Soil sensing and machine learning reveal factors affecting maize yield in the mid-Atlantic United States
AGRONOMY JOURNAL(2023)
摘要
In large-scale arable cropping systems, understanding within-field yield variations and yield-limiting factors are crucial for optimizing resource investments and financial returns, while avoiding adverse environmental effects. Sensing technologies can collect various crop and soil information, but there is a need to assess whether they reveal within-field yield constraints. Spatial data regarding grain yields, proximal soil sensing data, and topographical and soil properties were collected from 26 maize (Zea mays L.) growing fields in the U.S. Mid-Atlantic. Apparent soil electrical conductivity (ECa) collected by an on-the-go sensor (Veris) was an effective method for estimating subsoil textural variation and water holding capacity in the Coastal Plain region, which was also the best predictor of spatial yield pattern when combined with surface pH and topographic wetness index in a Random Forest (RF) model. In the Piedmont Plateau region, proximal soil sensors showed a lower correlation to measured soil properties, while topographical properties (aspect and slope) were important estimators of spatial yield patterns in an RF model. In locations where the RF model failed to predict yield variation, soil compaction appeared to be limiting crop yields. In conclusion, the application of RF models using ECa sensors and topographical properties was effective in revealing within-field yield constraints, especially in the Coastal Plain region. On the Piedmont Plateau, the calibration of proximal sensor information needs to be improved with a particular focus on soil compaction.
更多查看译文
AI 理解论文
溯源树
样例
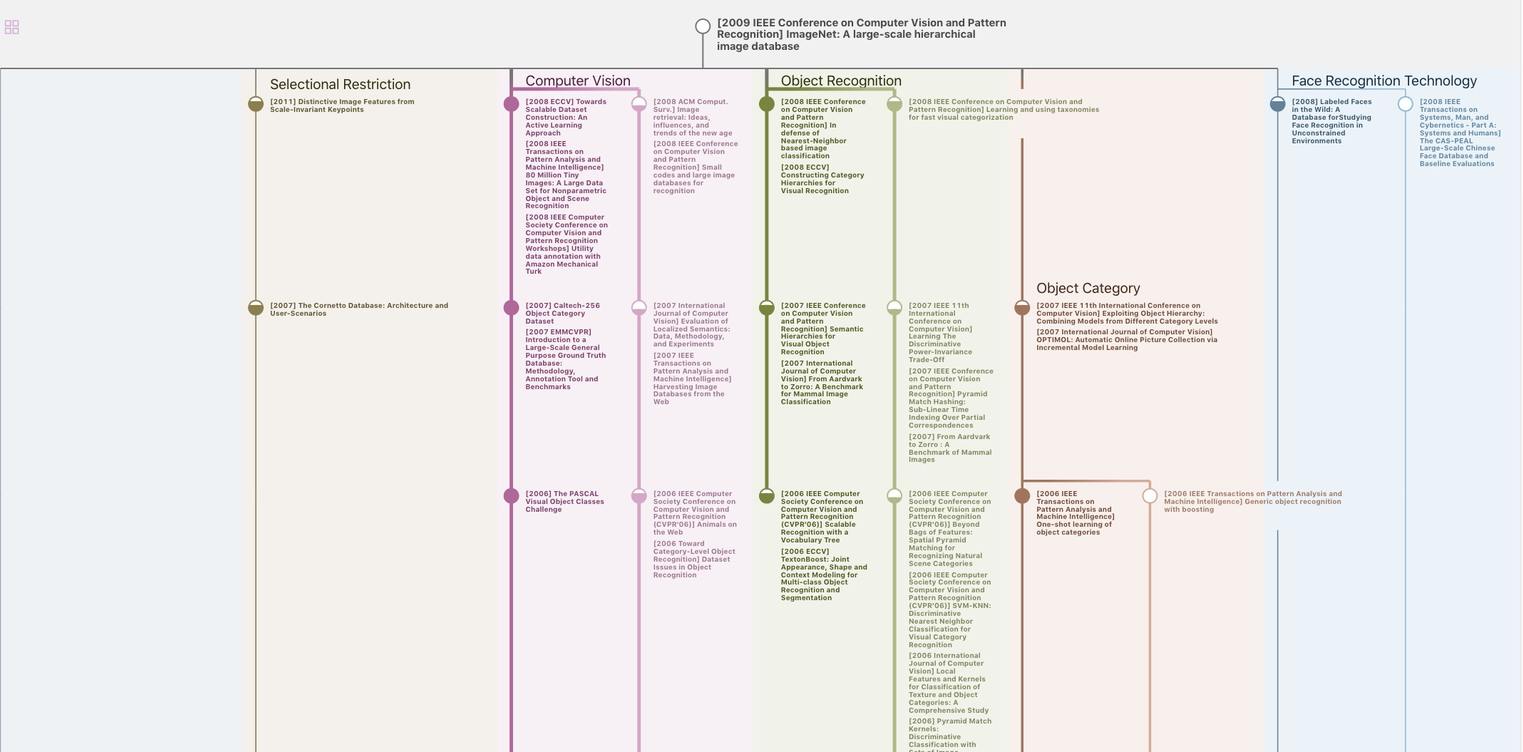
生成溯源树,研究论文发展脉络
Chat Paper
正在生成论文摘要