GAGCN: Generative adversarial graph convolutional network for non-homogeneous texture extension synthesis
IET IMAGE PROCESSING(2023)
摘要
In the non-homogeneous texture synthesis task, the overall visual characteristics should be consistent when extending the local patterns of the exemplar. The existing methods mainly focus on the local visual features of patterns but ignore the relative position features that are important for non-homogeneous texture synthesis. Although these methods have achieved success on homogeneous textures, they cannot perform well on non-homogeneous textures. Thus, it is desirable to model the dependence between pixels to improve the synthesis performance. To ensure synthesis results from both the local detail structure and the overall structure, this paper proposes a non-homogeneous texture extended synthesis model (GAGCN) combining the generate adversarial network (GAN) and the graph convolutional network (GCN). The GAN learns the internal distribution of image patches, which makes the synthetic image have rich local details. The GCN learns the latent dependence between pixels according to the statistical characteristics of the image. Based on this, a novel graph similarity loss is proposed. This loss describes the latent spatial differences between the sample image and the generated image, which helps the model to better capture global features. Experiments show that our method outperforms existing methods on non-homogeneous textures.
更多查看译文
关键词
attention mechanism,generative adversarial networks,graph convolutional networks,non-homogeneous texture synthesis
AI 理解论文
溯源树
样例
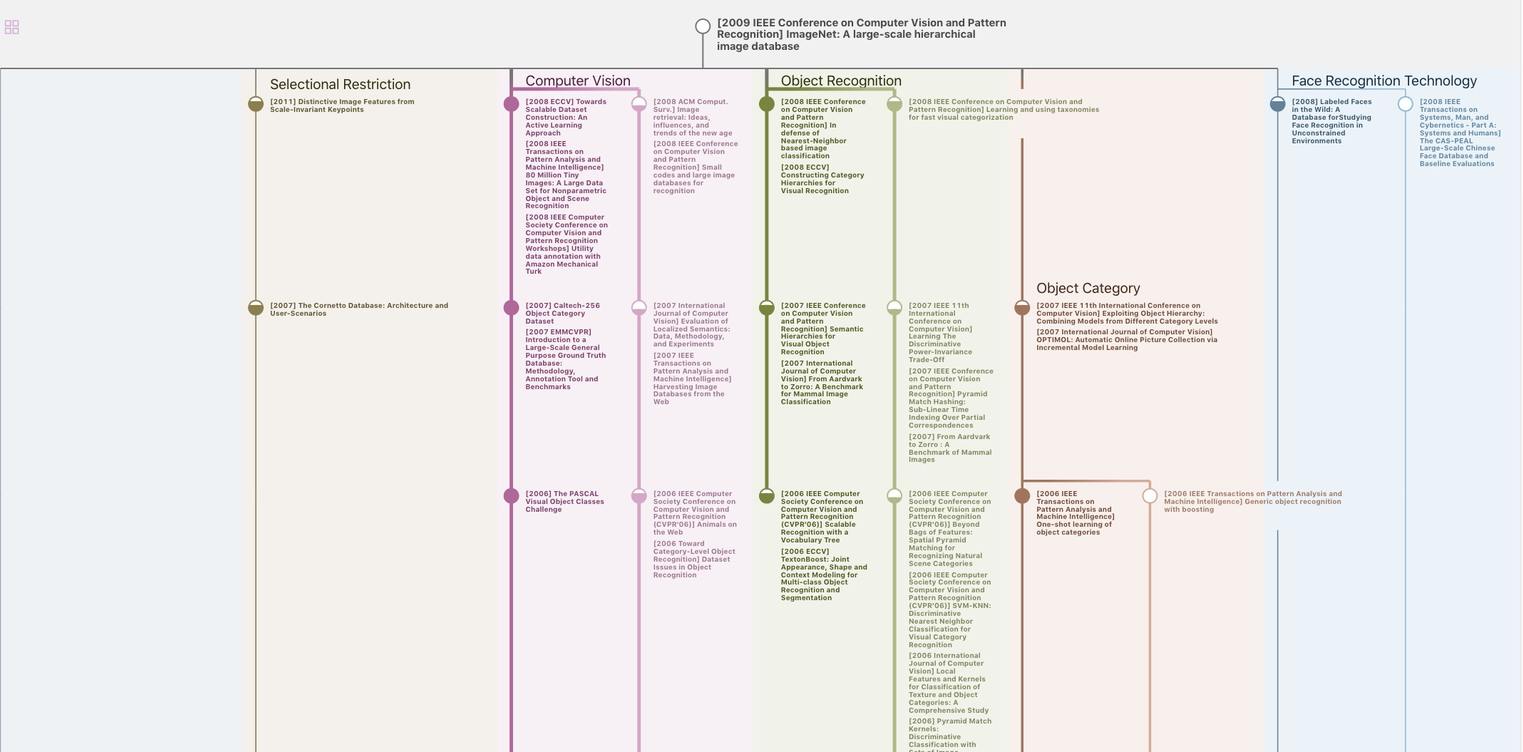
生成溯源树,研究论文发展脉络
Chat Paper
正在生成论文摘要