An improved GraphSAGE to detect power system anomaly based on time-neighbor feature
ENERGY REPORTS(2023)
摘要
With the development of smart grids, more and more information appears in power systems. Therefore, information security has attracted more attention, since malicious attacks aimed at power systems are increasing and causing heavy losses. However, the current research on data identification of power systems has not considered hidden false data. Therefore, a time-neighborbased GraphSAGE anomaly detection model is constructed by this paper, which can detect the features of the target node type from the neighbor features and its own time series features. More importantly, it can effectively detect hidden anomalies in the data, and provide more secure data for power system operation. First, in order to detect the authenticity of the data, the similarities between the features of the target node and the neighbor nodes are calculated, and the similarity of the features of the target node and the neighbor nodes varies with time. Second, the GraphSAGE introduced the neighbor information of the target node, and used the similarity to supervise the training of the GraphSAGE. Finally, the model this paper constructed is verified on the public datasets and compared with the traditional anomaly detection method. The results show that the timeneighbor-based GraphSAGE anomaly detection can effectively detect abnormal data, the Recall and Auc indicators increased by 0.2 and 0.3 respectively using this anomaly detection method. (c) 2022 The Author(s). Published by Elsevier Ltd. This is an open access article under theCCBY-NC-ND license (http://creativecommons.org/licenses/by-nc-nd/4.0/).
更多查看译文
关键词
Anomaly detection,GraphSAGE,FDIA,Power system,Time-neighbor
AI 理解论文
溯源树
样例
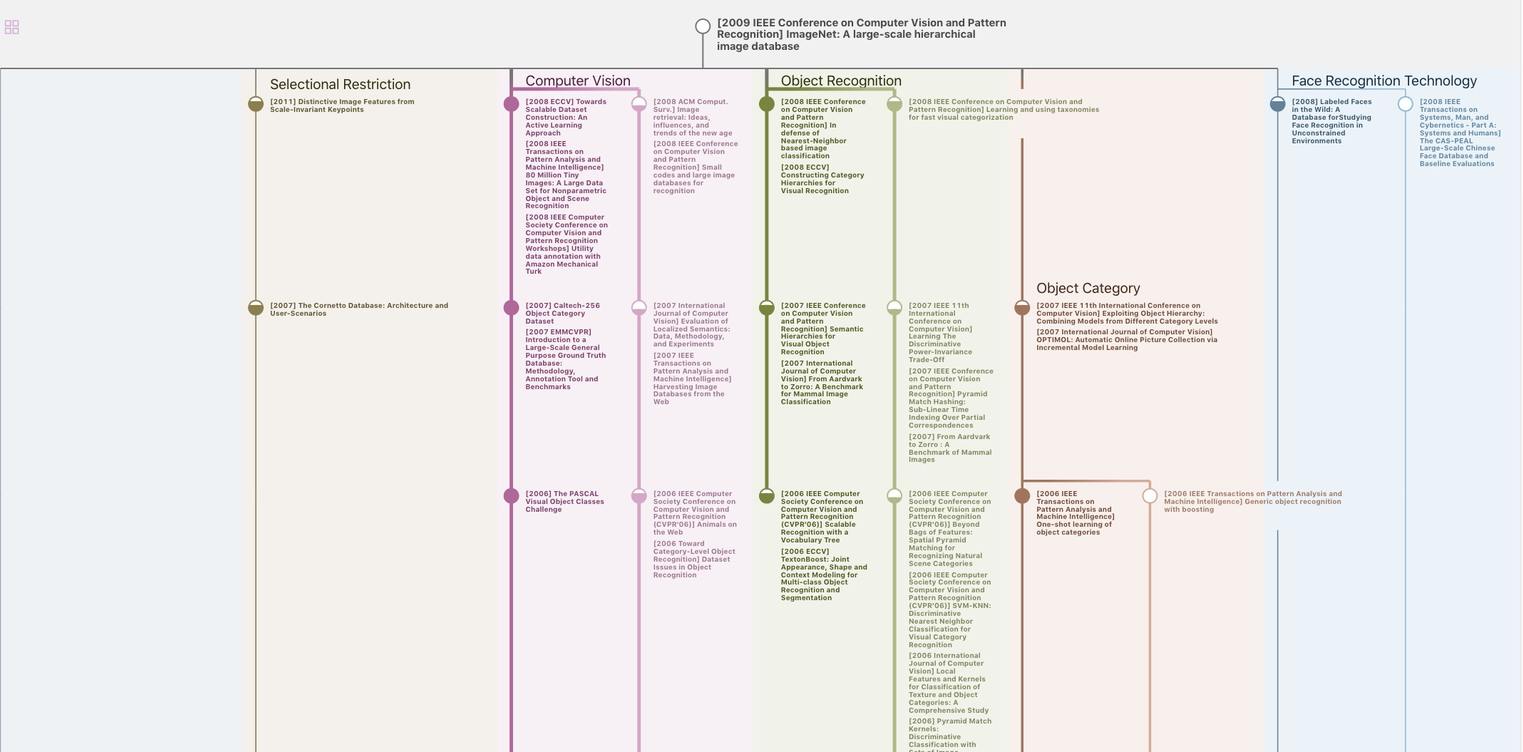
生成溯源树,研究论文发展脉络
Chat Paper
正在生成论文摘要