Modified Residual Dense Network based super-resolution localization method for high-concentration microbubbles
2022 IEEE INTERNATIONAL ULTRASONICS SYMPOSIUM (IEEE IUS)(2022)
摘要
The practical limitation of ultrasound localization microscopy for clinical translation is the trade-off between microbubble concentration and data acquisition time. Recently, deep learning-based approaches have shown promising capability in microbubble localization accuracy when using a high-concentration microbubble injection to shorten acquisition time. In this study, we construct a Modified Residual Dense Network (MRDN) for high-concentration microbubble super-resolution localization. By subjecting the collected data to non-local mean filtering operations, the MRDN is used for continuous learning. This method can be well used at high concentrations of 16 mm(-2) with a high localization accuracy (localization error:22.3 mu m) and high localization reliability (Jaccard index:0.78).
更多查看译文
关键词
Deep Learning,microbubble localization,high-concentration
AI 理解论文
溯源树
样例
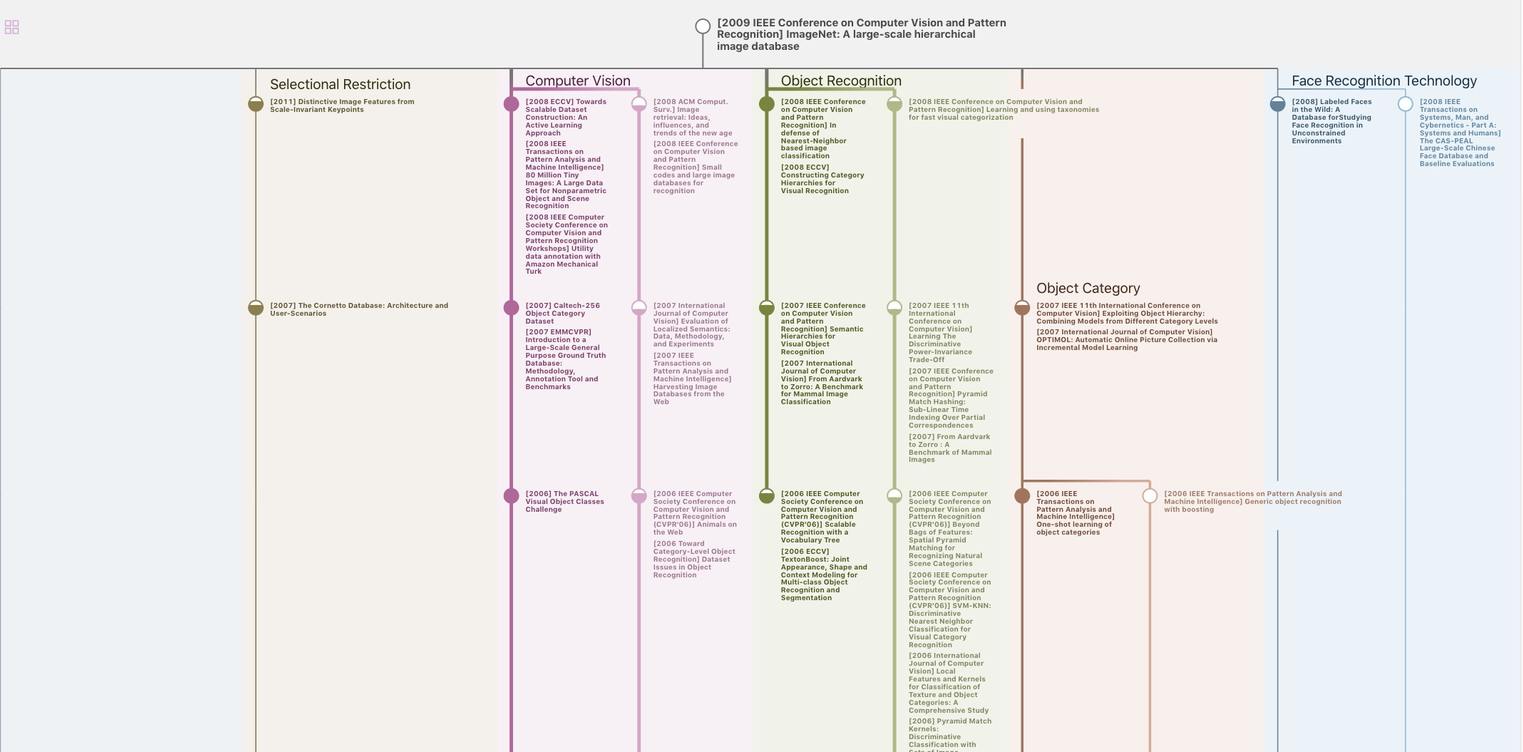
生成溯源树,研究论文发展脉络
Chat Paper
正在生成论文摘要