Personalized federated learning with model interpolation among client clusters and its application in smart home
WORLD WIDE WEB-INTERNET AND WEB INFORMATION SYSTEMS(2023)
摘要
The proliferation of high-performance personal devices and the widespread deployment of machine learning (ML) applications have led to two consequences: the volume of private data from individuals or groups has exploded over the past few years; and the traditional central servers for training ML models have experienced communication and performance bottlenecks in the face of massive amounts of data. However, this reality also provides the possibility of keeping data local for ML training and fusing models on a broader scale. As a new branch of ML application, Federated Learning (FL) aims to solve the problem of multi-party joint learning on the premise of protecting personal data privacy. However, due to the heterogeneity of devices, including network connection, network bandwidth, computing resources, etc., it is unrealistic to train, update and aggregate models in all devices in parallel, while personal data is often not independent and identically distributed (Non-IID) due to multiple reasons. This reality poses a challenge to the speed and convergence of FL. In this paper, we propose the pFedCAM algorithm, which aims to improve the robustness of the FL system to device heterogeneity and Non-IID data, while achieving some degree of federation model personalization. pFedCAM is based on the idea of clustering and model interpolation by classifying heterogeneous clients and performing FedAvg algorithm in parallel, and then combining them into personalized federated global models by inter-cluster model interpolation. Experiments show that the accuracy of pFedCAM improves 10.3% on Fashion-MNIST and 11.3% on CIFAR-10 compared to the benchmark in the case of Non-IID data. In the end, we applied pFedCAM in HomeProtect, a smart home privacy protection framework we designed, and achieved good practical results in the case of flame recognition.
更多查看译文
关键词
Federated Learning,Personalization,Clustering,Model interpolation
AI 理解论文
溯源树
样例
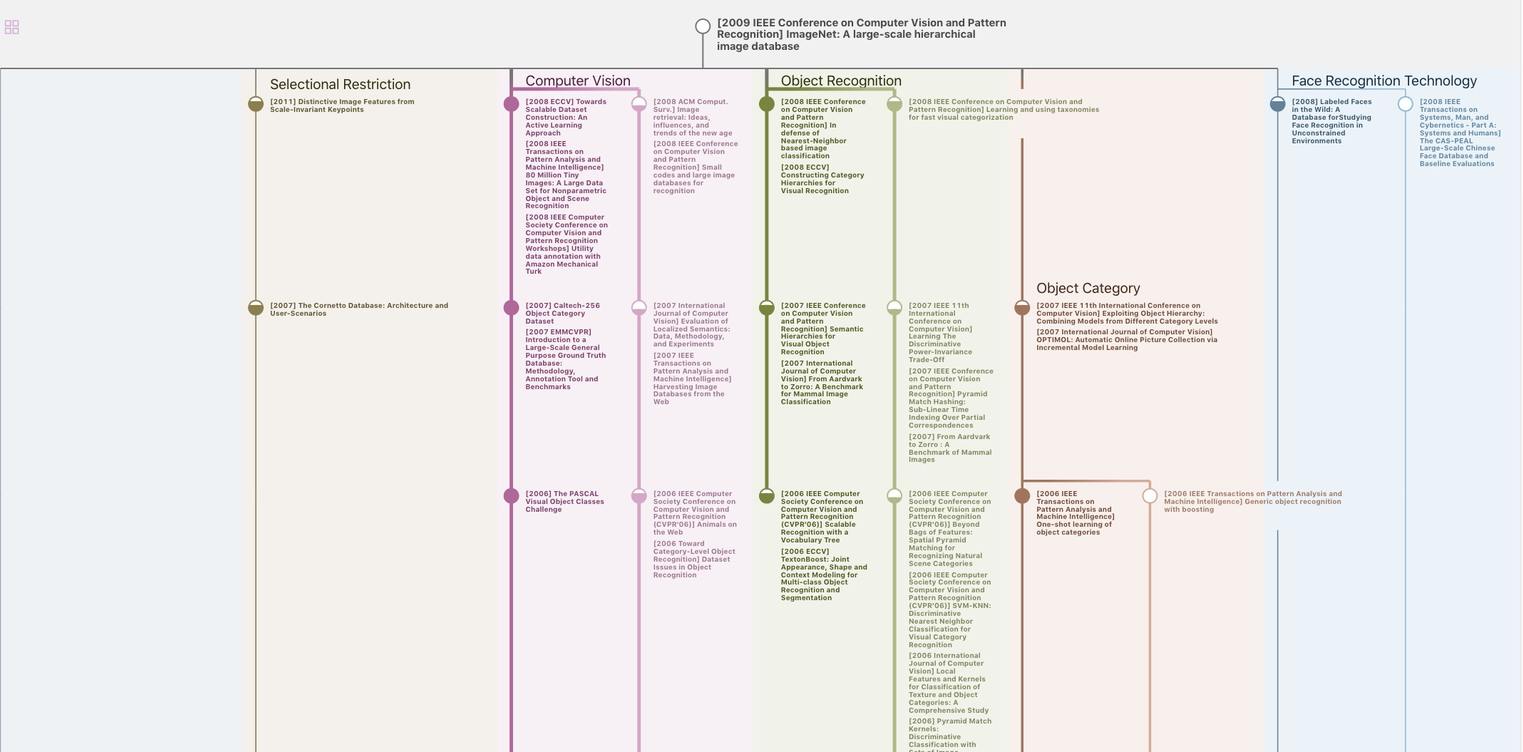
生成溯源树,研究论文发展脉络
Chat Paper
正在生成论文摘要