A progressively-enhanced framework to broad networks for efficient recognition applications
MULTIMEDIA TOOLS AND APPLICATIONS(2022)
摘要
Broad neural networks provide an alternative way of deep learning with a novel flatted structure, which employ a non-iterative training mechanism and exhibit high efficiency in various recognition tasks. However, such broad networks unavoidably suffer from unstable performance due to the double random mappings in the generation of feature representations and the consequent uncertainty. The existing research often neglect to explore the effect of the quality of the broaden feature representations, which is crucial for the model performance. This paper presents a progressively-enhanced framework taking broad network as basic learners (PE-BL) to address the existing issues. The basic broad learners in PE-BL are trained in sequence, and the core manipulation is to modify the primitive hidden feature representations of the current learner through the nonlinear transformation of the prediction from the previous one, so the resulting broaden representations become more discriminative. Further, PE-BL is adapted to the scenarios where only a single broad learner is employed. Finally, extensive comparative experiments on some benchmark datasets and Electroencephalogram (EEG)-based emotion recognition task verify the effectiveness of the proposed methods.
更多查看译文
关键词
Pattern recognition,Broad networks,Broad pseudoinverse learner,Progressively-enhanced learning
AI 理解论文
溯源树
样例
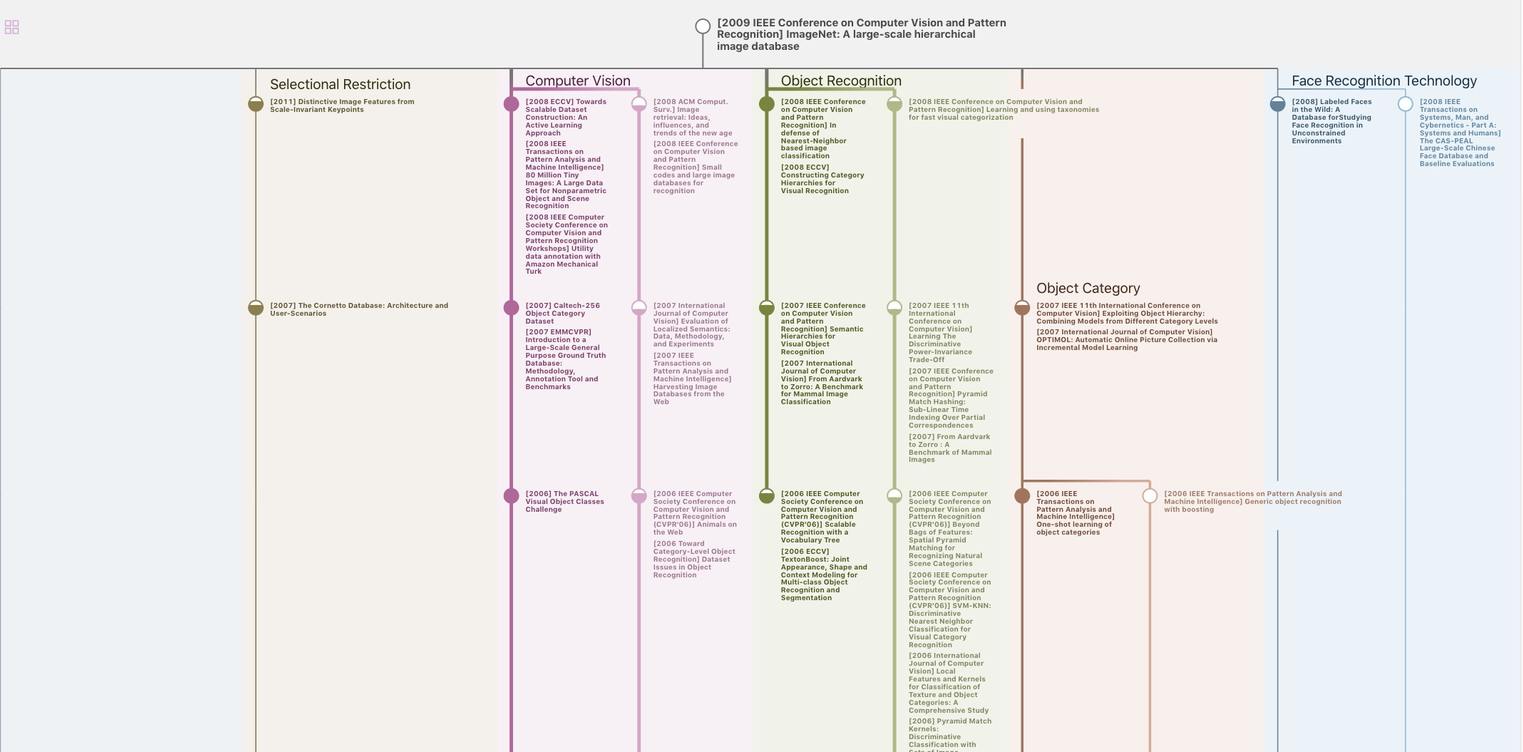
生成溯源树,研究论文发展脉络
Chat Paper
正在生成论文摘要