On the Importance of Direct-Levelling for Constitutive Material Model Calibration using Digital Image Correlation and Finite Element Model Updating
EXPERIMENTAL MECHANICS(2022)
摘要
Background Finite element model updating (FEMU) is an inverse technique that is used to identify material (constitutive) model parameters based on experimental data. These experimental data, often in the form of full-field strains, may be subject to a filtering bias unique to the measurement technique, which can propagate to material parameter identification error. Objective Numerically adjusting for this filtering mismatch between the finite element analysis (FEA) and experimental measurements, here from Digital Image Correlation (DIC), is necessary to produce an accurate calibration. We investigate “direct-leveling” the FEA to the DIC data, i.e. computing strains using consistent methods and length scales for both data sets, before performing model calibration. Thus, both data sets have the same spatial resolution and can be quantitatively compared more readily. Methods We generated two sets of synthetic “experimental” DIC displacement data: one directly from FEA nodal displacements and one from DIC images synthetically deformed according to the FEA displacements. We then explored how the FEMU material model parameter identification is affected by DIC user-defined settings, including virtual strain gauge size, step size, and subset shape function, as well as misalignment between the FEA and DIC datasets. Results We found that direct-levelling of the FEA data before FEMU calibration returned more accurate results. This accuracy was independent of the DIC settings and spatial resolution. In contrast, performing FEMU with the unlevelled FEA data resulted in significant biases in the identified parameters. Conclusion In FEMU-based calibrations, it is advantageous to properly level the strain from the FEA to match the filtering and spatial resolution of the DIC results.
更多查看译文
关键词
Finite Element Model Updating,Digital Image Correlation,Virtual Strain Gage,DIC Levelling,Direct-Levelling
AI 理解论文
溯源树
样例
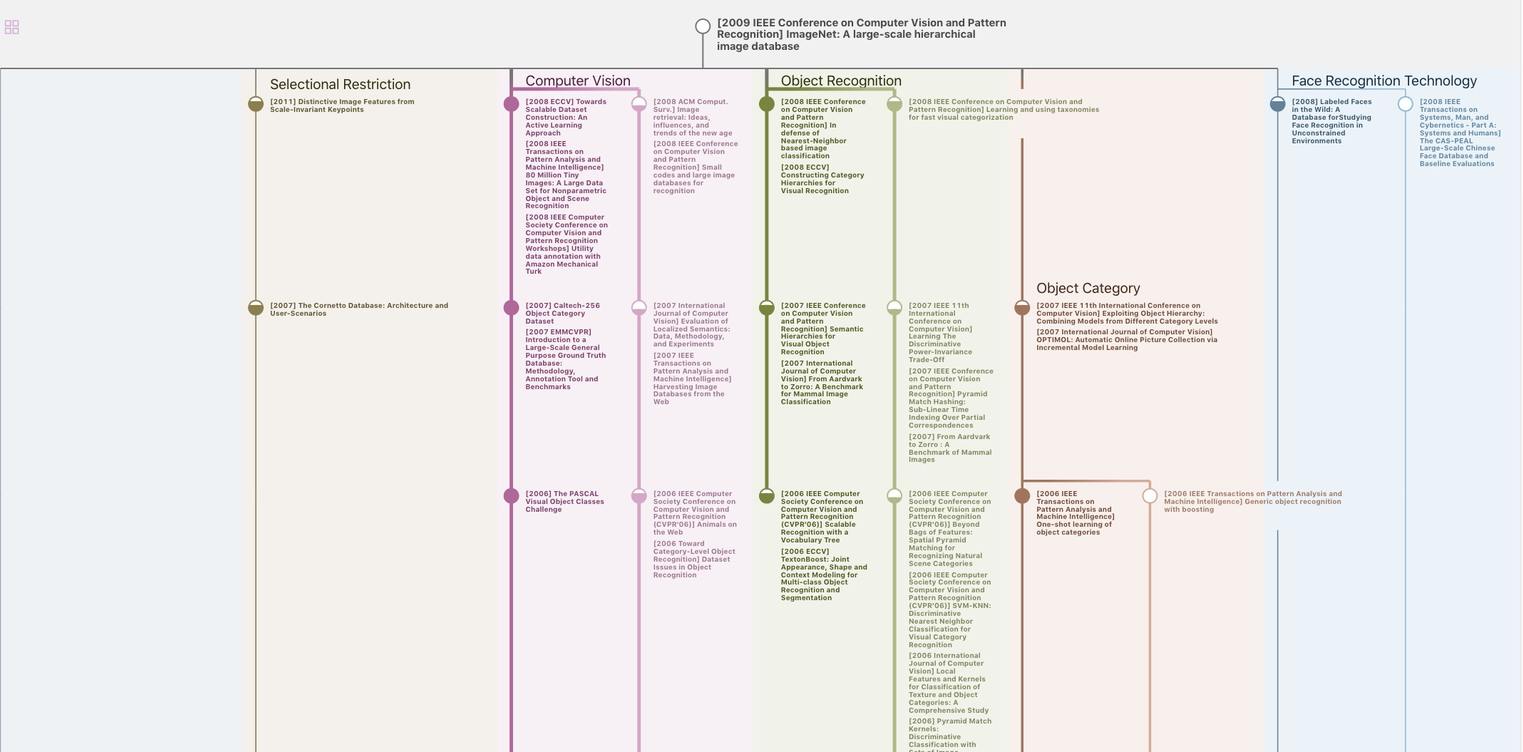
生成溯源树,研究论文发展脉络
Chat Paper
正在生成论文摘要