Two-stage anomaly detection for positive samples and small samples based on generative adversarial networks
MULTIMEDIA TOOLS AND APPLICATIONS(2023)
摘要
Anomaly detection approaches based on generative adversary networks usually directly input the image into the generator for reconstruction. As a result, the results of anomaly detection are not ideal. This paper proposes a novel anomaly detection model based on a two-stage generative adversarial network to improve the results. It consists of feature extraction and anomaly detection networks. The former combines a convolutional neural network and multi-scale feature extraction to study latent code. The latent code from the former model instead of the original image is fed to the generator of the anomaly detection module. The experiment shows the proposed method outperforms several existing anomaly detection methods with multiple datasets. Additionally, the quantitative result indicates the proposed model optimizes anomaly detection performance and improves by 8.8 % and 19.2 % on both the liver CT image medical dataset and the CIFAR10 public dataset respectively compared to the baseline of the skip-GANomaly model.
更多查看译文
关键词
Anomaly detection,Small samples,Positive samples,Generative adversarial networks
AI 理解论文
溯源树
样例
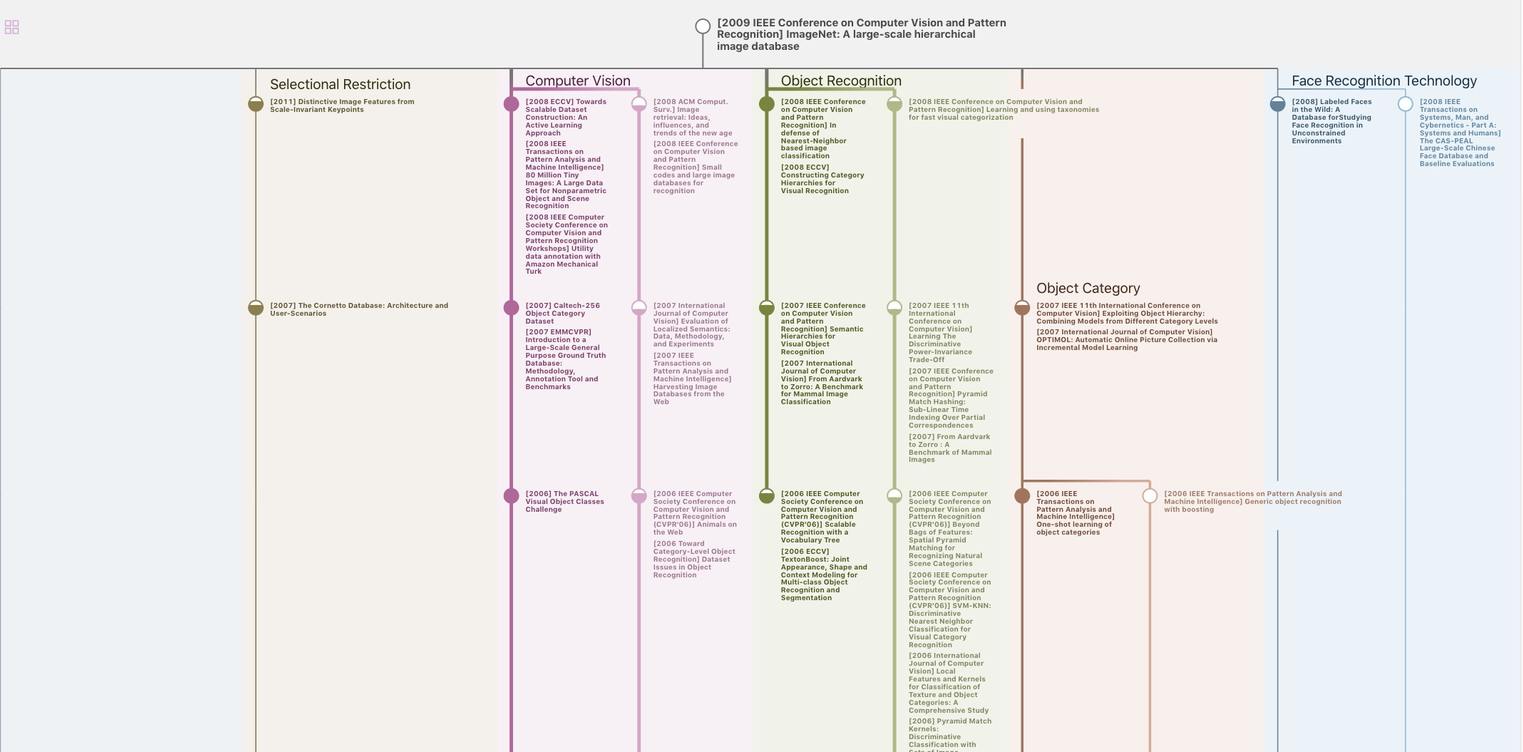
生成溯源树,研究论文发展脉络
Chat Paper
正在生成论文摘要