Adaptive Style Modulation for Artistic Style Transfer
NEURAL PROCESSING LETTERS(2022)
摘要
Arbitrary-style-per-model (ASPM) style transfer algorithms transfer arbitrary styles based on a single model. Statistics-based learning algorithms of ASPM, represented by adaptive instance normalization (AdaIN), conduct instance normalization and then perform an affine transformation on target features. These algorithms are computationally efficient and easy to embed in convolutional neural networks. Consequently, they are widely used in image synthesis tasks to control the style of the resulting images. However, the style of stylized images may be a combination of content and stylized images, which suggests that these methods do not transform styles accurately. In this work, we rethink the function of AdaIN in controlling style. We show that the role of AdaIN is to (1) give each input content image a specific optimization target, (2) dynamically set cross-channel correlations, and (3) act as a feature selector after combining it with an activation function. Accordingly, we propose adaptive style modulation (AdaSM), which fully leverages the three roles mentioned above and thereby enables more precise control of global style. Experimental results show that AdaSM provides superior style controllability, alleviates the style blending problem, and outperforms state-of-the-art methods in artistic style transfer tasks.
更多查看译文
关键词
Style transfer,Neural networks,Image processing,Deep learning
AI 理解论文
溯源树
样例
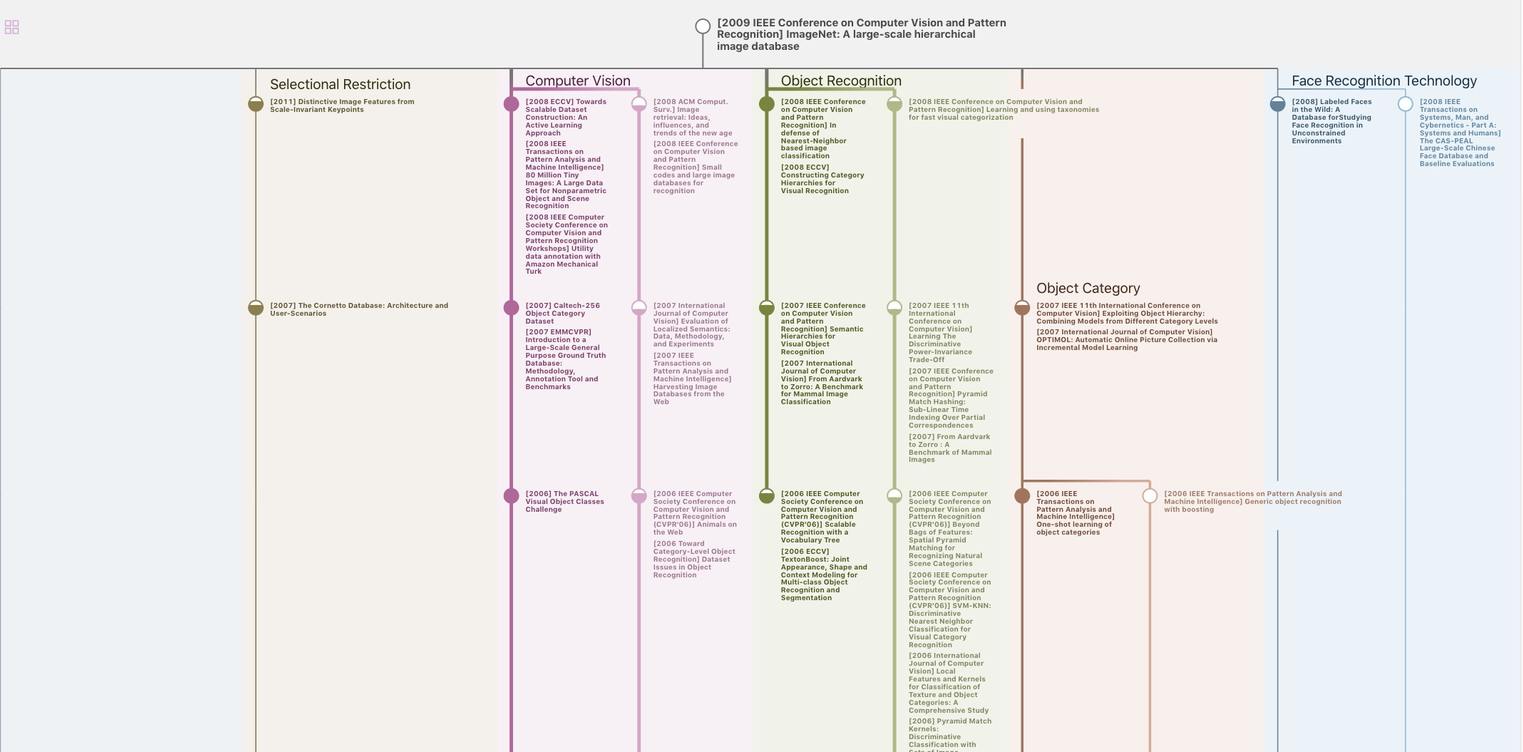
生成溯源树,研究论文发展脉络
Chat Paper
正在生成论文摘要