On the Feasibility of Real-Time HRV Estimation Using Overly Noisy PPG Signals
COMPUTERS(2022)
摘要
Heart Rate Variability (HRV) is a biomarker that can be obtained non-invasively from the electrocardiogram (ECG) or the photoplethysmogram (PPG) fiducial points. However, the accuracy of HRV can be compromised by the presence of artifacts. In the herein presented work, a Simulink((R)) model with a deep learning component was studied for overly noisy PPG signals. A subset with these noisy signals was selected for this study, with the purpose of testing a real-time machine learning based HRV estimation system in substandard artifact-ridden signals. Home-based and wearable HRV systems are prone to dealing with higher contaminated signals, given the less controlled environment where the acquisitions take place, namely daily activity movements. This was the motivation behind this work. The results for overly noisy signals show that the real-time PPG-based HRV estimation system produced RMSE and Pearson correlation coefficient mean and standard deviation of 0.178 +/- 0.138 s and 0.401 +/- 0.255, respectively. This RMSE value is roughly one order of magnitude above the closest comparative results for which the real-time system was also used.
更多查看译文
关键词
photoplethysmogram,heart rate variability,real-time,Simulink((R)),deep learning
AI 理解论文
溯源树
样例
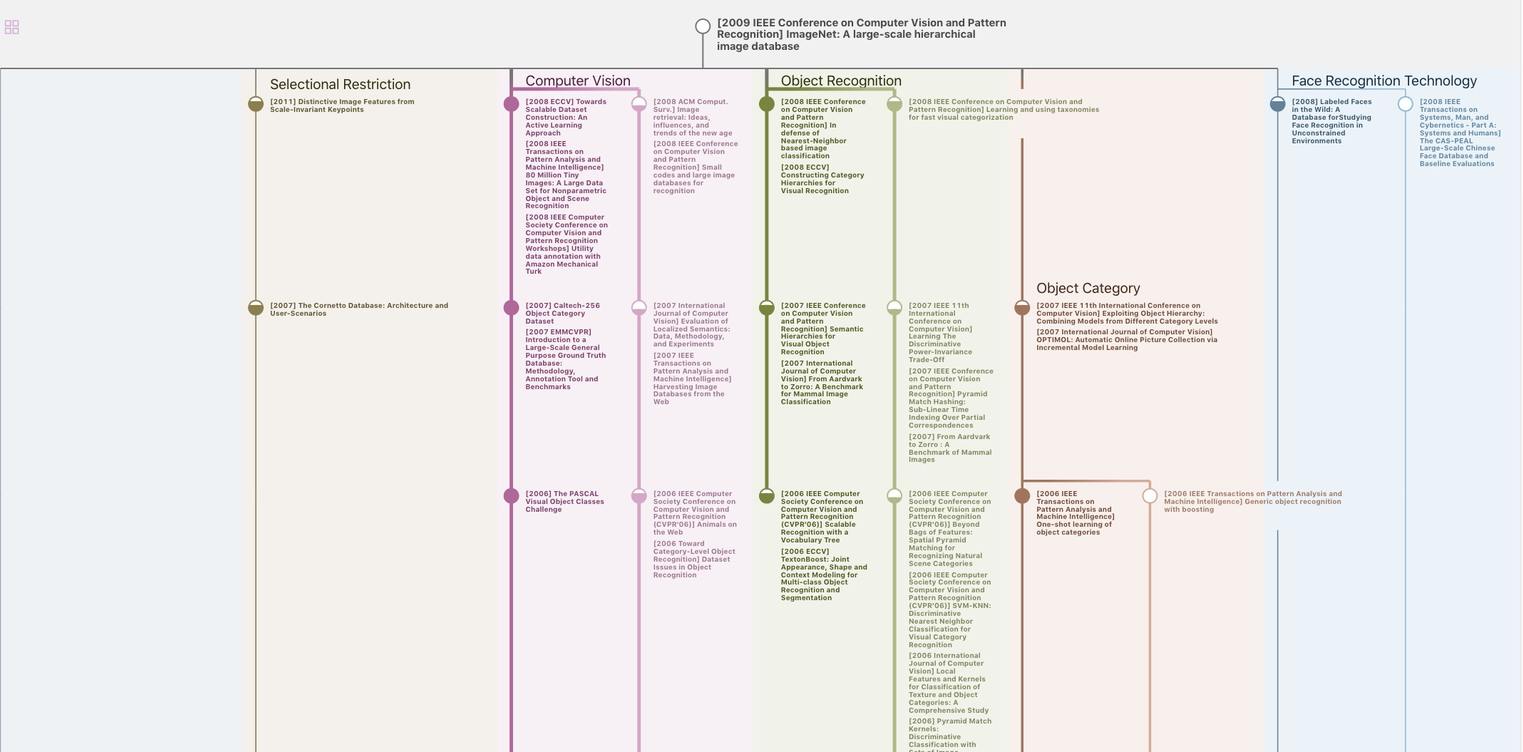
生成溯源树,研究论文发展脉络
Chat Paper
正在生成论文摘要