Abnormal Data Recovery of Structural Health Monitoring for Ancient City Wall Using Deep Learning Neural Network
INTERNATIONAL JOURNAL OF ARCHITECTURAL HERITAGE(2024)
摘要
Continuous structural health monitoring is of great importance to preventive conservation for ancient architectural heritages. However, abnormal monitoring data may trigger false alarming of structural damages. SHM of ancient buildings also needs abnormal data recovering. Most of the existing studies used the neural network with single input dimension and forward prediction to recover abnormal data, which is difficult to accurately predict long data sequences. This study developed a novel abnormal data recovery framework. The main novelty of the proposed framework is that the input and output configurations of the GRU model are optimized. Meanwhile, to make full use of the forward and backward information of the abnormal data sequence, bidirectional prediction is used to improve the prediction accuracy. The framework is implemented in the abnormal monitoring data recovering for an ancient city wall built 600 years ago in Beijing. Three types of abnormal data, including outlier, drift, and missing, are considered in this study. The results reveal that the proposed framework has high accuracy in abnormal data recovering of strain and crack width. The recovered data has the same regular diurnal variation as the normal monitoring data. The linear correlation between the structural responses and wall temperature gets obviously improved after data recovering. The proposed framework shows great capacity of abnormal data recovery for structural static responses of ancient buildings, which are usually influenced by environmental temperature variation.
更多查看译文
关键词
Abnormal data recovery,ancient city wall,deep learning,neural network,structural health monitoring (SHM)
AI 理解论文
溯源树
样例
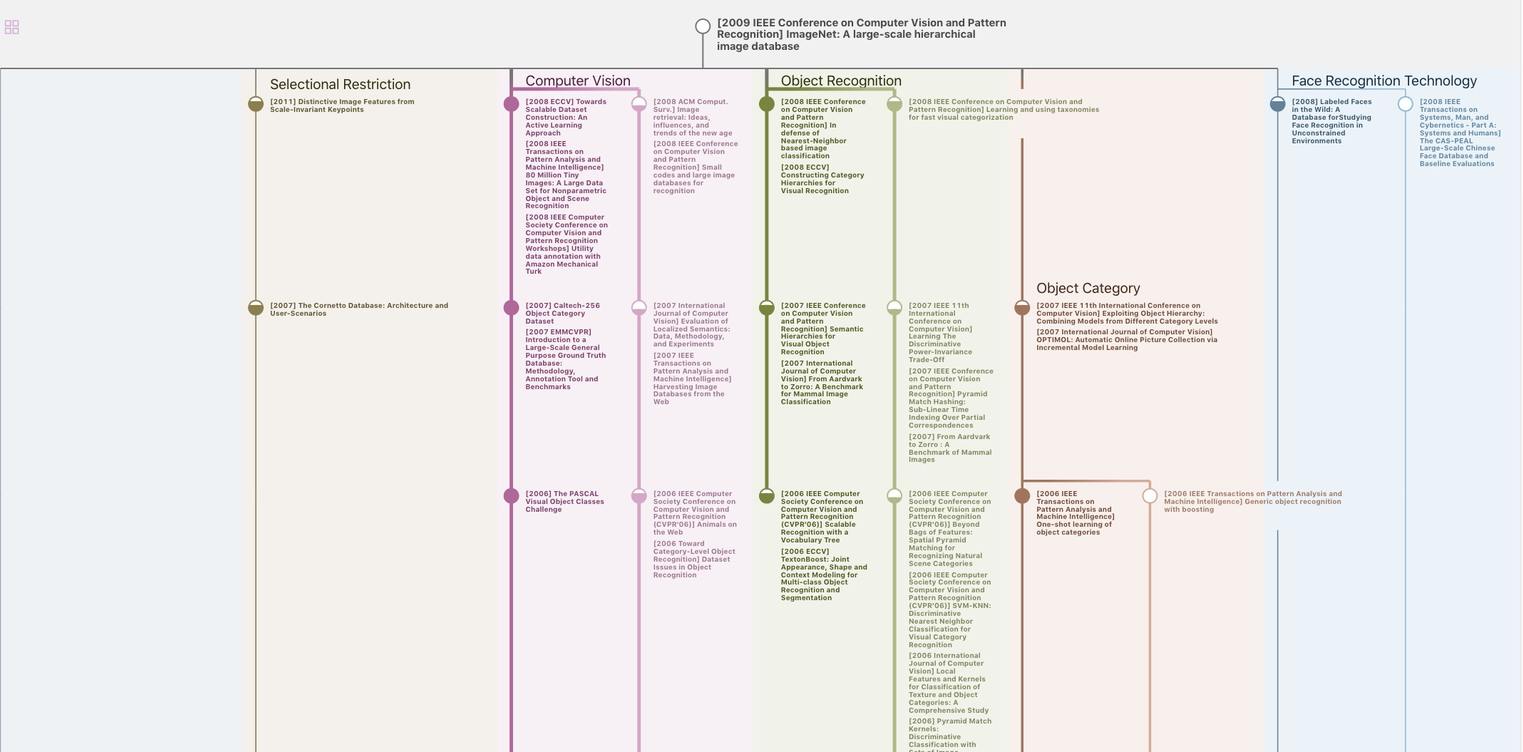
生成溯源树,研究论文发展脉络
Chat Paper
正在生成论文摘要