROUND: Adaptive radiotherapy (ART) aims to address anatomical modifications appearing during the treatment of patients by modifying the planning treatment according to the daily positioning image. Clinical implementation of ART relies on the quality of the deformable image registration (DIR) algorithms included in the ART workflow. To translate ART into clinical practice, automatic DIR assessment is needed. OBJECTIVE: This article aims to estimate spatial misalignment between two head and neck kilovoltage computed tomography (kVCT) images by using two convolutional neural networks (CNNs). METHODS: The first CNN quantifies misalignments between 0 mm and 15 mm and the second CNN detects and classifies misalignments into two classes (poor alignment and good alignment). Both networks take pairs of patches of 33x33x33 mm(3) as inputs and use only the image intensity information. The training dataset was built by deforming kVCT images with basis splines (B-splines) to simulate DIR error maps. The test dataset was built using 2500 landmarks, consisting of hard and soft landmark tissues annotated by 6 clinicians at 10 locations. RESULTS: The quantification CNN reaches a mean error of 1.26 mm ( +/- 1.75 mm) on the landmark set which, depending on the location, has annotation errors between 1 mm and 2 mm. The errors obtained for the quantification network fit the computed interoperator error. The classification network achieves an overall accuracy of 79.32%, and although the classification network overdetects poor alignments, it performs well (i.e., it achieves a rate of 90.4%) in detecting poor alignments when given one. CONCLUSION: The performances of the networks indicate the feasibility of using CNNs for an agnostic and generic approach to misalignment quantification and detection.
更多查看译文
关键词
DIR in radiotherapy,DIR validation,Registration error,adaptive radiotherapy,convolutional neural networks,deep learning,supervised learning
AI 理解论文
溯源树
样例
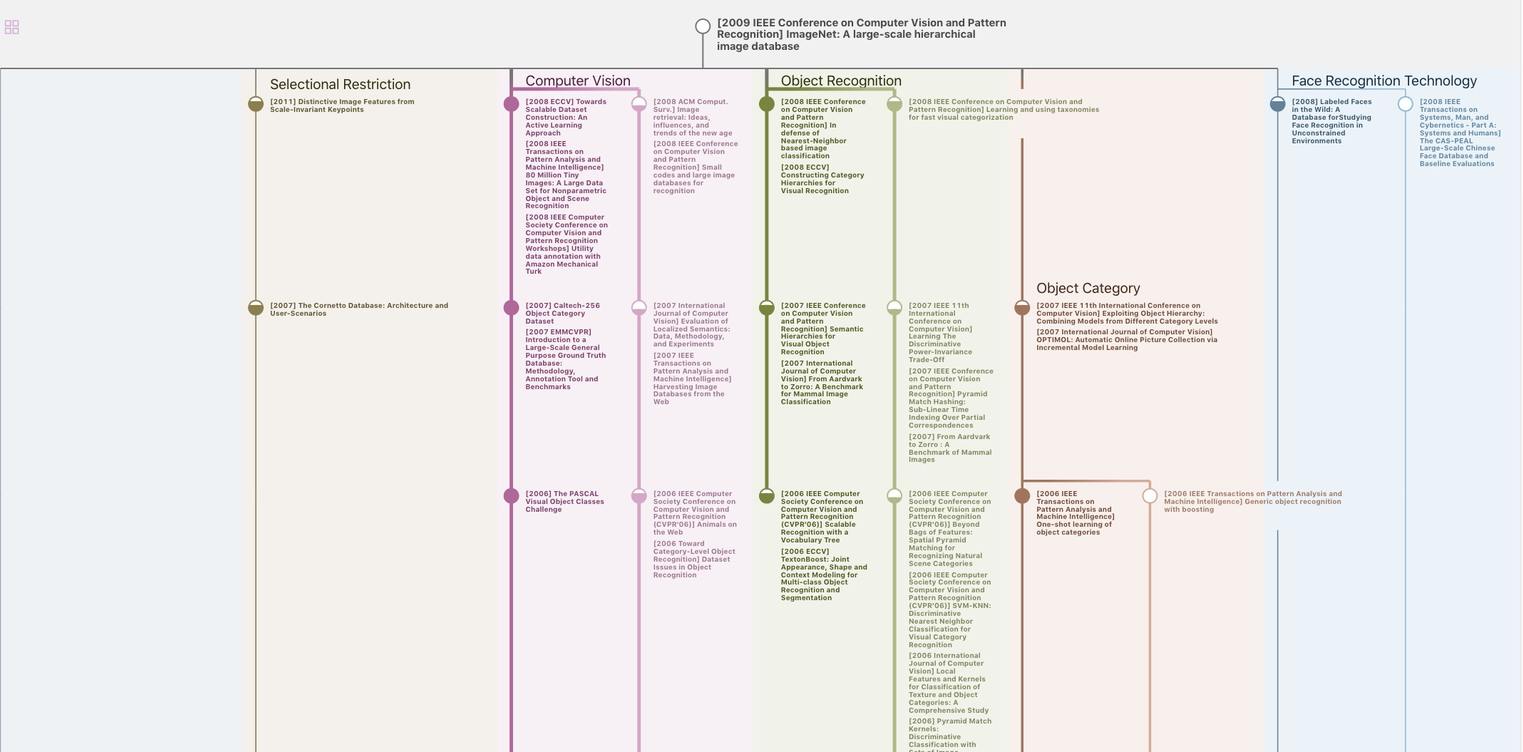
生成溯源树,研究论文发展脉络
Chat Paper
正在生成论文摘要