HW-ADAM: FPGA-Based Accelerator for Adaptive Moment Estimation
ELECTRONICS(2023)
摘要
The selection of the optimizer is critical for convergence in the field of on-chip training. As one second moment optimizer, adaptive moment estimation (ADAM) shows a significant advantage compared with non-moment optimizers such as stochastic gradient descent (SGD) and first-moment optimizers such as Momentum. However, ADAM is hard to implement on hardware due to the computationally intensive operations, including square, root extraction, and division. This work proposed Hardware-ADAM (HW-ADAM), an efficient fixed-point accelerator for ADAM highlighting hardware-oriented mathematical optimizations. HW-ADAM has two designs: Efficient-ADAM (E-ADAM) unit reduced the hardware resource consumption by around 90% compared with the related work. E-ADAM achieved a throughput of 2.89 MUOP/s (Million Updating Operation per Second), which is 2.8x of the original ADAM. Fast-ADAM (F-ADAM) unit reduced 91.5% flip-flops, 65.7% look-up tables, and 50% DSPs compared with the related work. The F-ADAM unit achieved a throughput of 16.7 MUOP/s, which is 16.4x of the original ADAM.
更多查看译文
关键词
adaptive moment estimation,FPGA,on-chip training,accelerator
AI 理解论文
溯源树
样例
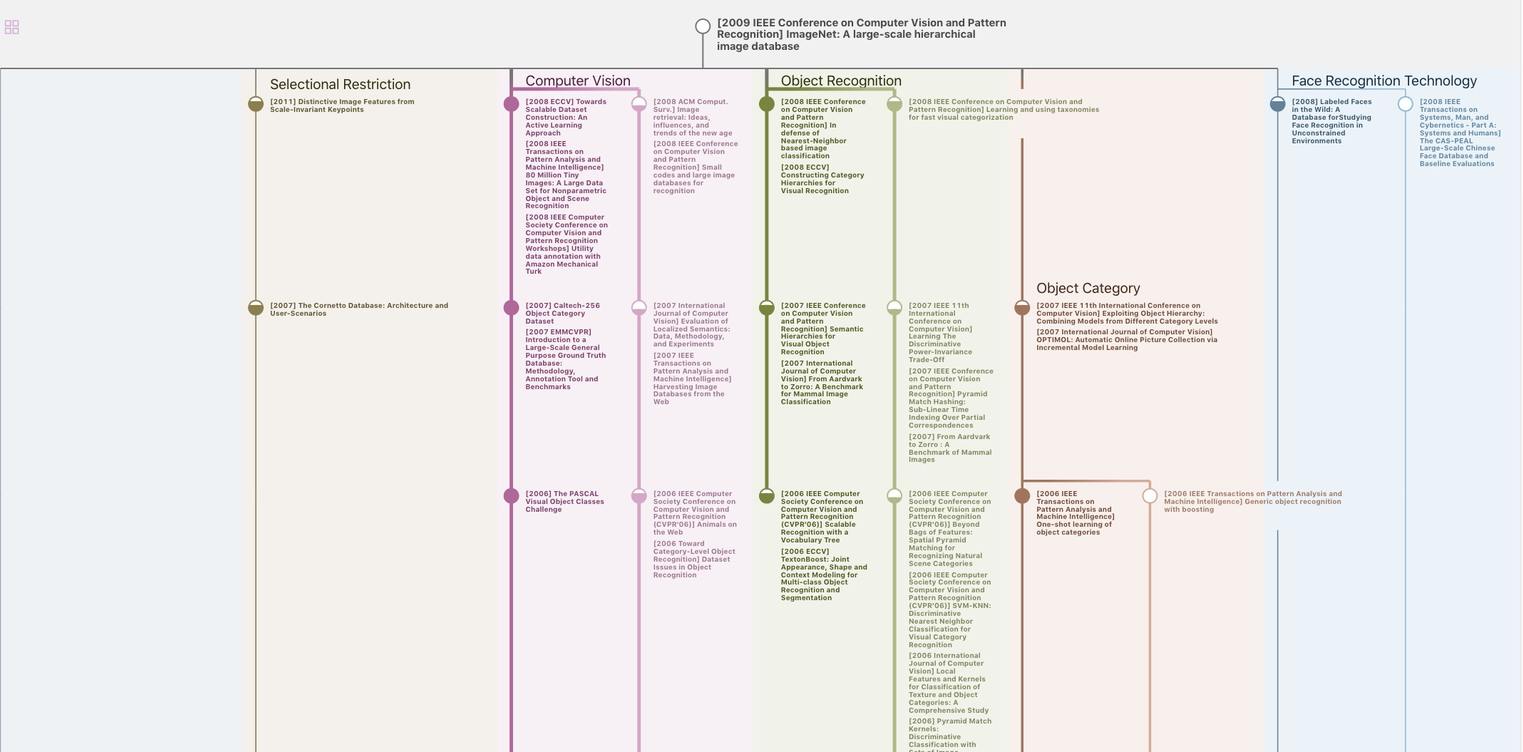
生成溯源树,研究论文发展脉络
Chat Paper
正在生成论文摘要