EFND: A Semantic, Visual, and Socially Augmented Deep Framework for Extreme Fake News Detection
SUSTAINABILITY(2023)
摘要
Due to the exponential increase in internet and social media users, fake news travels rapidly, and no one is immune to its adverse effects. Various machine learning approaches have evaluated text and images to categorize false news over time, but they lack a comprehensive representation of relevant features. This paper presents an automated method for detecting fake news to counteract the spread of disinformation. The proposed multimodal EFND integrates contextual, social context, and visual data from news articles and social media to build a multimodal feature vector with a high level of information density. Using a multimodal factorized bilinear pooling, the gathered features are fused to improve their correlation and offer a more accurate shared representation. Finally, a Multilayer Perceptron is implemented over the shared representation for the classification of fake news. EFND is evaluated using a group of standard fake news datasets known as "FakeNewsNet". EFND has outperformed the baseline and state-of-the-art machine learning and deep learning models. Furthermore, the results of ablation studies have demonstrated the efficacy of the proposed framework. For the PolitiFact and GossipCop datasets, the EFND has achieved an accuracy of 0.988% and 0.990%, respectively.
更多查看译文
关键词
multimodal fake news detection,Multimodal Factorized Bilinear pooling,natural language processing,social sensing,misinformation,disinformation
AI 理解论文
溯源树
样例
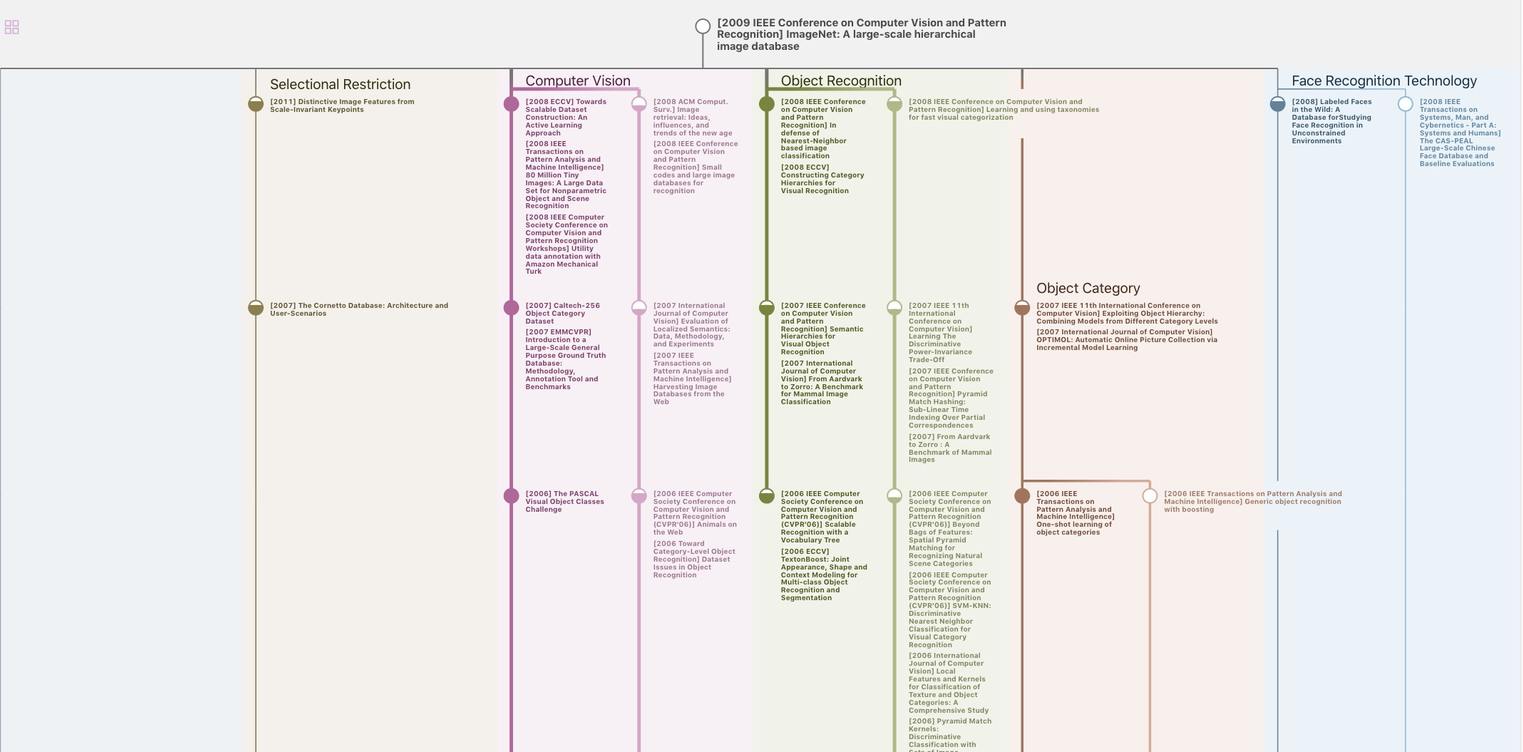
生成溯源树,研究论文发展脉络
Chat Paper
正在生成论文摘要