Distributions of fatigue damage from data-driven strain prediction using Gaussian process regression
STRUCTURAL HEALTH MONITORING-AN INTERNATIONAL JOURNAL(2023)
摘要
Fatigue is a leading cause of structural failure; however, monitoring and prediction of damage accumulation remains an open problem, particularly in complex environments where maintaining sensing equipment is challenging. As a result, there is a growing interest in virtual loads monitoring, or inferential sensing, particularly for predicting strain in areas of interest using machine learning methods. This paper pursues a probabilistic approach, relying on a Gaussian process (GP) regression, to produce both strain predictions and a predictive distribution of the accumulated fatigue damage in a given time period. Here, the fatigue distribution is achieved via propagation of successive draws from the posterior GP through a rainflow count. The establishment of such a distribution crucially accounts for uncertainty in the predictive model and will form a valuable element in any probabilistic risk assessment. For demonstration of the method, distributions for predicted fatigue damage in an aircraft wing are produced across 84 flights. The distributions provide a robust measure of predicted damage accumulation and model uncertainty.
更多查看译文
关键词
Gaussian process,probabilistic,fatigue assessment,posterior sampling,uncertainty propagation,data-driven,strain prediction
AI 理解论文
溯源树
样例
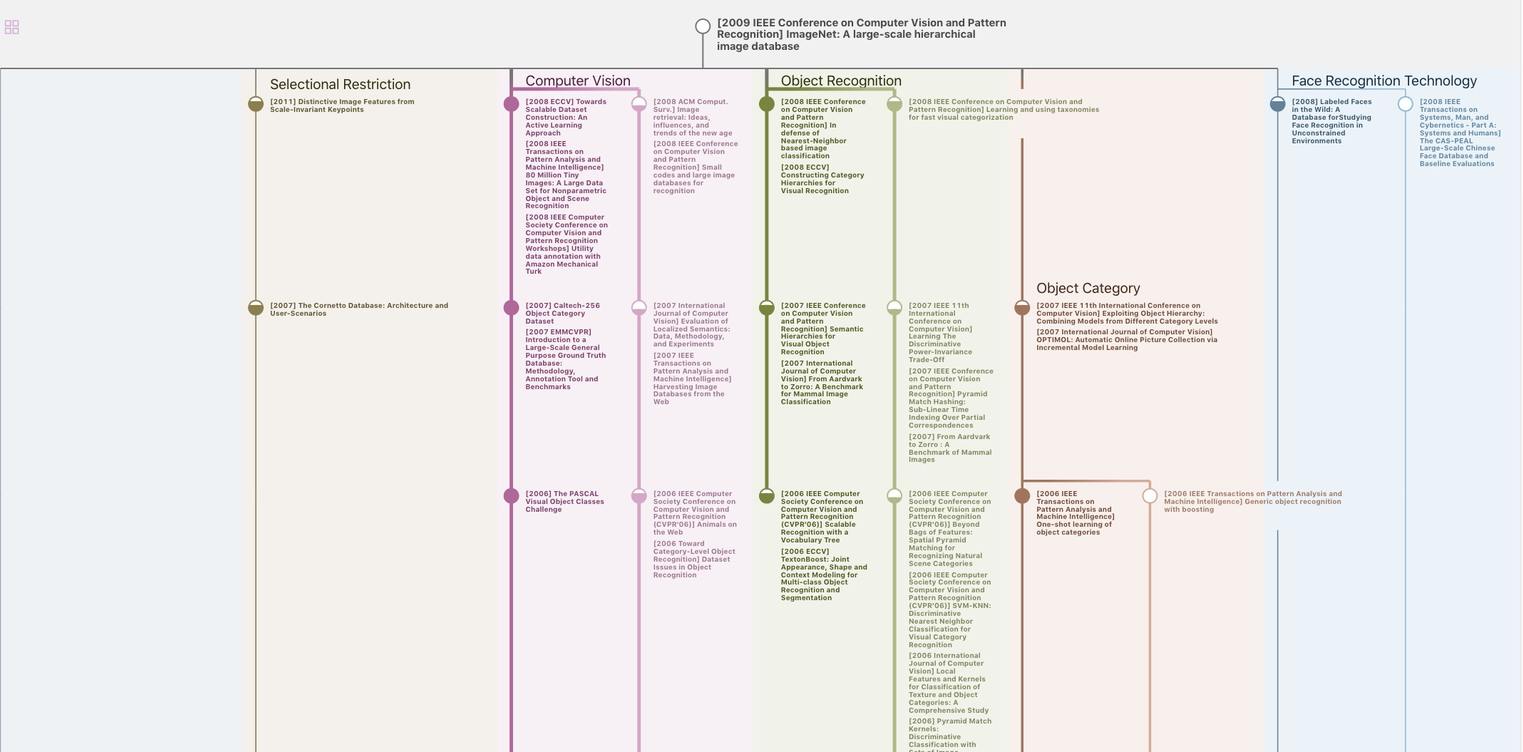
生成溯源树,研究论文发展脉络
Chat Paper
正在生成论文摘要